Ising-CIM: A Reconfigurable and Scalable Compute Within Memory Analog Ising Accelerator for Solving Combinatorial Optimization Problems
IEEE Journal of Solid-State Circuits(2022)
摘要
Combinatorial optimization problems (COPs) find applications in real-world scientific, industrial, and societal scenarios. Such COPs are computationally NP-hard, and performing an exhaustive brute force search for the optimal solution becomes untenable as the COP size increases. To expedite the COP computation, the Ising model formalism is used, which abstracts spin dynamics in a ferromagnet. The spins are orientated to reach the minimum energy state, representing the optimum COP solution. Previous Ising engine designs utilized dedicated annealing processors or additional digital arithmetic circuits next to the memory bitcells. These custom circuits or processors cannot be repurposed for other applications, incurring significant area and power overhead. In contrast to the prior approaches, this work presents a reconfigurable and scalable compute-within-memory analog approach for Ising computation (called Ising-CIM). This area-efficient approach repurposes existing embedded memory bitcell columns and peripheral circuits to perform analog domain Hamiltonian calculations on the bitlines minimizing area and power overhead significantly. A 13.18-Kb silicon prototype, implemented in a 65-nm CMOS process, demonstrates the Ising-CIM concept and functionality using a 100
$\times $
64 pixel image in a max-cut COP. The Ising-CIM design achieves 48-
$\mu \text{m}~^{\mathrm{ 2}}$
/spin unit spin area and
$1091\times $
speedup in annealing time compared to the CPU.
更多查看译文
关键词
Analog computation,compute-in-memory,Hamiltonian,hardware accelerator,Ising model,max-cut problem,simulated annealing
AI 理解论文
溯源树
样例
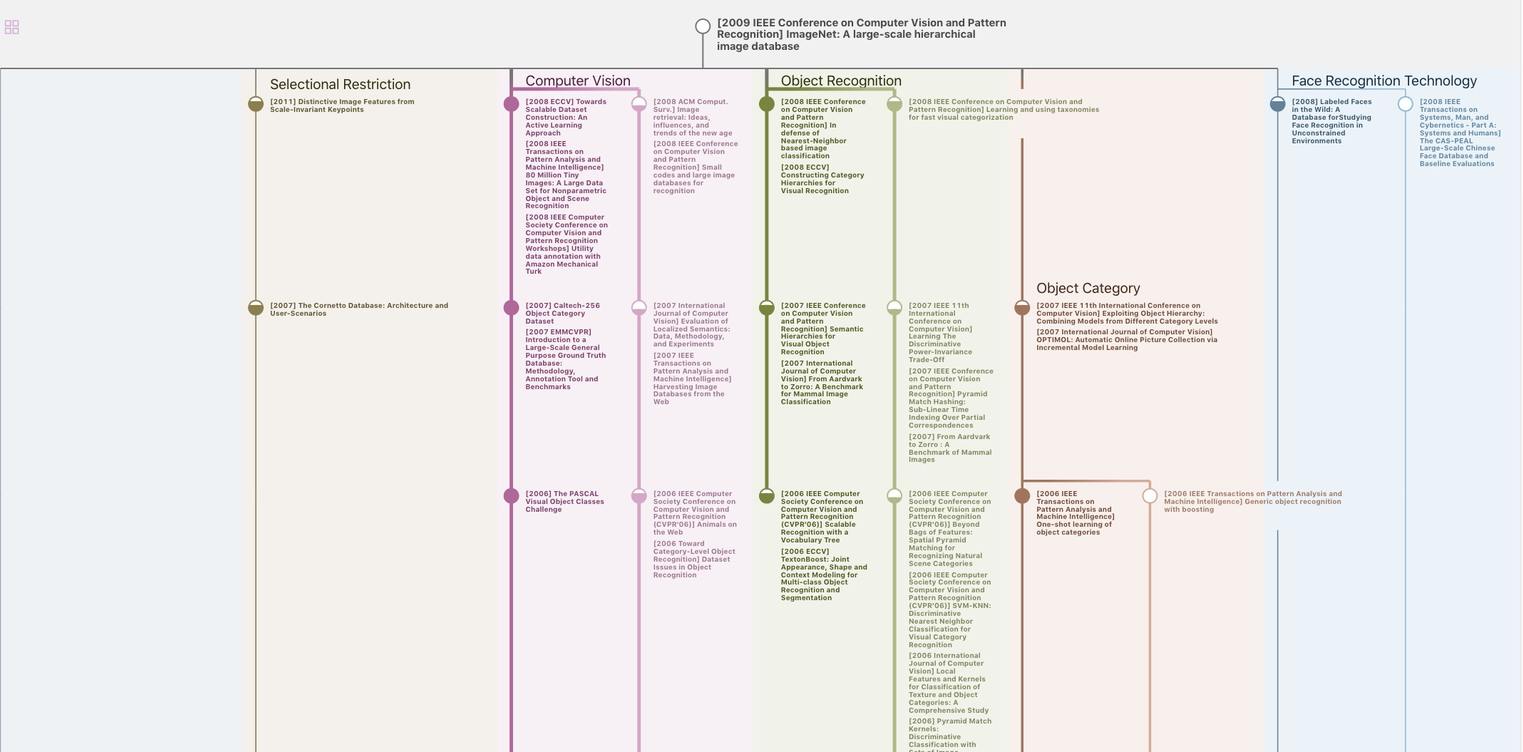
生成溯源树,研究论文发展脉络
Chat Paper
正在生成论文摘要