Feasibility of Image Analysis Coupled with Machine Learning for Detection and Quantification of Extraneous Water in Milk
Food analytical methods(2022)
摘要
Milk is one of the most vulnerable food items for fraudulent activities such as dilution with water. Such malpractices have the potential to reduce the role of milk for food and nutrition security unless monitored and corrected. Research on simple and rapid techniques that allow users to diagnose adulteration in milk has the potential to ease the diagnosis of adulterated milk. This study proposed a procedure based on digital image analysis coupled with machine learning techniques for the detection of water adulteration in milk. Among the compared machine learning tools, SVM-based class prediction model performed best in classifying adulterated milk samples based on the amount of added water with 94% of total accuracy and 97% precision. In an attempt to the quantitative determination of the amount of added water, XGB-based regression model was able to achieve the best prediction performance compared to PCR, PLSR, SVMR, and ANNR, with R 2 ( P ) and RMSEP of 0.83 and 5.94, respectively. The proposed technique can be used as an alternative approach for the rapid determination of milk adulteration with water.
更多查看译文
关键词
Milk adulteration,Multivariate classification,Support vector machine,Validation,XGB
AI 理解论文
溯源树
样例
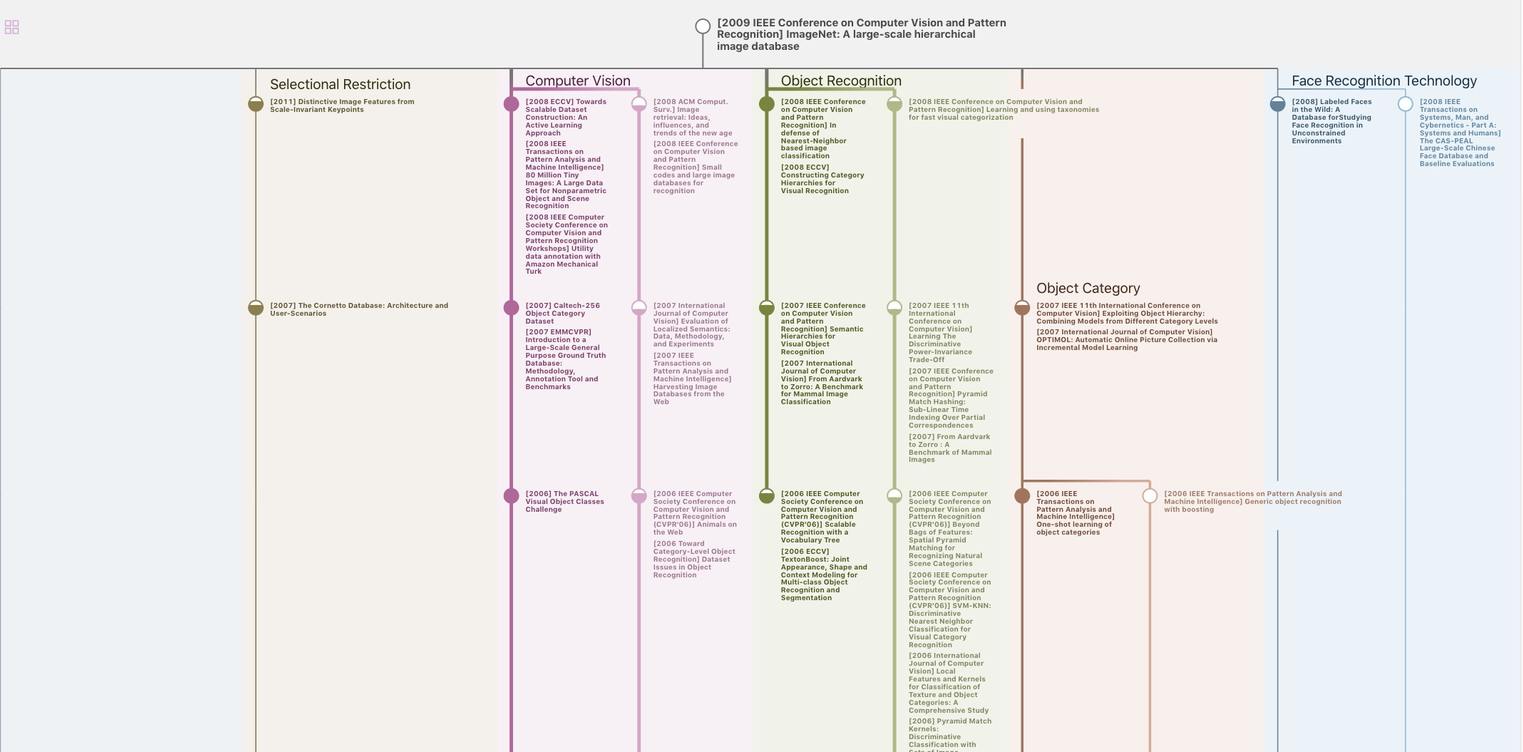
生成溯源树,研究论文发展脉络
Chat Paper
正在生成论文摘要