Network Pruning Towards Highly Efficient RRAM Accelerator
IEEE Transactions on Nanotechnology(2022)
摘要
RRAM crossbar arrays have contributed to the acceleration of deep neural networks (DNNs) by processing in-memory. How to make use of crossbar structure's properties to reduce the resource consumption while maintaining the reasonable accuracy and throughput is a critical issue for large scale sparse neural networks. In this paper, a pruning method for DNNs implemented on RRAM accelerator is proposed, which maps the 2D weight matrix to the crossbar arrays in blocks and reduces the row/column synapses of three sparse scenarios. To overcome the weights imbalance problem during the pruning process, we design an imbalance norm to regularize the network weights, leading to a more compact neural network after pruning. In addition, the corresponding hierarchical architecture of RRAM accelerator is proposed, which utilizes the index module to restore the orderly connection between the input voltage, output current and the cross array after network pruning. Experimental results demonstrated that the proposed pruning method could not only save 45.93% of crossbar resources, reduce the 81.26% power consumption, achieve 73.68% area saving, and speed up by 3.2x, with acceptable loss of accuracy, but also support neural network acceleration with limited precision weights (signed 5-bits).
更多查看译文
关键词
Crossbar architecture,neural network,pruning method,RRAM,sparsity
AI 理解论文
溯源树
样例
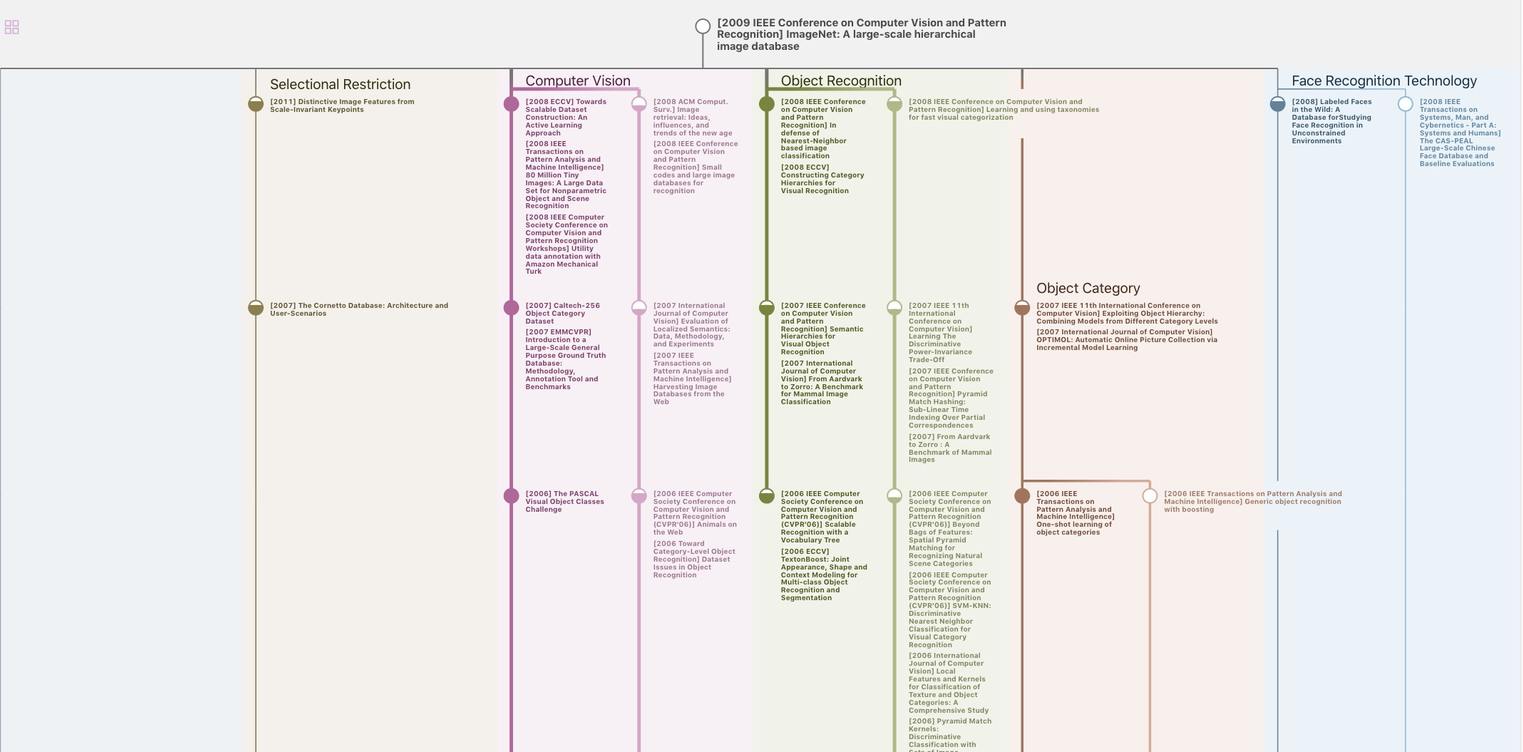
生成溯源树,研究论文发展脉络
Chat Paper
正在生成论文摘要