Two-stage semi-supervised learning inversion for reservoir physical parameters
Journal of Petroleum Science and Engineering(2022)
摘要
Reservoir physical parameters are crucial indexes for reservoir description. Deep learning is a data-driven algorithm, which may avoid some issues in conventional deterministic and stochastic inversion methods, such as cumulative and approximate errors. We develop a two-stage semi-supervised learning inversion method to predict porosity, shale content, and water saturation. Firstly, an inversion network is designed to predict physical parameters from pre-stack seismic data and initial models. The Spatio-temporal characteristics of seismic data and the low-frequency structural information of the initial model can be utilized efficiently by the network. In addition, considering the high production cost of label data in exploration geophysics, we construct a semi-supervised learning inversion workflow to relieve the dependence on the label in training. The rock physics model and seismic convolution forward model are invoked as a forward process in the workflow. Finally, we propose a two-stage inversion strategy to deal with the inversion of physical parameters with different data characteristics. The proposed method is applied to a field survey successfully. A high-resolution and high-accuracy inversion result can be obtained with our method, and potential locations of the gas reservoir can be indicated by prediction results. Meanwhile, the comparisons of the two stages demonstrate that illusions in predicted water saturation can be eliminated effectively.
更多查看译文
关键词
Deep learning,Semi-supervised learning,Reservoir physical parameters inversion,Rock physics
AI 理解论文
溯源树
样例
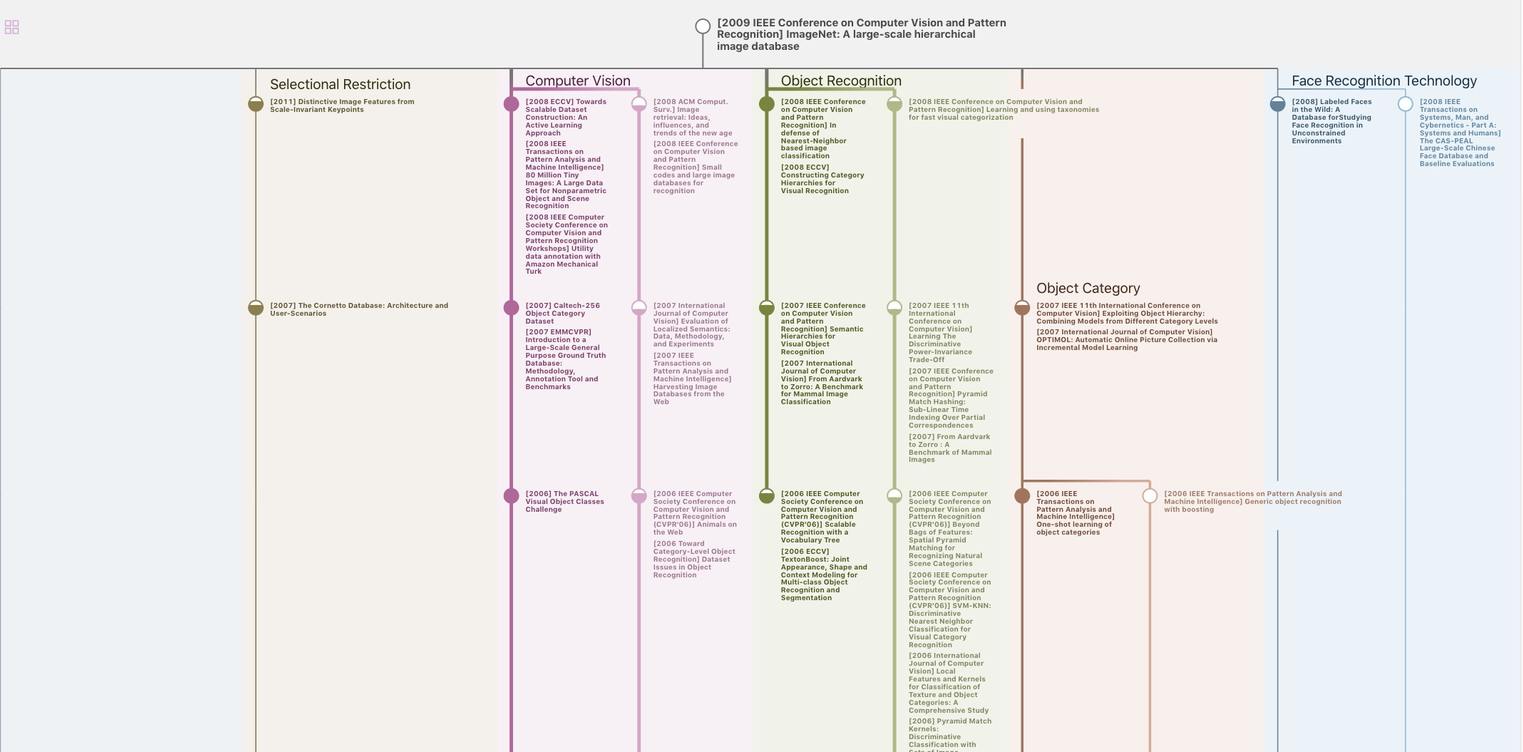
生成溯源树,研究论文发展脉络
Chat Paper
正在生成论文摘要