Multivariate Density Estimation by Neural Networks
IEEE TRANSACTIONS ON NEURAL NETWORKS AND LEARNING SYSTEMS(2024)
摘要
We propose nonparametric methods to obtain the Probability Density Function (PDF) to assess the properties of the underlying data generating process (DGP) without imposing any assumptions on the DGP, using neural networks (NNs). The proposed NN has advantages compared to well-known parametric and nonparametric density estimators. Our approach builds on literature on cumulative distribution function (CDF) estimation using NN. We extend this literature by providing analytical derivatives of this obtained CDF. Our approach hence removes the numerical approximation error in differentiating the CDF output, leading to more accurate PDF estimates. The proposed solution applies to any NN model, i.e., for any number of hidden layers or hidden neurons in the multilayer perceptron (MLP) structure. The proposed solution applies the PDF estimation by NN to continuous distributions as well as discrete distributions. We also show that the proposed solution to obtain the PDF leads to good approximations when applied to correlated variables in a multivariate setting. We test the performance of our method in a large Monte Carlo simulation using various complex distributions. Subsequently, we apply our method to estimate the density of the number of vehicle counts per minute measured with road sensors for a time window of 24 h.
更多查看译文
关键词
Artificial neural networks,Estimation,Probability density function,Neurons,Input variables,Global Positioning System,Bandwidth,Count data,Faa di Bruno (FDB) derivatives,Monte Carlo simulation,nonparametric density estimation,unsupervised learning
AI 理解论文
溯源树
样例
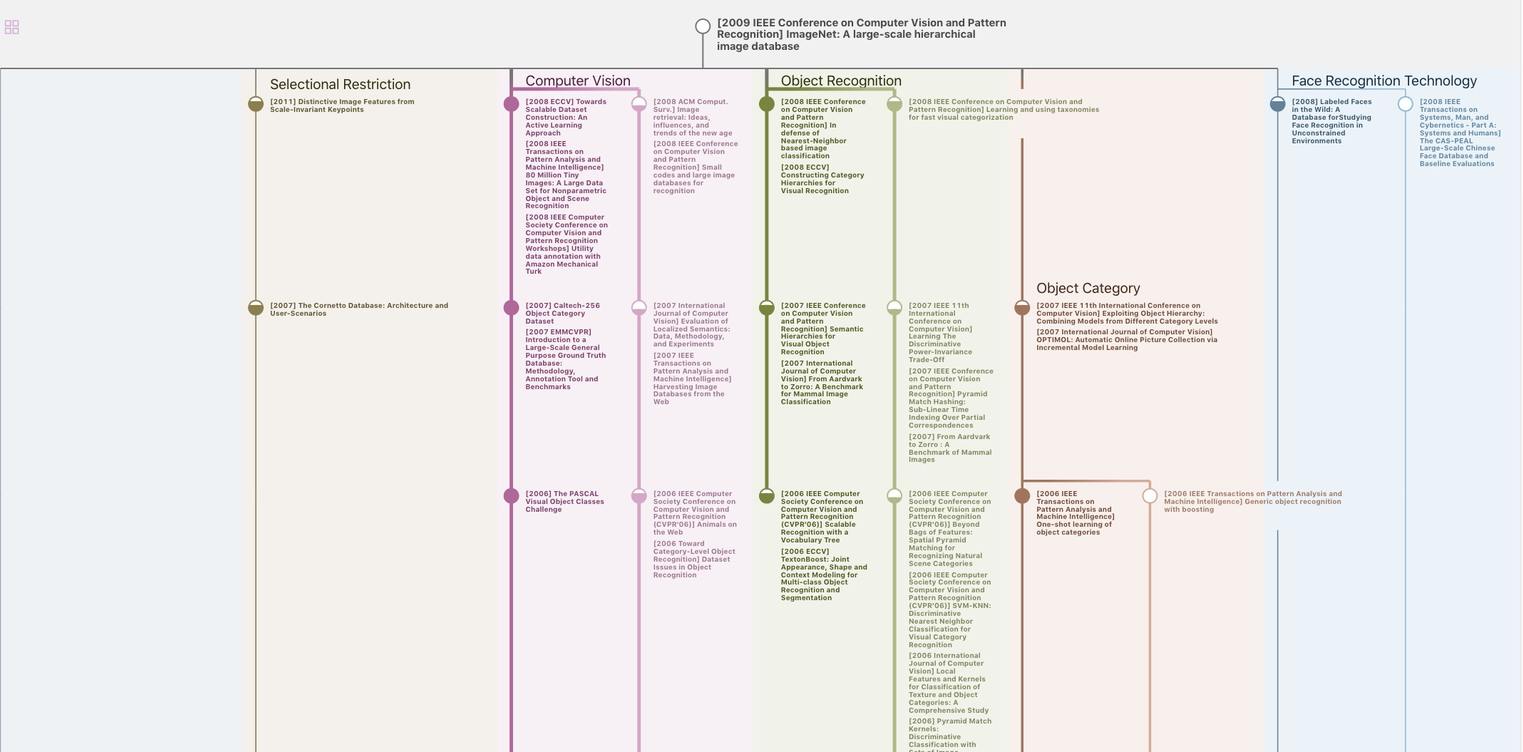
生成溯源树,研究论文发展脉络
Chat Paper
正在生成论文摘要