Block-sparse sign algorithm and its performance analysis
Digital Signal Processing(2022)
Abstract
System identification is often encountered in applications such as echo cancellation, active noise control, and channel equalization. If the unknown system is sparse, using sparsity-induced methods can improve the convergence performance. Recently, the l2,0-norm constraint was used to derive a block-sparse LMS (BS-LMS) algorithm to accelerate convergence for identifying multi-clustering sparse systems. In some cases, output of the unknown system is contaminated by impulsive noise, and BS-LMS performs poorly or even diverges when identifying such systems. To address this problem, this paper constructs a loss function by combining the absolute error and the l2,0-norm of adaptive filter weight vector, and then uses the subgradient descent method to develop a block-sparse sign algorithm (BS-SA). Its mean and mean-square performance is also analyzed based on the Gaussian-Bernoulli impulsive noise model under some frequently used assumptions. Finally, simulations are performed to test the robustness of BS-SA against impulsive noise and to evaluate the accuracy of theoretical expressions derived for statistical performance.
MoreTranslated text
Key words
Block-sparse system,l2,0-norm optimization,Impulsive noise,Performance analysis
AI Read Science
Must-Reading Tree
Example
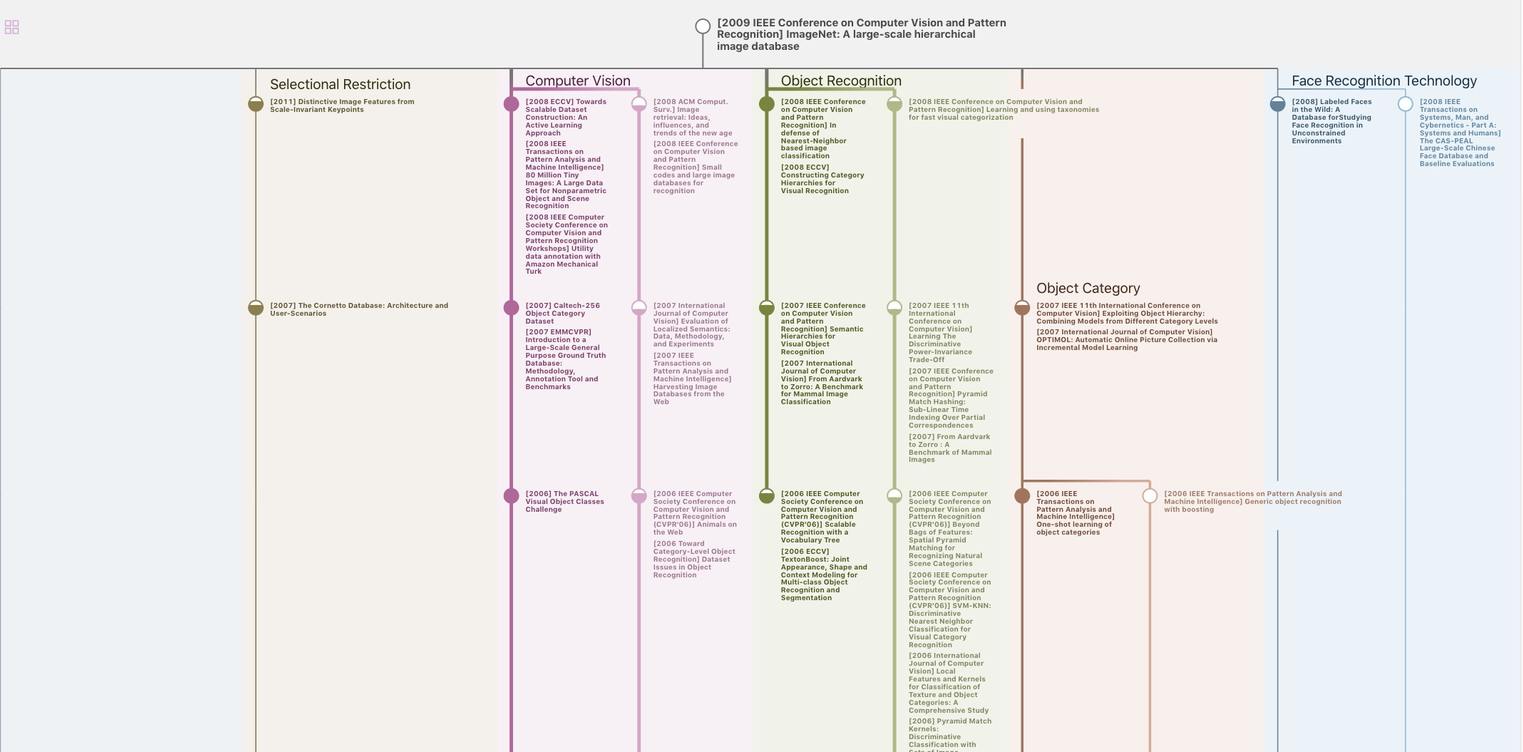
Generate MRT to find the research sequence of this paper
Chat Paper
Summary is being generated by the instructions you defined