Forecasting Water Temperature in Cascade Reservoir Operation-Influenced River with Machine Learning Models
WATER(2022)
摘要
Water temperature (WT) is a critical control for various physical and biochemical processes in riverine systems. Although the prediction of river water temperature has been the subject of extensive research, very few studies have examined the relative importance of elements affecting WT and how to accurately estimate WT under the effects of cascaded dams. In this study, a series of potential influencing variables, such as air temperature, dew temperature, river discharge, day of year, wind speed and precipitation, were used to forecast daily river water temperature downstream of cascaded dams. First, the permutation importance of the influencing variables was ranked in six different machine learning models, including decision tree (DT), random forest (RF), gradient boosting (GB), adaptive boosting (AB), support vector regression (SVR) and multilayer perceptron neural network (MLPNN) models. The results showed that day of year (DOY) plays the most important role in each model for the prediction of WT, followed by flow and temperature, which are two commonly important factors in unregulated rivers. Then, combinations of the three most important inputs were used to develop the most parsimonious model based on the six machine learning models, where their performance was compared according to statistical metrics. The results demonstrated that GB3 and RF3 gave the most accurate forecasts for the training dataset and the test dataset, respectively. Overall, the results showed that the machine learning model could be effectively applied to predict river water temperature under the regulation of cascaded dams.
更多查看译文
关键词
water temperature prediction, machine learning, importance ranking, air temperature, flow discharge
AI 理解论文
溯源树
样例
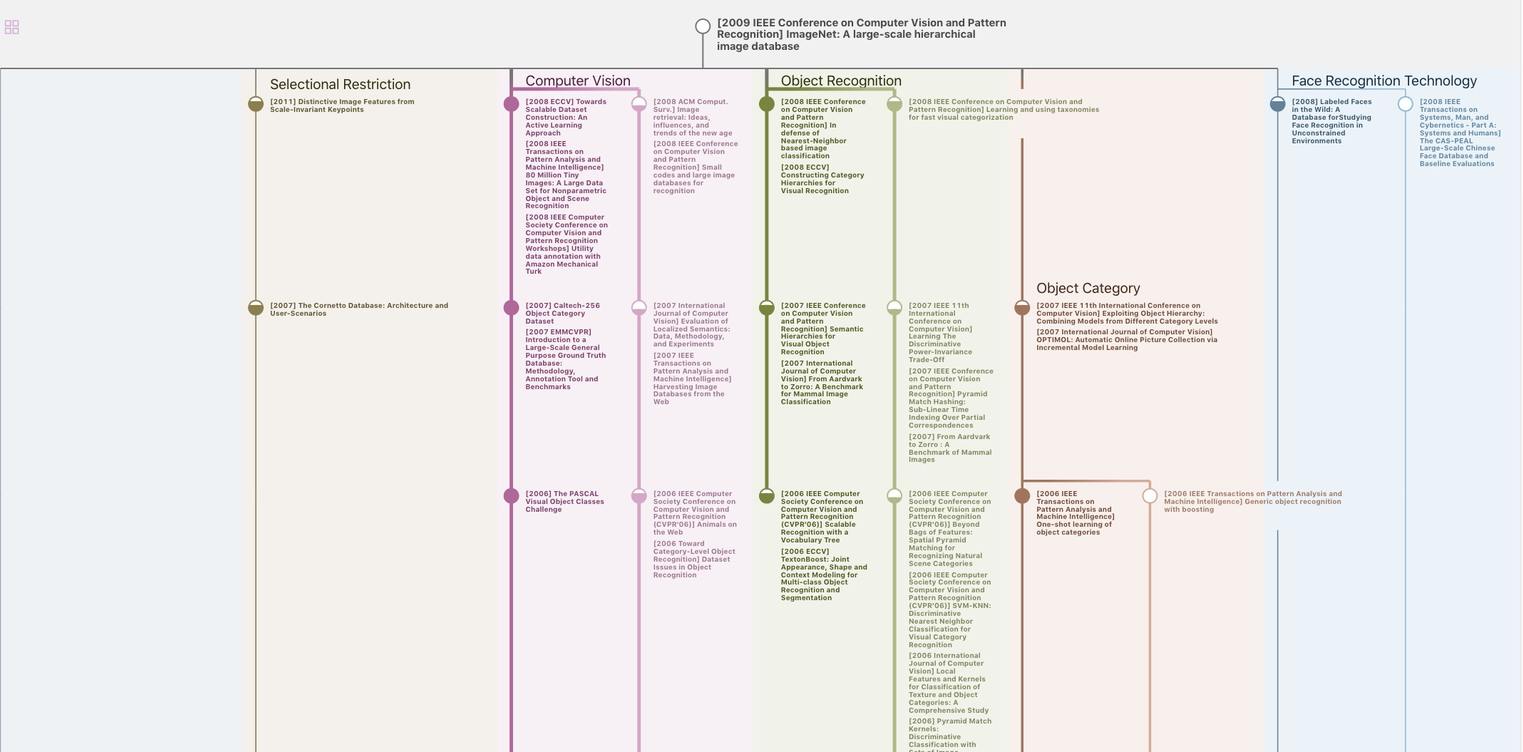
生成溯源树,研究论文发展脉络
Chat Paper
正在生成论文摘要