Enhanced Aggregation-Induced Emission Activity of Metal-Organic Frameworks by Using Machine Learning Technology
ACS SUSTAINABLE CHEMISTRY & ENGINEERING(2022)
摘要
Metal-organic frameworks (MOFs) with aggregation-induced emission (AIE) activity show a high emission intensity, high sensitivity, and high resolution in biological imaging and identification technologies. However, their AIE activity is controlled by various Eu precursors' components and synthesis process parameters, and traditional research methods are hard to deal with these complex multiple parameter systems. In this work, we utilize two machine learning technologies to optimize the synthesis process parameters of Eu-MOFs and analyze their synthesis mechanism. First, we choose gradient boosting decision tree (GBDT) regression as the best fitting model. Second, on the basis of the SHapley Additive exPlanation (SHAP) calculation method with the PL/UV intensity ratio regarded as the evaluation standard, we demonstrate that the Eu-precursor concentration (1.91 x 10(7)) and synthesis time (1.73 x 10(7)) dominate in the synthesis systems. Meanwhile, these two parameters show synergic and antagonistic effects on the PL/UV intensity ratio, respectively. Finally, we employ a greedy random walk method to work out that "142-0.83-4.1" should be the best optimization process parameters, and the corresponding sample shows a high photoluminescence quantum yield (PLQY) with a value of 7.65% in the solid state. More importantly, the screen-printed pattern exhibits bright red fluorescence under UV light.
更多查看译文
关键词
metal-organic frameworks, aggregation-induced emission activity, machine learning technology, synthesis mechanism, screen printing
AI 理解论文
溯源树
样例
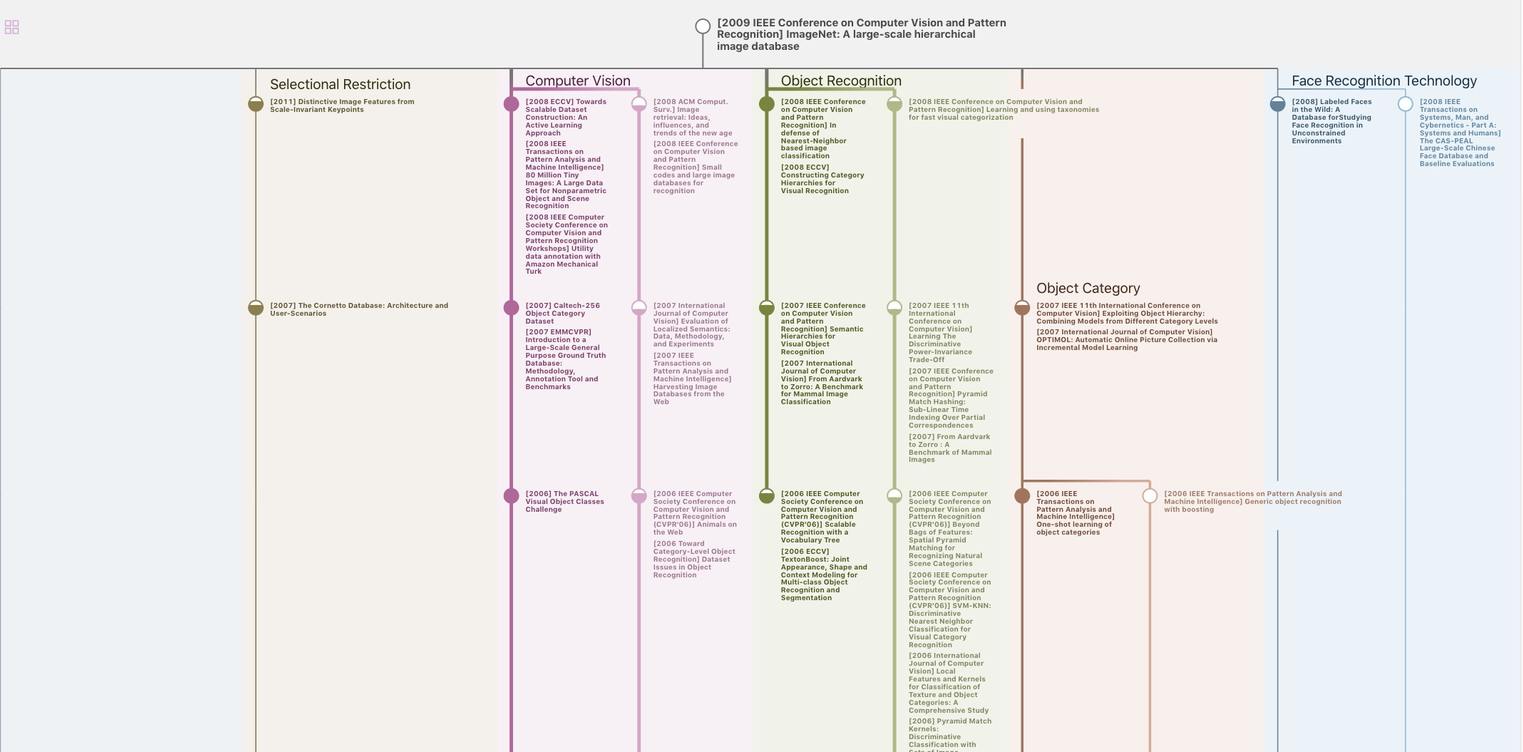
生成溯源树,研究论文发展脉络
Chat Paper
正在生成论文摘要