Machine Learning Prediction of Superconducting Critical Temperature through the Structural Descriptor
JOURNAL OF PHYSICAL CHEMISTRY C(2022)
摘要
Superconductivity allows electric conductance with no energy losses when the ambient temperature drops below a critical value (T-c). Currently, the machine learning (ML)-based prediction of potential superconductors has been limited to chemical formulas without explicit treatment of material structures. Herein, we implement an efficient structural descriptor, the smooth overlap of atomic position (SOAP), into the ML models to predict the T-c values with explicit atomic structural information. Using a data set containing 5713 compounds, our ML models with the SOAP descriptor achieved a 92.9% prediction accuracy of coefficient of determination (R-2) score via rigorous multialgorithm cross-verification procedures, exceeding the 86.3% accuracy record without atomic structure information. Several new high-temperature superconductors with T-c values over 90 K were predicted using the SOAP-assisted ML model. This study provides insights into the structure-property relationship superconductors.
更多查看译文
AI 理解论文
溯源树
样例
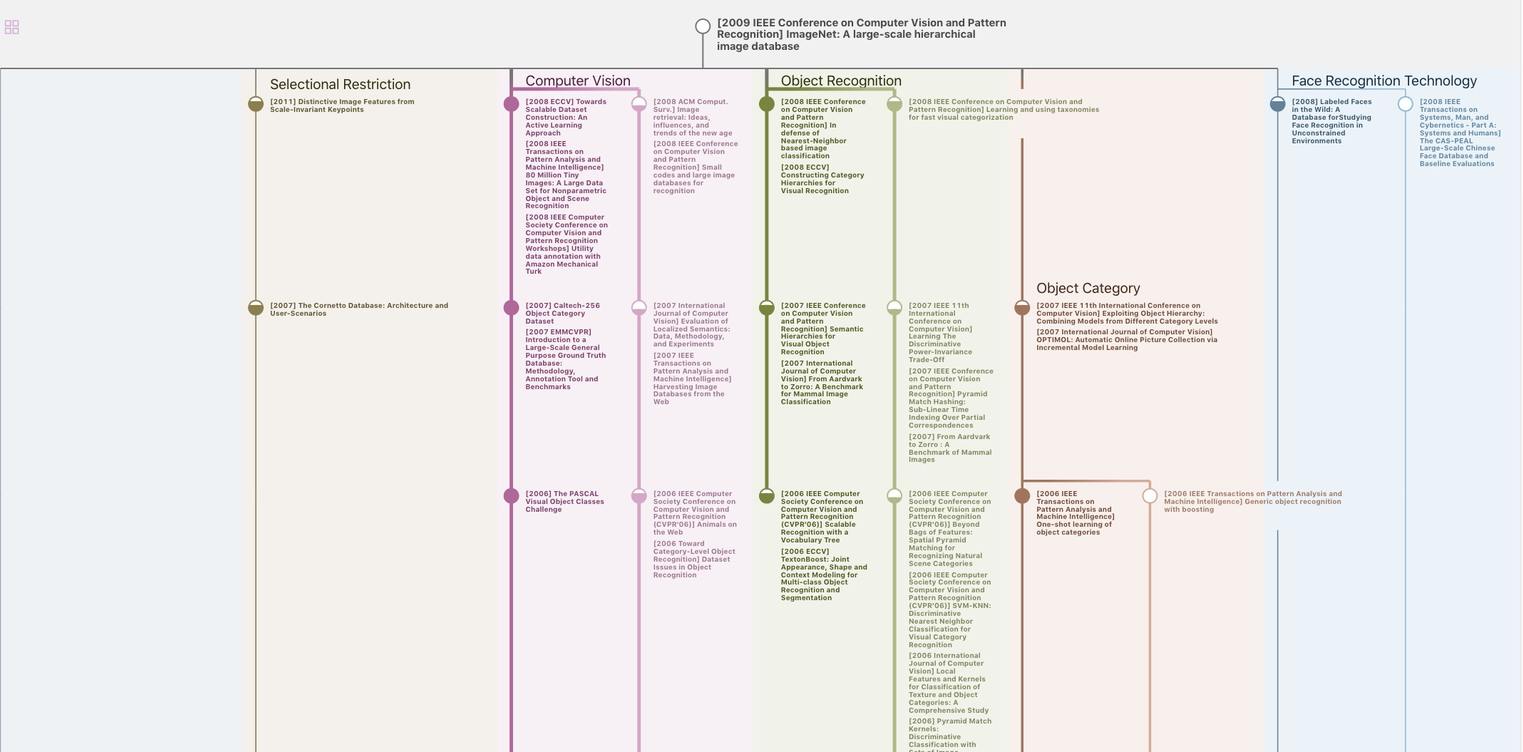
生成溯源树,研究论文发展脉络
Chat Paper
正在生成论文摘要