An enhanced Gray Wolf Optimization for cluster head selection in wireless sensor networks
INTERNATIONAL JOURNAL OF COMMUNICATION SYSTEMS(2022)
摘要
The energy efficiency sustenance in wireless sensor networks completely depends on the process of clustering to prolong network lifetime. The success of this clustering process completely depends on the cluster head (CH) selection process as potential CH selection prevents frequent clustering and aids in maintaining maximized energy in the network. In this paper, an improved Game Theory-Gray Wolf Optimization algorithm (GAGWO)-based efficient CH selection strategy is improved for maintaining the energy in the network through the construction of equal size clusters. This GWOA mimicked the hunting and attacking characteristics of gray wolves and facilitated better CH selection, which completely prevented worst sensor nodes from being selected as CH. It is proposed for establishing a better trade-off between the local and global search for the objective of preventing re-clustering that unnecessarily drains network energy. The simulation results of the proposed GAGWO confirmed better performance in terms of network lifetime, energy stability, mean throughput, and packet delivery ratio, on an average by 23.18%, 21.86%, 19.84%, and 20.98%, compared to the baseline CH approaches used for investigation.
更多查看译文
关键词
energy efficiency,game theory,Gray Wolf Optimization,meta-heuristic optimization,sensor node
AI 理解论文
溯源树
样例
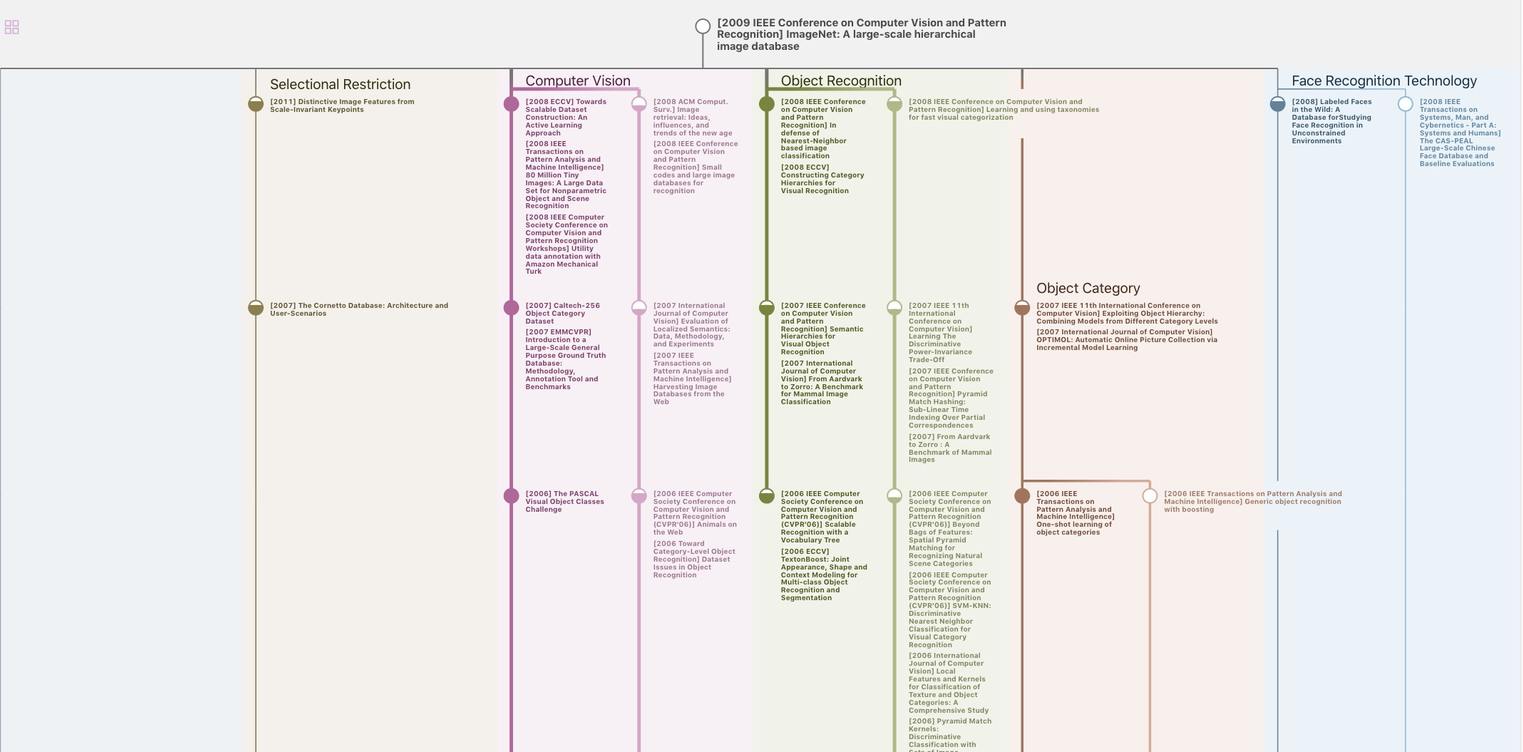
生成溯源树,研究论文发展脉络
Chat Paper
正在生成论文摘要