A Discovery Strategy for Active Compounds of Chinese Medicine Based on the Prediction Model of Compound-Disease Relationship
JOURNAL OF ONCOLOGY(2022)
Abstract
An accurate characterization of diseases and compounds is the key to predicting the compound-disease relationship (CDR). However, due to the difficulty of a comprehensive description of CDR, the accuracy of traditional drug development models for large-scale CDR prediction is usually unsatisfactory. In order to solve this problem, we propose a new method that integrates the molecular descriptors of compounds and the symptom descriptors of diseases to build a CDR two-dimensional matrix to predict candidate active compounds. The Matlab software draws grayscale images of CDRs, which are used as a benchmark dataset for training convolutional neural network (CNN) models. The trained model is used to predict candidate antitumor active compounds. Among the AlexNet and GoogLeNet models, we selected the GoogLeNet model for the prediction of active compounds in Chinese medicine, and its Acc, Sen, Pre, F-measure, MCC, and AUC are 0.960, 0.956, 0.965, 0.960, 0.920, and 0.964, respectively. In the prediction results of compounds, 1624 candidate CDRs were found in 124 Chinese medicines. Among them, we obtained 31 features of candidate antitumor active compounds. This method provides new insights for the discovery of candidate active compounds in Chinese medicine.
MoreTranslated text
AI Read Science
Must-Reading Tree
Example
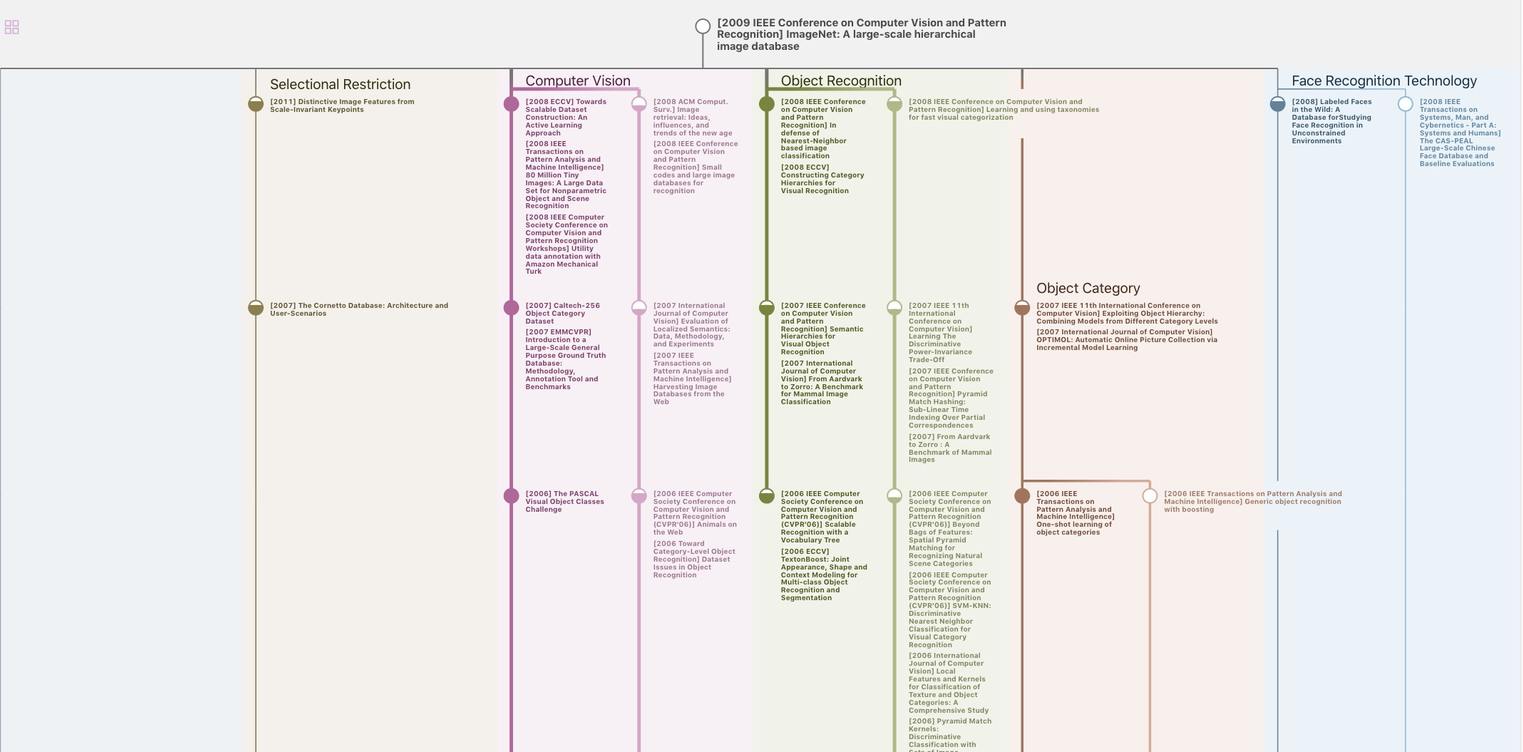
Generate MRT to find the research sequence of this paper
Chat Paper
Summary is being generated by the instructions you defined