Data Quality of Automated Comorbidity Lists in Patients With Mental Health and Substance Use Disorders
CIN-COMPUTERS INFORMATICS NURSING(2022)
摘要
EHRs provide an opportunity to conduct research on underrepresented oncology populations with mental health and substance use disorders. However, a lack of data quality may introduce unintended bias into EHR data. The objective of this article is describe our analysis of data quality within automated comorbidity lists commonly found in EHRs. Investigators conducted a retrospective chart review of 395 oncology patients from a safety-net integrated healthcare system. Statistical analysis included kappa coefficients and a condition logistic regression. Subjects were racially and ethnically diverse and predominantly used Medicaid insurance. Weak kappa coefficients (kappa = 0.2-0.39, P < .01) were noted for drug and alcohol use disorders indicating deficiencies in comorbidity documentation within the automated comorbidity list. Further, conditional logistic regression analyses revealed deficiencies in comorbidity documentation in patients with drug use disorders (odds ratio, 11.03; 95% confidence interval, 2.71-44.9; P = .01) and psychoses (odds ratio, 0.04; confidence interval, 0.02-0.10; P < .01). Findings suggest deficiencies in automatic comorbidity lists as compared with a review of provider narrative notes when identifying comorbidities. As healthcare systems increasingly use EHR data in clinical studies and decision making, the quality of healthcare delivery and clinical research may be affected by discrepancies in the documentation of comorbidities.
更多查看译文
关键词
Automated comorbidity lists,Data quality,Drug use disorders,EHRs,Mental health disorders,Substance use disorders
AI 理解论文
溯源树
样例
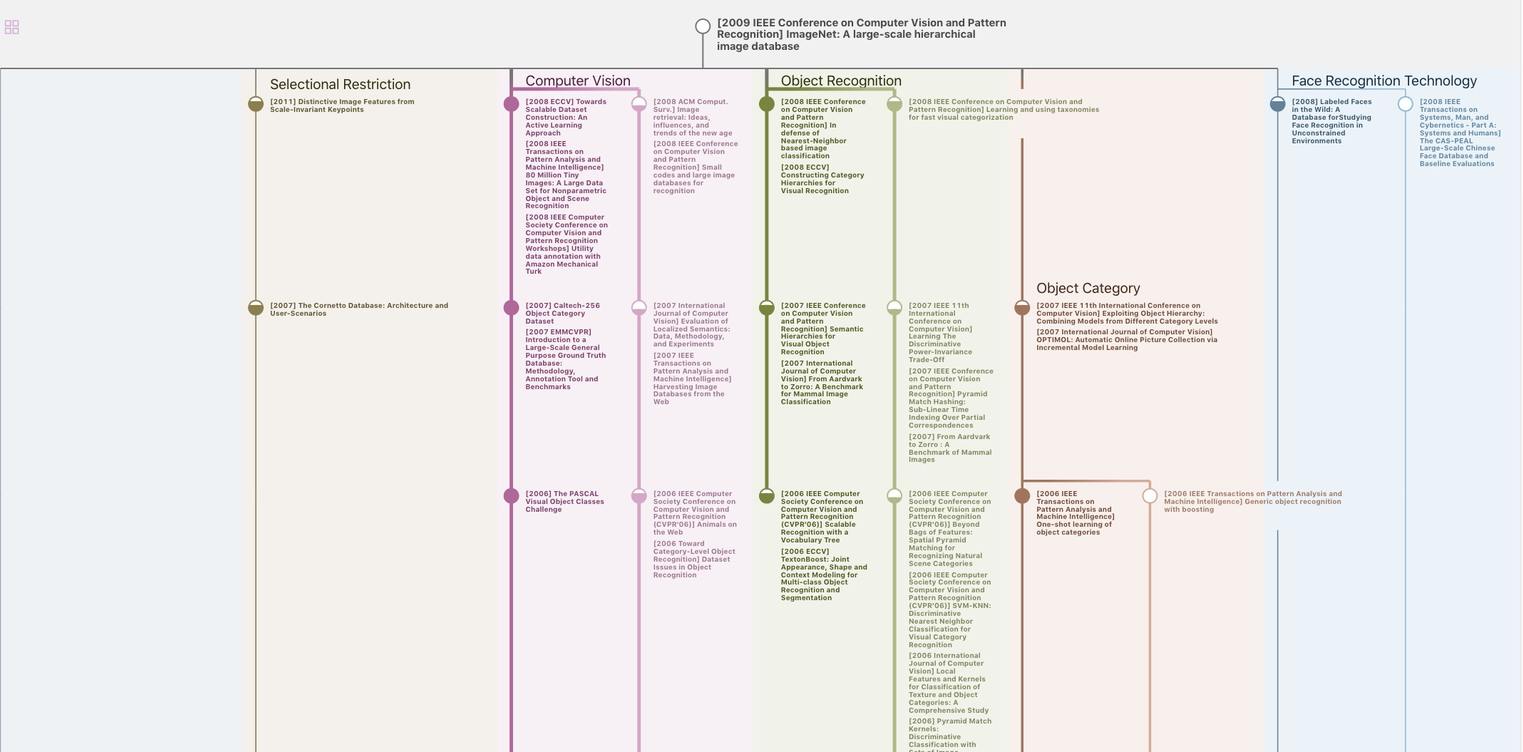
生成溯源树,研究论文发展脉络
Chat Paper
正在生成论文摘要