Robust disturbance classification in power transmission systems with denoising recurrent autoencoders
Sustainable Energy, Grids and Networks(2022)
Abstract
The automated classification of grid disturbances based on phasor measurements is a key technology for the reliable operation of power transmission systems. The predominant use of simulated training data limits the applicability of existing classification approaches due to the missing consideration of measurement errors or data quality issues. To mitigate these shortcomings, this study presents a robust disturbance classification procedure incorporating denoising recurrent autoencoders within a novel two-stage training approach. The developed disturbance classification procedure is evaluated for different noise characteristics and dataset combinations created with an optimization based error model. Experimental results based on a generic power transmission system show superior performance of the proposed two-stage design compared to a conventional, one-stage model training.
MoreTranslated text
Key words
Phasor measurements,Disturbance classification,Denoising autoencoders,Recurrent neural networks,Data quality
AI Read Science
Must-Reading Tree
Example
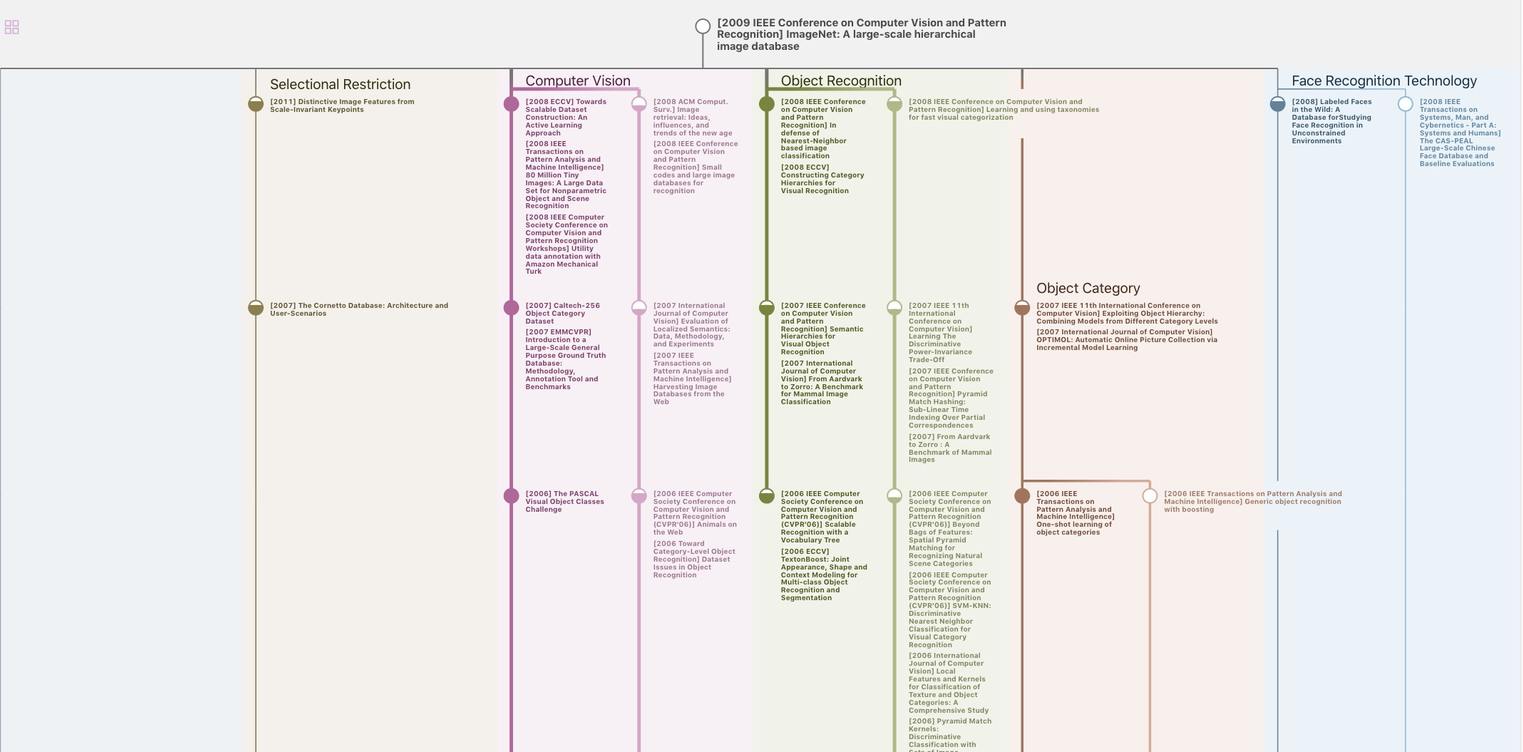
Generate MRT to find the research sequence of this paper
Chat Paper
Summary is being generated by the instructions you defined