A Comprehensive Framework for Learning Declarative Action Models
JOURNAL OF ARTIFICIAL INTELLIGENCE RESEARCH(2022)
摘要
A declarative action model is a compact representation of the state transitions of dynamic systems that generalizes over world objects. The specification of declarative action models is often a complex hand-crafted task. In this paper we formulate declarative action models via state constraints, and present the learning of such models as a combinatorial search. The comprehensive framework presented here allows us to connect the learning of declarative action models to well-known problem solving tasks. In addition, our framework allows us to characterize the existing work in the literature according to four dimensions: (1) the target action models, in terms of the state transitions they define; (2) the available learning examples; (3) the functions used to guide the learning process, and to evaluate the quality of the learned action models; (4) the learning algorithm. Last, the paper lists relevant successful applications of the learning of declarative actions models and discusses some open challenges with the aim of encouraging future research work.
更多查看译文
关键词
action,models,learning
AI 理解论文
溯源树
样例
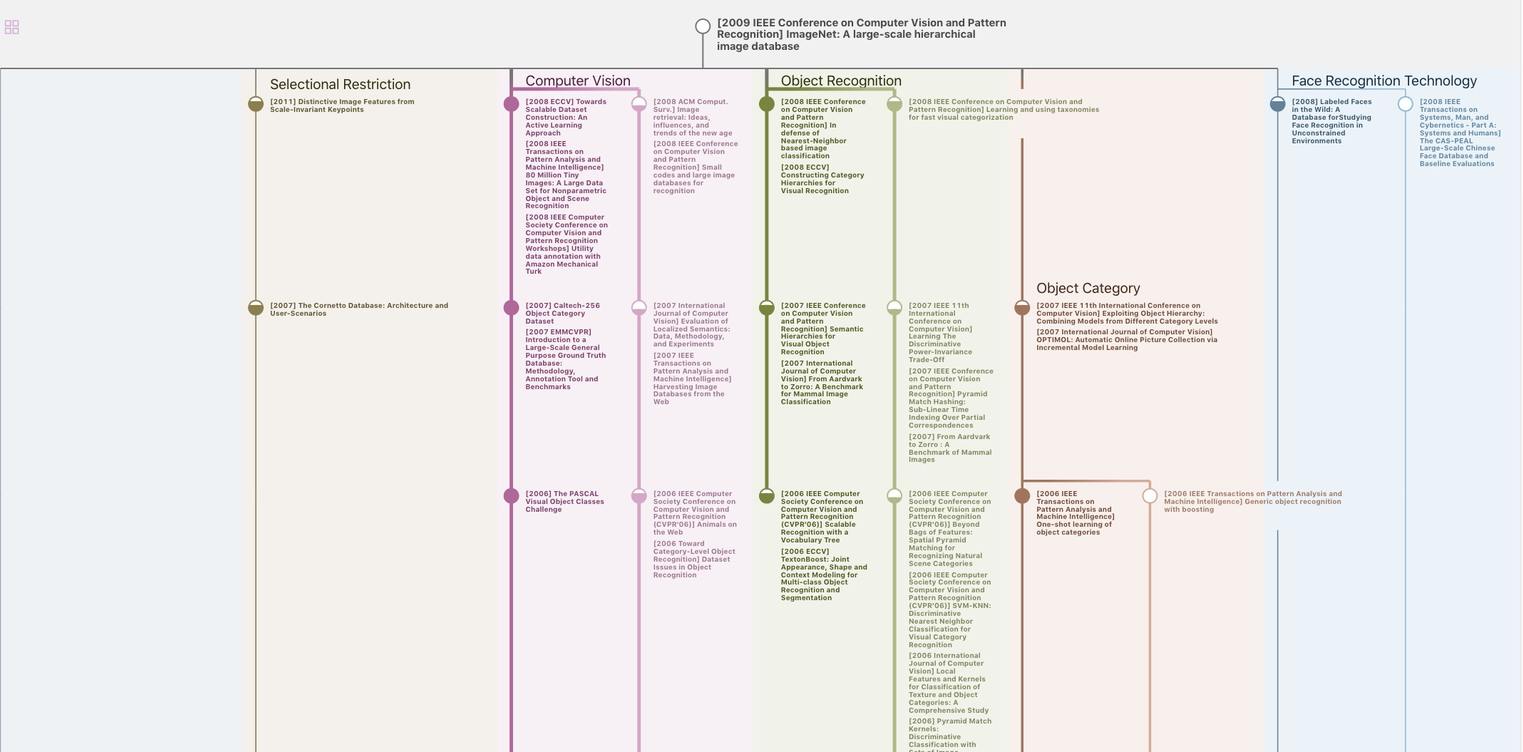
生成溯源树,研究论文发展脉络
Chat Paper
正在生成论文摘要