WRAP: Weight RemApping and Processing in RRAM-based Neural Network Accelerators Considering Thermal Effect
PROCEEDINGS OF THE 2022 DESIGN, AUTOMATION & TEST IN EUROPE CONFERENCE & EXHIBITION (DATE 2022)(2022)
摘要
Resistive random-access memory (RRAM) has shown great potential for computing in memory (CIM) to support the requirements of high memory bandwidth and low power in neuromorphic computing systems. However, the accuracy of RRAM-based neural network (NN) accelerators can degrade significantly due to the intrinsic statistical variations of the resistance of RRAM cells, as well as the negative effects of high temperatures. In this paper, we propose a subarray-based thermal-aware weight remapping and processing framework (WRAP) to map the weights of a neural network model into RRAM subarrays. Instead of dealing with each weight individually, this framework maps weights into subarrays and performs subarray-based algorithms to reduce computational complexity while maintaining accuracy under thermal impact. Experimental results demonstrate that using our framework, inference accuracy losses of four DNN models are less than 2% compared to the ideal results and 1% with compensation applied even when the surrounding temperature is around 360K.
更多查看译文
关键词
computational complexity,thermal impact,inference accuracy losses,WRAP,RRAM-based neural network accelerators,thermal effect,resistive random-access memory,high memory bandwidth,neuromorphic computing systems,intrinsic statistical variations,RRAM cells,neural network model,RRAM subarrays,subarray-based thermal-aware weight remapping and processing framework,subarray-based algorithms
AI 理解论文
溯源树
样例
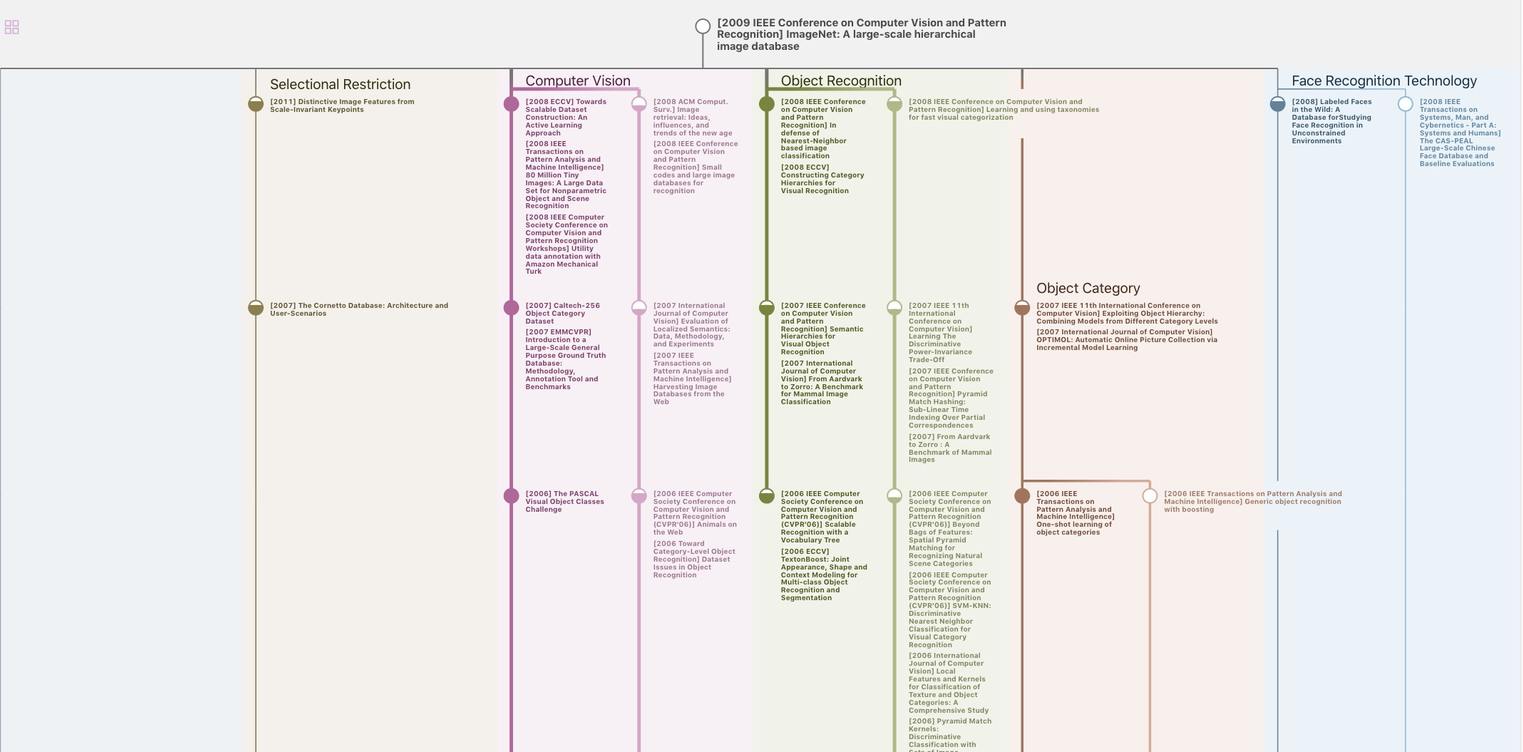
生成溯源树,研究论文发展脉络
Chat Paper
正在生成论文摘要