Performance evaluation of GRNN and ANFIS controlled DVR using machine learning in distribution network
OPTIMAL CONTROL APPLICATIONS & METHODS(2023)
摘要
A machine learning based generalized neural network estimator (GRNNE) and Takagi-Sugeno (T-S) fuzzy control system is implemented to accelerate the functional performance indices of dynamic voltage restorer (DVR). The GRNNE predictive model is recommended for the fast estimation of the reference load voltage under the distorted power supply. The fruit fly optimization learning strategy is employed to optimize the weights and smoothing parameters for the extraction of the reference voltage signals as well as unit vectors, resulting in the required sinusoidal load component. The dynamics in the DC-link voltage are optimized by the metaheuristic-based gray wolf optimization algorithm. The coefficients of the adaptive controller are updated automatically to achieve the best fitted adaptive neuro-fuzzy inference system predictive model with the least tracking voltage deviation error even in the presence of voltage disturbances. In comparison to classical techniques, the recommended neural-based approach offers a faster convergence speed with fewer parameters to tune, shorter training time, and lower risks of local entrapment. The performance metrics such as mean square error, root mean square error, mean absolute error, mean absolute percent error, coefficients of correlational and determination (R and R-2) are used to evaluate the efficacy of the proposed controller and hence enhance the DVR performance. Finally, the simulation results of the hybrid approach confirm that the NN-based DVR estimator has proven its ability to alleviate voltage sensible issues at critical loads and outperform others in reducing power quality issues.
更多查看译文
关键词
DVR, FOA, fuzzy C-means, GRNN, GWO, sag and swell, statistical tools
AI 理解论文
溯源树
样例
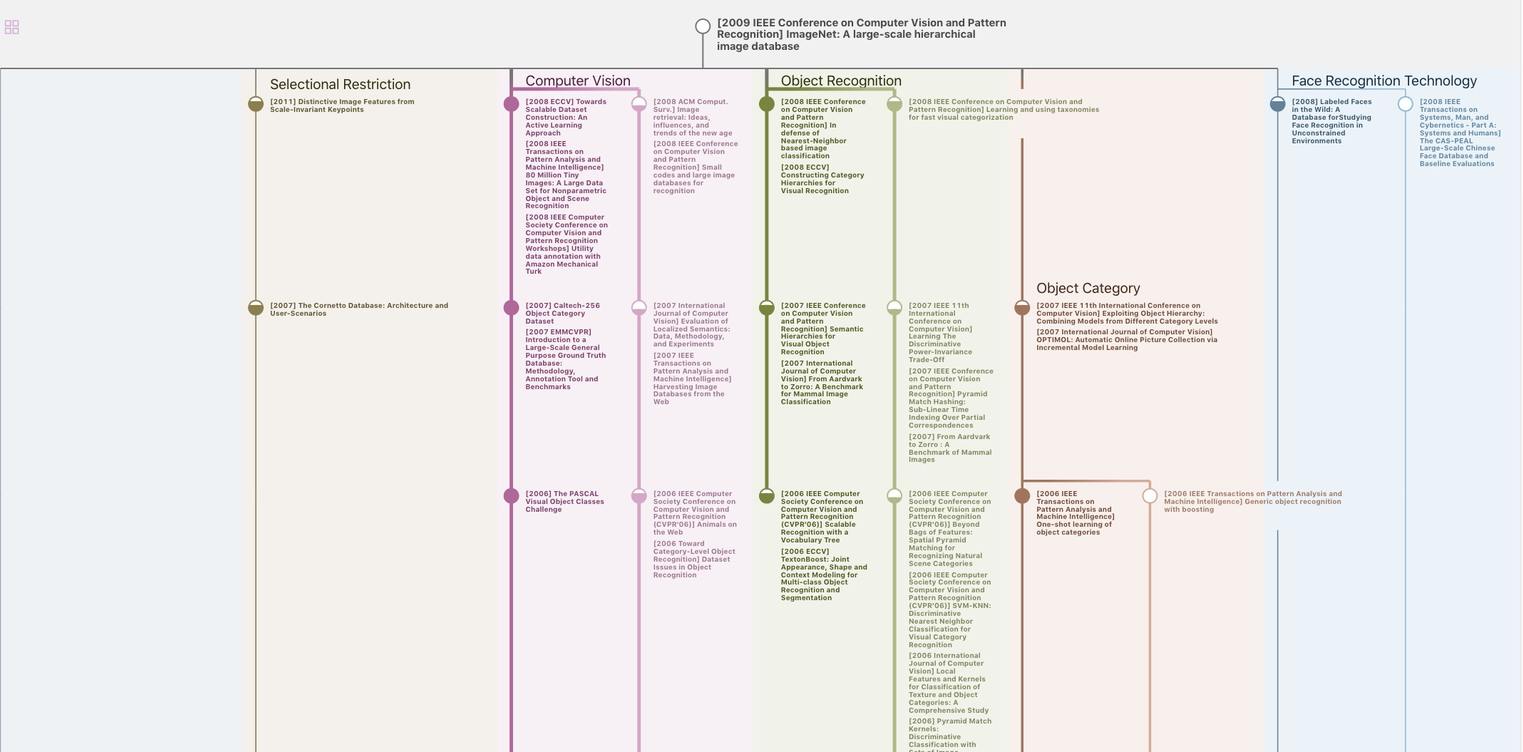
生成溯源树,研究论文发展脉络
Chat Paper
正在生成论文摘要