ISBFK-means: A new clustering algorithm based on influence space
Expert Systems with Applications(2022)
摘要
The time overhead is huge and the clustering quality is unstable when running the K-means algorithm on massive raw data. To solve these problems, the concept of the influence space is introduced, and on this basis, a new clustering algorithm named ISBFK-means based on the influence space is proposed in this paper. First, the influence space divides the given data set into multiple small regions. Then, the representative data objects in each region are obtained to form a new data set, in which the class labels of representative data objects are those of all the data objects in the correlation influence space. Next, the K-means clustering is performed on the new data set, thereby obtaining the final clustering result. Theoretical analysis and experimental results show that this approach effectively reduces the amount of data in the clustering process and improves the stability of clustering quality. As a major feature of this work, the celestial spectral data observed by the LAMOST survey are especially employed to verify the algorithm ISBFK-means. The experimental results indicate that this algorithm has higher performance than other similar algorithms on the correctness, efficiency and sensitivity to the quality of spectral data.
更多查看译文
关键词
Clustering,Influence space,Region partition,Representative data objects
AI 理解论文
溯源树
样例
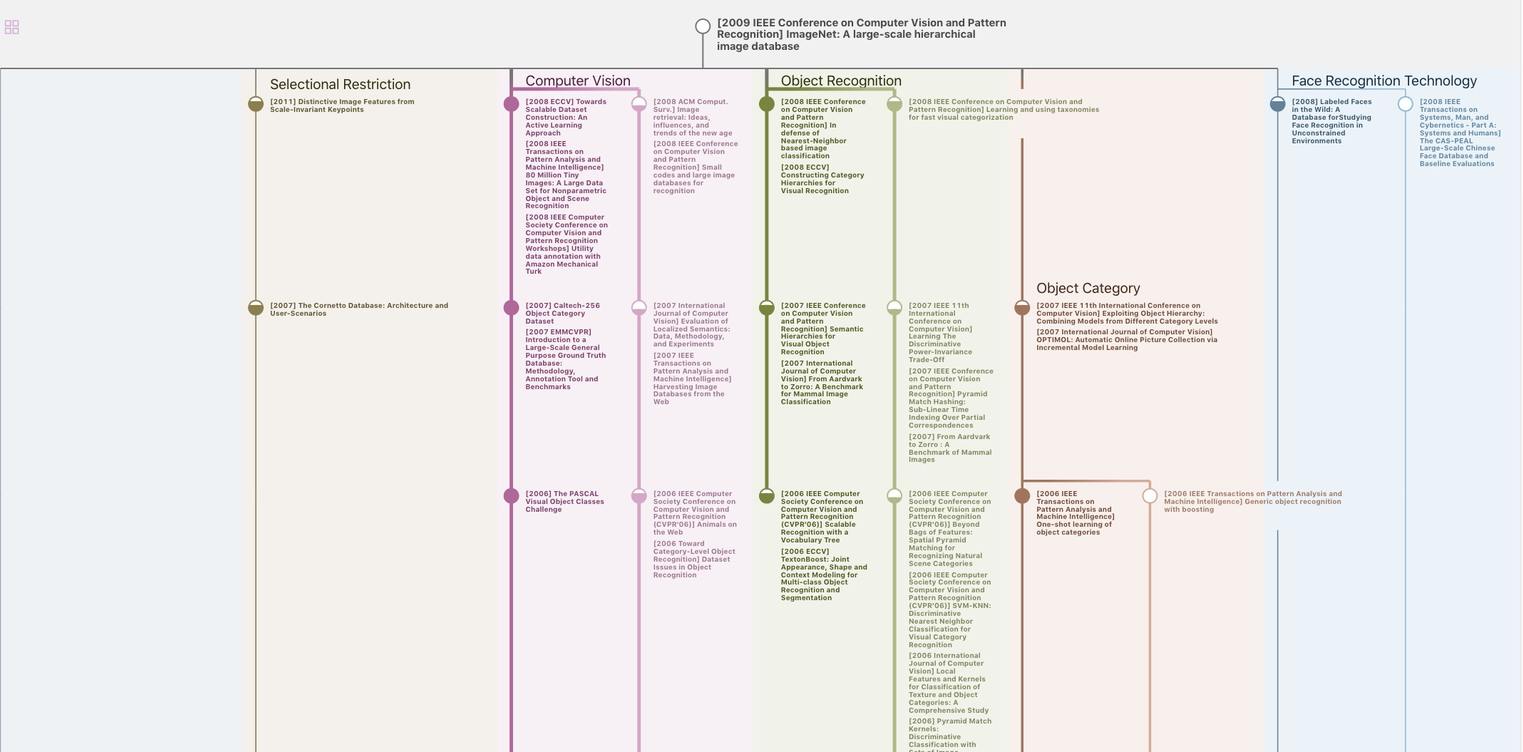
生成溯源树,研究论文发展脉络
Chat Paper
正在生成论文摘要