Multi-Task Conformer with Multi-Feature Combination for Speech Emotion Recognition
SYMMETRY-BASEL(2022)
摘要
Along with automatic speech recognition, many researchers have been actively studying speech emotion recognition, since emotion information is as crucial as the textual information for effective interactions. Emotion can be divided into categorical emotion and dimensional emotion. Although categorical emotion is widely used, dimensional emotion, typically represented as arousal and valence, can provide more detailed information on the emotional states. Therefore, in this paper, we propose a Conformer-based model for arousal and valence recognition. Our model uses Conformer as an encoder, a fully connected layer as a decoder, and statistical pooling layers as a connector. In addition, we adopted multi-task learning and multi-feature combination, which showed a remarkable performance for speech emotion recognition and time-series analysis, respectively. The proposed model achieves a state-of-the-art recognition accuracy of 70.0 +/- 1.5% for arousal in terms of unweighted accuracy on the IEMOCAP dataset.
更多查看译文
关键词
speech emotion recognition, arousal, valence, spoken language understanding
AI 理解论文
溯源树
样例
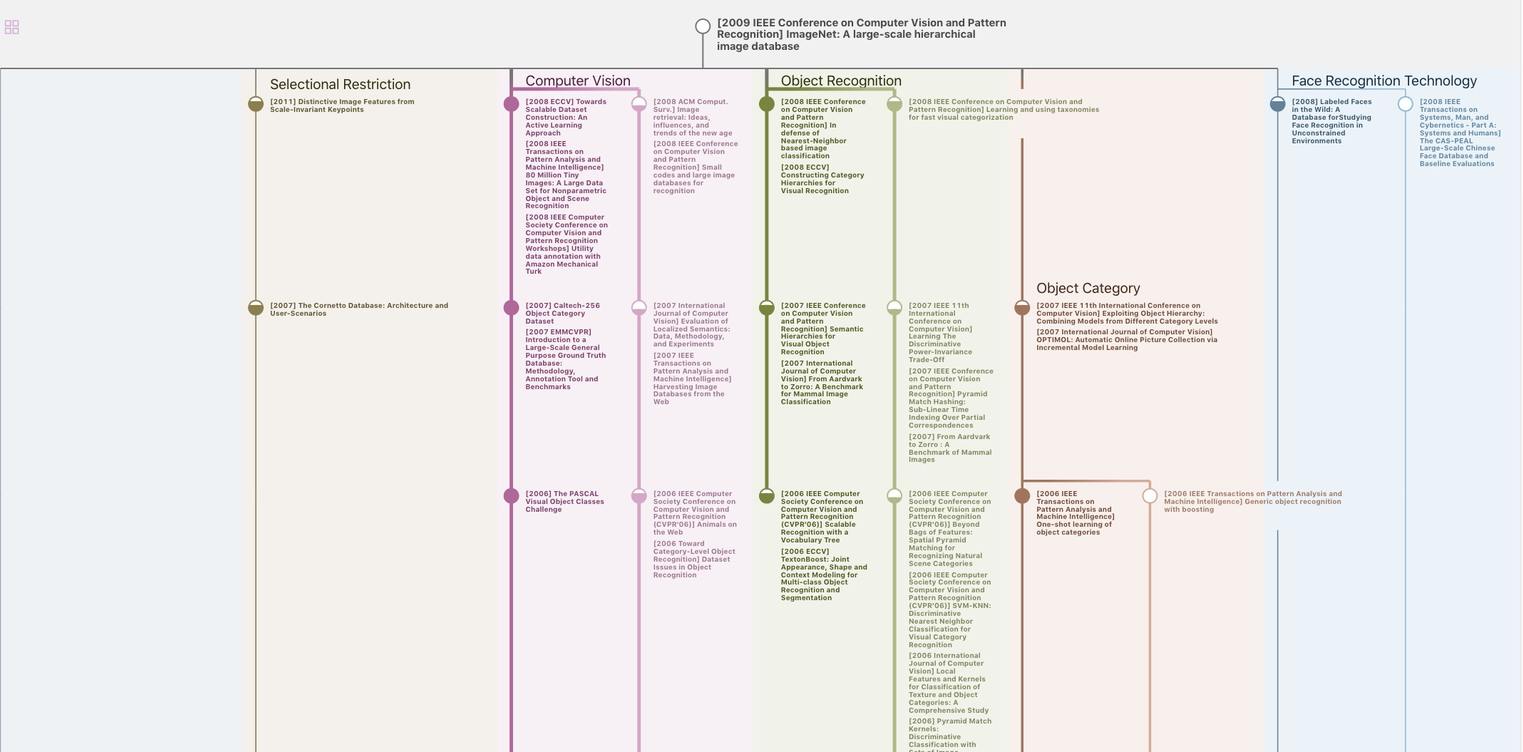
生成溯源树,研究论文发展脉络
Chat Paper
正在生成论文摘要