Fractional-weighted entropy-based fuzzy G-2DLDA algorithm: a new facial feature extraction method
MULTIMEDIA TOOLS AND APPLICATIONS(2022)
摘要
This paper presents a new facial feature extraction method on entropy based fractional fuzzy generalized two-dimensional linear discriminant analysis (EnFFG-2DLDA) where the concept of class uncertainty is incorporated within the generalized two-dimensional linear discriminant analysis. The EnFFG-2DLDA achieves the optimal projection matrix (in both directions) by maximizing the entropy and fuzzy membership value, based on inter-class similarity, while minimizing intra-class discrepancy by integrating weight function. This method ameliorates the shortcomings of 2DLDA method, which fails in case of many real-world situations as it focuses on the global relationship of data, and relies on Gaussian distributed data. Our EnFFG-2DLDA method does not depend on the assumptions that the data distributions and it exploits the intrinsic local structure of the data. Moreover, the F k NN has been incorporated into G-2DLDA algorithm to highlight distinguishable features by redefining the intra- (within) and inter- (between) class scatter matrices along horizontal (row) and vertical (column) directions. The global and per-class mean training vectors are regenerated by combining the fuzzy membership values. The weighting function is associated into inter class scatter matrix, in both direction, to solve contour based problem. Finally, Entropy membership values are specifically used for measuring uncertainty in the special variation in a facial image. Therefore, more significant feature information have been distilled out by combining F k NN, weight function and entropy with the G-2DLDA method, which solves the multi-classification problem. The feasibility of fractional fuzzy G-2DLDA with entropy membership value has been validated over frontal, occluded, and rotationally- angled images with four public standard face databases using RBF neural network. The investigational results demonstrate the substantial accuracy of 99.06% ( k = 6 ) for ORL, 97.81% ( k = 10) for UMIST and 66.04 ( k = 4) for FERET dataset in terms of average recognition accuracy.
更多查看译文
关键词
Entropy,FkNN,Weighting fraction,EnFFG-2DLDA,Membership grade matrix
AI 理解论文
溯源树
样例
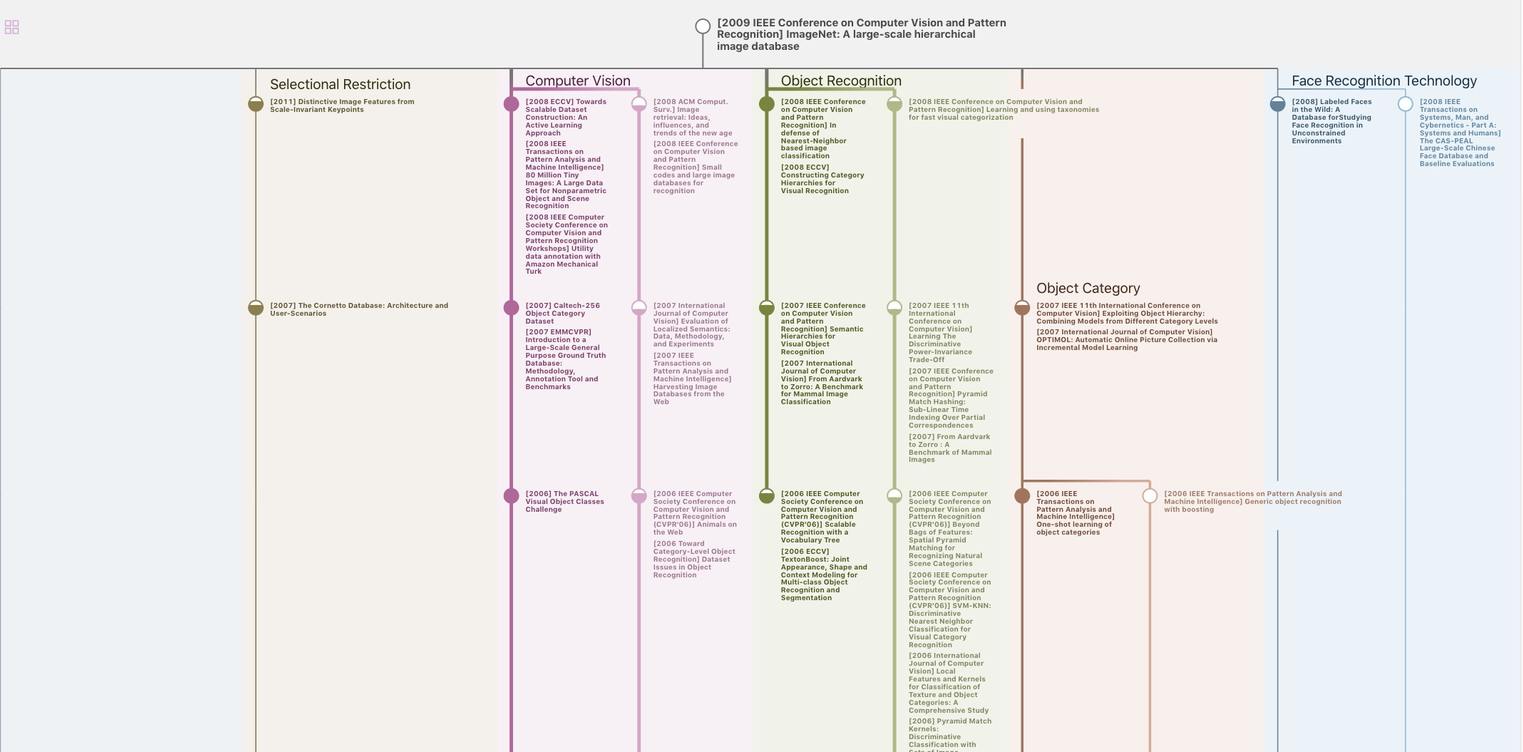
生成溯源树,研究论文发展脉络
Chat Paper
正在生成论文摘要