FGO-Net: Feature and Gaussian Optimization Network for visual saliency prediction
Applied Intelligence(2022)
摘要
Convolutional neural networks (CNNs) have become a major driving force for visual saliency prediction. However, since the features of different layers have diverse characteristics, not all of them are effective for saliency detection, and some even cause interference. To effectively fuse multiscale features from different layers, in this paper, we propose a feature and Gaussian optimization network (FGO-Net) for saliency prediction. Specifically, we first design a novel attention ConvLSTM (ACL) module that contains circular soft layer attention to iteratively reweight multilevel features from different layers. Besides, previous works generally employed Gaussian blur with fixed kernel size after saliency maps generation as post-processing. To this end, we design an adaptive Gaussian blur (AGB) module that can automatically select the appropriate Gaussian kernel size to blur the saliency map without post-processing. Extensive experiments on several public saliency datasets demonstrate that the proposed FGO-Net achieves competitive results in terms of various evaluation metrics.
更多查看译文
关键词
Saliency detection,Artificial intelligence,Convolutional neural network,Attention mechanism
AI 理解论文
溯源树
样例
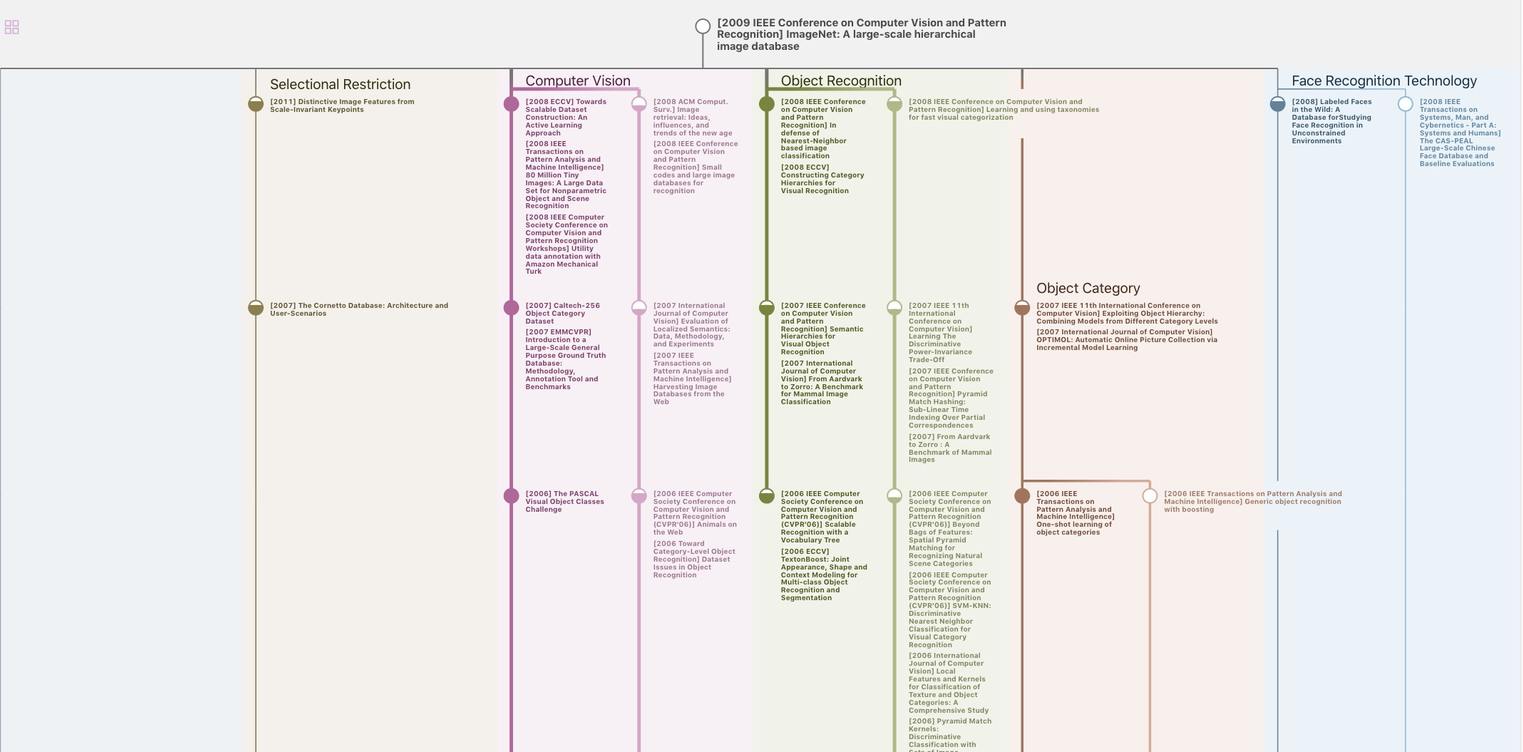
生成溯源树,研究论文发展脉络
Chat Paper
正在生成论文摘要