Adversarial Attacks on Deep-Learning RF Classification in Spectrum Monitoring with Imperfect Bandwidth Estimation
2022 IEEE WIRELESS COMMUNICATIONS AND NETWORKING CONFERENCE (WCNC)(2022)
摘要
In a spectrum-monitoring scenario, a monitor will attempt to intercept and classify a signal. If the monitor uses a Convolutional Neural Network (CNN) for classification, the intercepted signal can frustrate classification attempts by employing an adversarial waveform. An adversarial waveform is a small additive perturbation at the transmitter, and is generated similarly to adversarial attacks against image classifiers. We demonstrate that without foreknowledge of the CNN employed at the monitor the communication system can develop such an adversarial waveform and deploy it thus transferring the attack. The adversarial waveform is created by constraining the signal-to-interference ratio at the transmitter, which has the dual benefits of making the adversarial waveform easy to deploy and mitigates impairment to the communications link. We also demonstrate the vulnerability of a spectrum monitoring system to this type of attack as a function of symbol rate uncertainty, where the monitor does not have an exact estimate of the symbol rate employed by the communications link. The spectrum monitor becomes more susceptible to the attack as bandwidth is increased.
更多查看译文
关键词
Spectrum Monitoring, Modulation Classification, Adversarial Attacks, Deep Learning
AI 理解论文
溯源树
样例
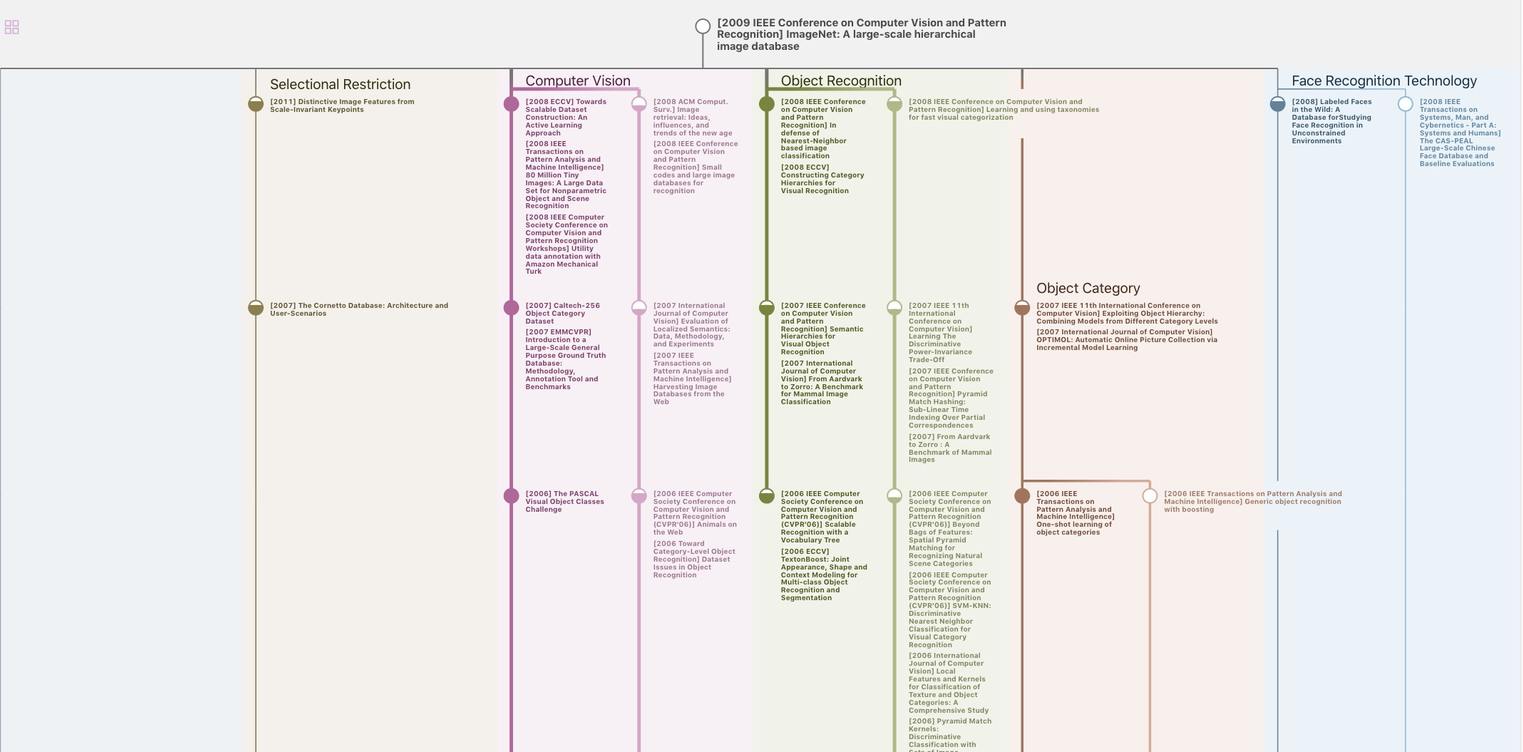
生成溯源树,研究论文发展脉络
Chat Paper
正在生成论文摘要