Density peak clustering for shadow detection of soil image
SIGNAL IMAGE AND VIDEO PROCESSING(2022)
摘要
Shadow detection is a significant preprocessing work that soil species are classified with machine vision because shadow may affect the accuracy of identifying soil species. To judge the number of shadows in a soil image used for soil species identification, density peak clustering for shadow detection of soil image (DPCSDSI) is proposed to segment shadows. Firstly, the Bright histogram of a soil image is built and its Gaussian smoothing is done. Then a new parameter-free density formula and decision value measure are reconstructed. Because shadow detection is a typical binary classification problem, the two x_i points with the largest decision value γ _i are chosen as the cluster centers of shadow and non-shadow. And the clustering labels gray levels are initialized by the original DPC algorithm with the cluster centers. Next, the Bright histogram is fitted with Fourier series to search valleys and update the clustering labels. Finally, the clustering labels are corrected with uncertainty coefficient until Eq. ( 21 ) is satisfied, which finishes the segmentation of shadow and non-shadow in a soil image. The simulation results show that DPCSDSI is better than the contrast algorithms and its average brightness standard deviations of the shadow and non-shadow are, respectively, 20.5484 and 20.9024. It can detect adaptively shadows in a soil image and there is not the “domino” error propagation in it, so it is effective.
更多查看译文
关键词
Image segmentation,Shadow detection,Density peak clustering (DPC),Soil images
AI 理解论文
溯源树
样例
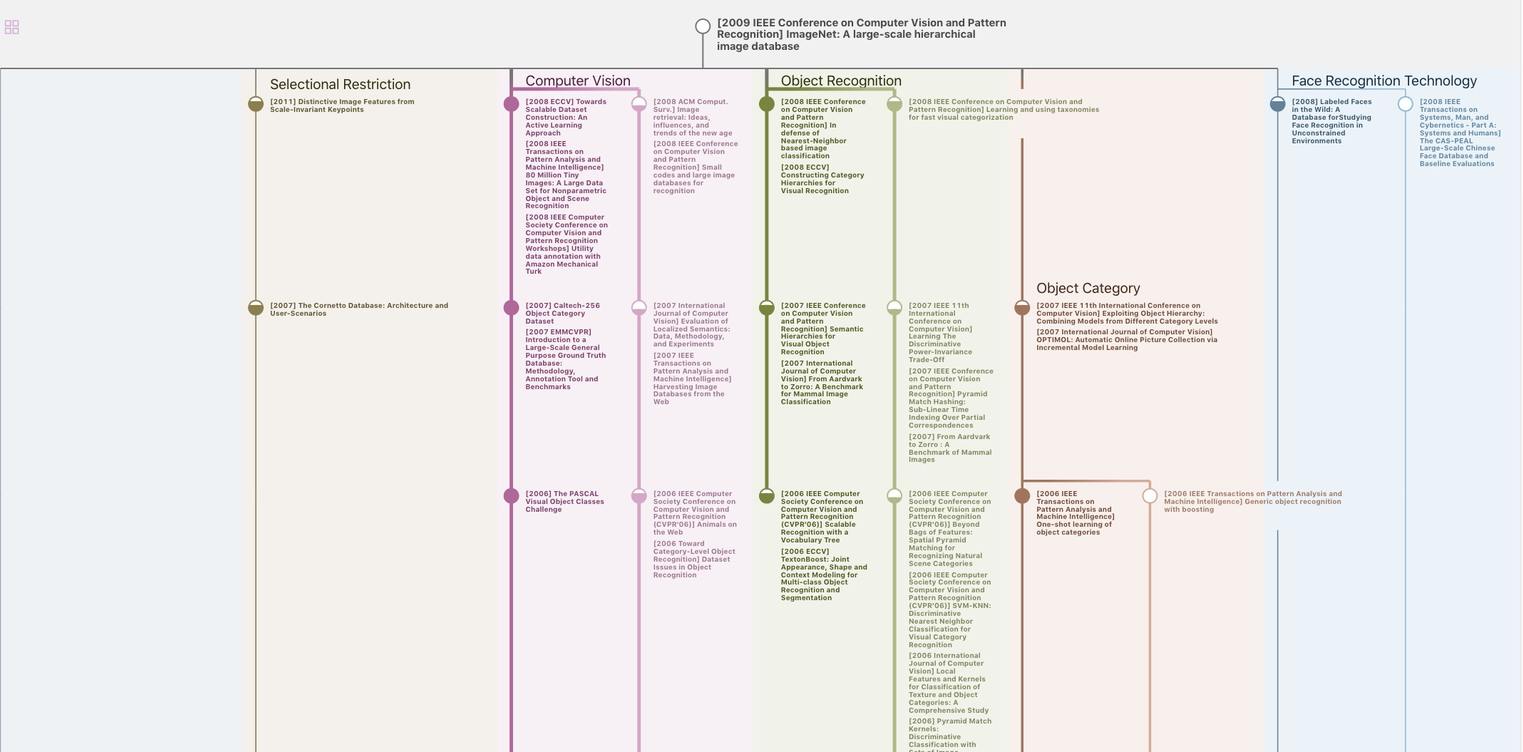
生成溯源树,研究论文发展脉络
Chat Paper
正在生成论文摘要