Explanations of Machine Learning Models in Repeated Nested Cross-Validation: An Application in Age Prediction Using Brain Complexity Features
APPLIED SCIENCES-BASEL(2022)
Abstract
SHAP (Shapley additive explanations) is a framework for explainable AI that makes explanations locally and globally. In this work, we propose a general method to obtain representative SHAP values within a repeated nested cross-validation procedure and separately for the training and test sets of the different cross-validation rounds to assess the real generalization abilities of the explanations. We applied this method to predict individual age using brain complexity features extracted from MRI scans of 159 healthy subjects. In particular, we used four implementations of the fractal dimension (FD) of the cerebral cortex-a measurement of brain complexity. Representative SHAP values highlighted that the most recent implementation of the FD had the highest impact over the others and was among the top-ranking features for predicting age. SHAP rankings were not the same in the training and test sets, but the top-ranking features were consistent. In conclusion, we propose a method-and share all the source code-that allows a rigorous assessment of the SHAP explanations of a trained model in a repeated nested cross-validation setting.
MoreTranslated text
Key words
brain complexity, explainable AI, fractal dimension, machine learning, SHAP
AI Read Science
Must-Reading Tree
Example
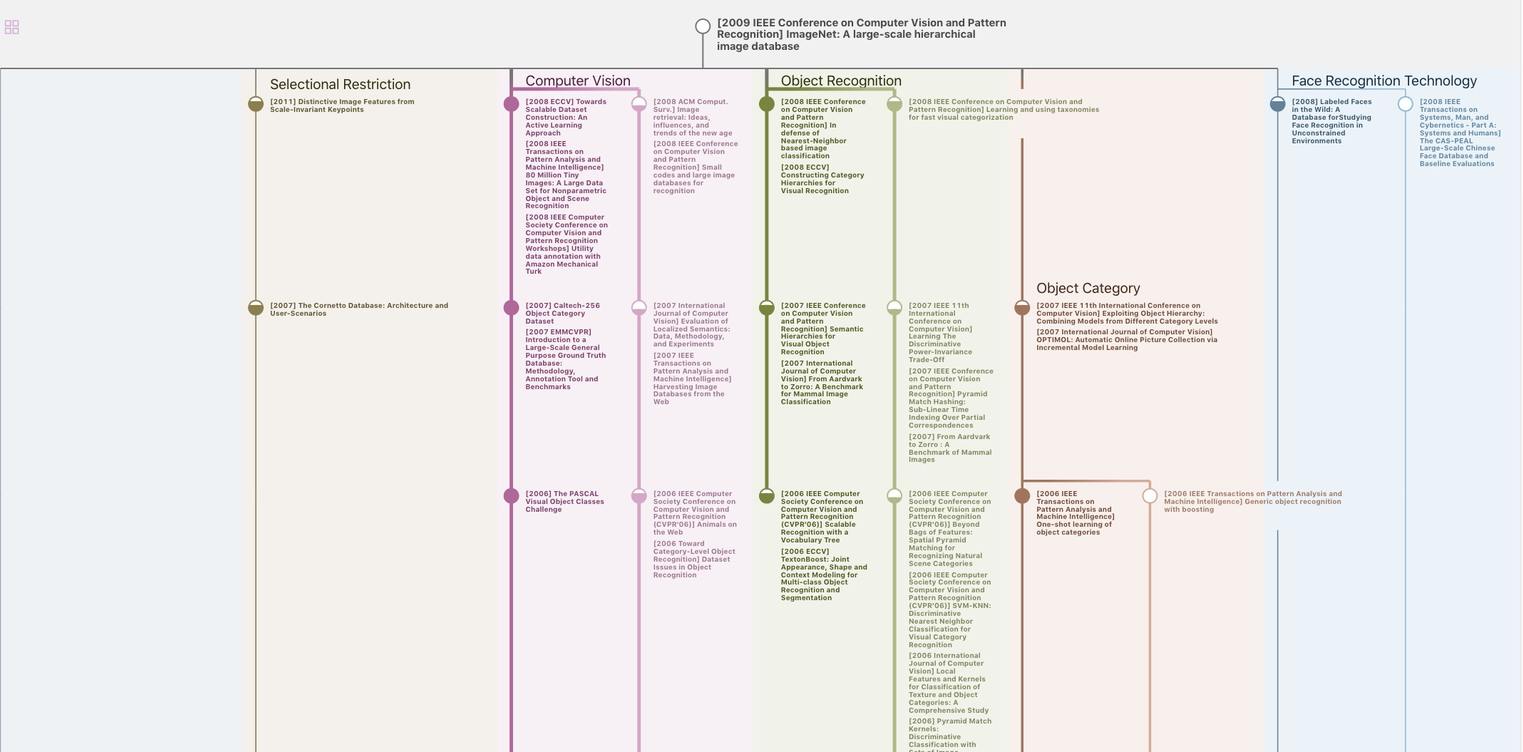
Generate MRT to find the research sequence of this paper
Chat Paper
Summary is being generated by the instructions you defined