Cross-lingual knowledge graph entity alignment based on relation awareness and attribute involvement
Applied Intelligence(2022)
摘要
Entity alignment is an effective means of matching entities from various knowledge graphs (KGs) that represent the equivalent real-world object. With the development of representation learning, recent entity alignment methods learn entity structure representation by embedding KGs into a low-dimensional vector space, and then entity alignment relies on the distance between entity vectors. In addition to the graph structures, relations and attributes are also critical to entity alignment. However, most existing approaches ignore the helpful features included in relations and attributes. Therefore, this paper presents a new solution RAEA ( R elation Awareness and A ttribute Involvement for E ntity A lignment), which includes relation and attribute features. Relation representation is incorporated into entity representation by Dual-Primal Graph CNN (DPGCNN), which alternates convolution-like operations on the original graph and its dual graph. Structure representation and attribute representation are learned by graph convolutional networks (GCNs). To further enrich the entity embedding, we integrate the textual information of the entity into the entity graph embedding. Moreover, we fine-tune the entity similarity matrix by integrating fine-grained features. Experimental results on three benchmark datasets from real-world KGs show that our approach has superior performance to other representative entity alignment approaches in most cases.
更多查看译文
关键词
Entity alignment,Knowledge graph,Representation learning,Relation,Attribute,Textual information
AI 理解论文
溯源树
样例
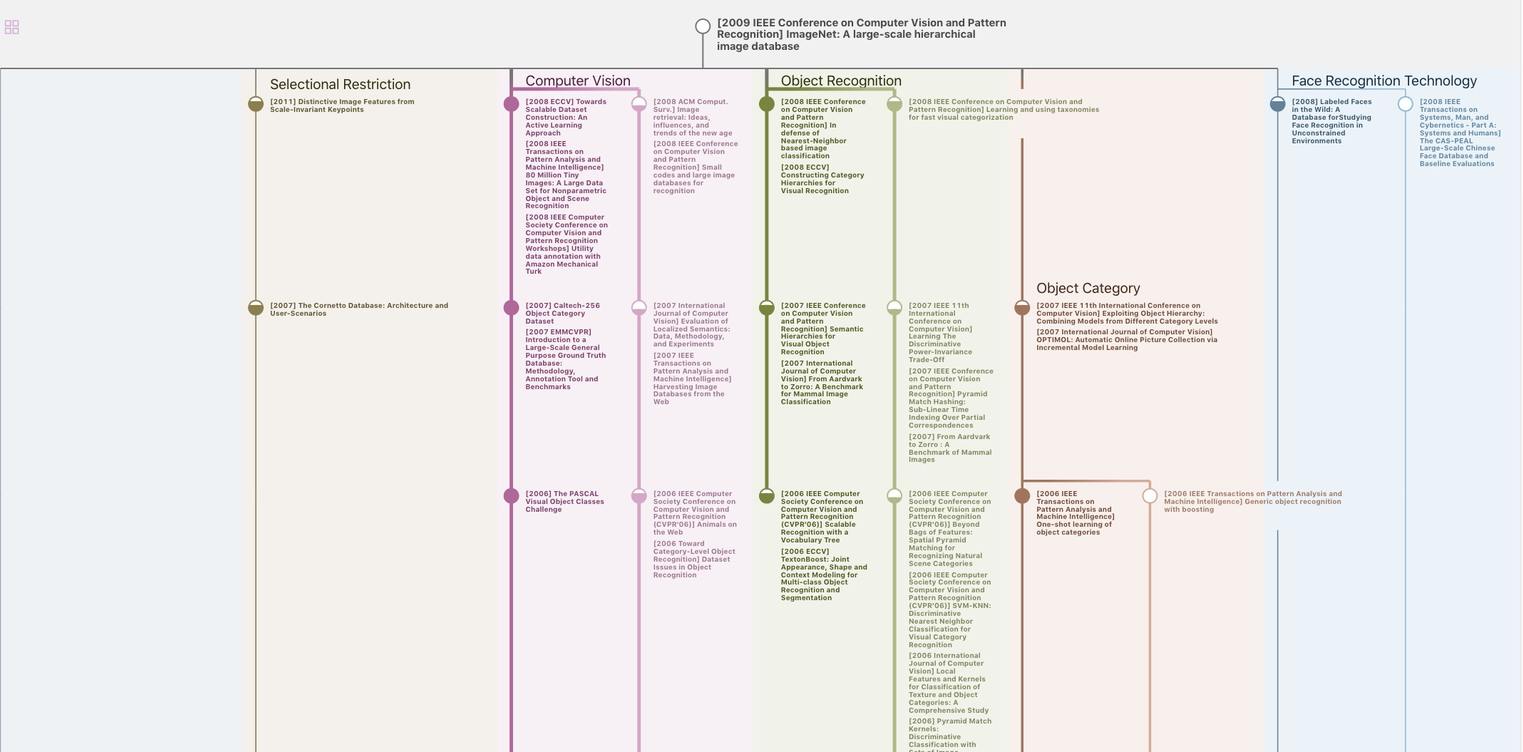
生成溯源树,研究论文发展脉络
Chat Paper
正在生成论文摘要