F-CBR: An Architecture for Federated Case-Based Reasoning
IEEE ACCESS(2022)
摘要
Case-based reasoning (CBR) is a problem-solving methodology in artificial intelligence that attempts to solve new problems using past experiences known as cases. Experiences collected in a single case base from an institution or geographical region are seldom sufficient to solve diverse problems, especially in rare situations. Additionally, many institutions do not promote peer-to-peer (p2p) communication or encourage data sharing through such networks to retain autonomy. The paper proposes a federated CBR (F-CBR) architecture to address these challenges. F-CBR enables solving new problems based on similar cases from multiple autonomous CBR systems without p2p communication. We also designed an algorithm to minimize (irrelevant or unsolicited) data sharing in an F-CBR system. We extend the F-CBR design to support institutions with organizational or geographical hierarchies. The F-CBR architecture was implemented and evaluated on two public datasets and a private real-world (non-specific musculoskeletal disorder patient) dataset. The findings demonstrate that the retrieval quality of F-CBR systems is comparable to or better than a single CBR system that persists all the cases on a centralized case base. F-CBR systems address data privacy by incorporating the data minimization principle. We foresee F-CBR as a viable real-world design that can aid in federating legacy CBR systems with minimal or no changes. The CBR systems used in this study are shared on GitHub to support reproducibility.
更多查看译文
关键词
Cognition, Artificial intelligence, Data privacy, Privacy, Collaborative work, Regulation, Peer-to-peer computing, Case-based reasoning, data minimization, data privacy, data silos, decision support systems, federated architecture, federated case-based reasoning
AI 理解论文
溯源树
样例
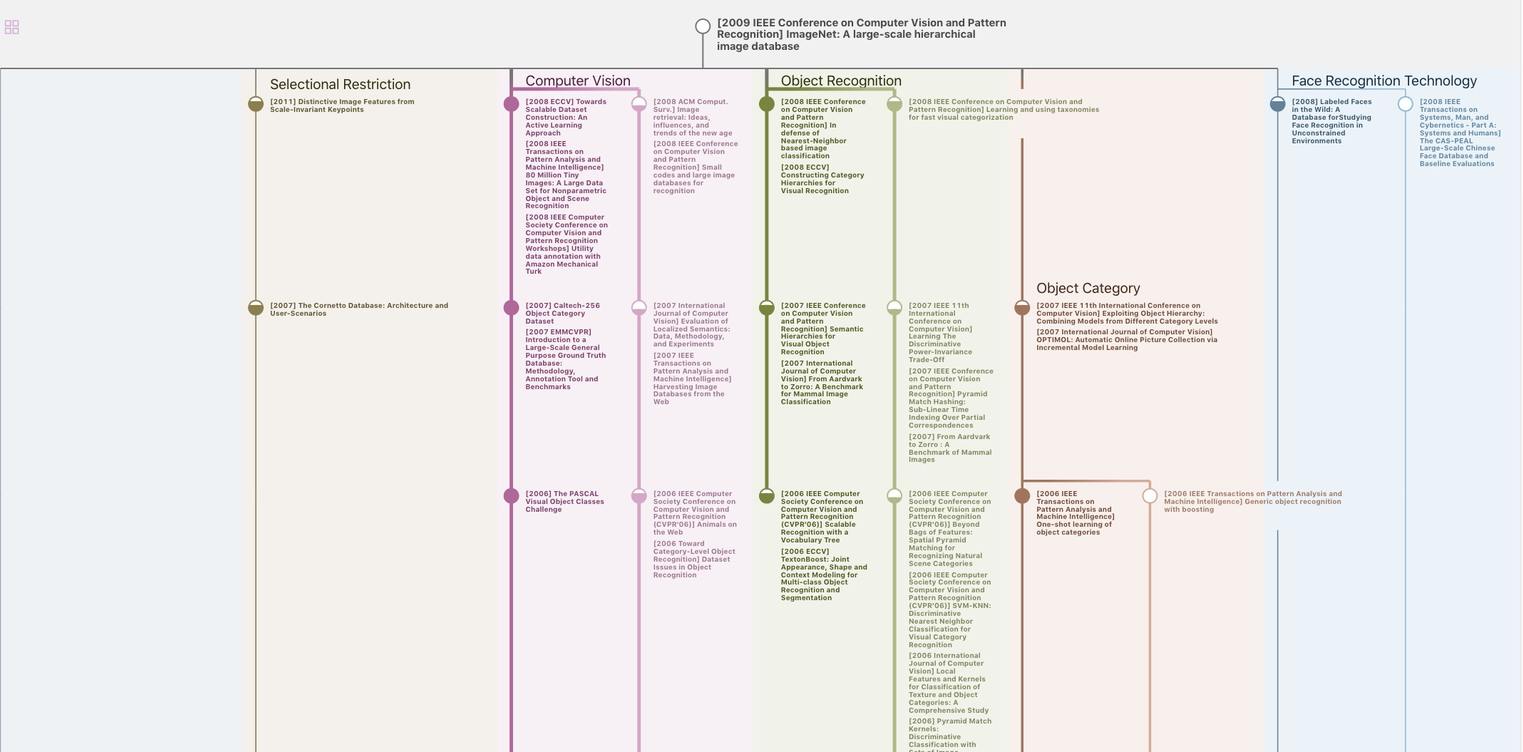
生成溯源树,研究论文发展脉络
Chat Paper
正在生成论文摘要