Machine Learning Enabled 3D Body Measurement Estimation Using Hybrid Feature Selection and Bayesian Search
APPLIED SCIENCES-BASEL(2022)
摘要
The 3D body scan technology has recently innovated the way of measuring human bodies and generated a large volume of body measurements. However, one inherent issue that plagues the use of the resultant database is the missing data usually caused by using automatic data extractions from the 3D body scans. Tedious extra efforts have to be made to manually fill the missing data for various applications. To tackle this problem, this paper proposes a machine learning (ML)-based approach for 3D body measurement estimation while considering the measurement (feature) importance. The proposed approach selects the most critical features to reduce the algorithm input and to improve the ML method performance. In addition, a Bayesian search is further used in fine-tuning the hyperparameters to minimize the mean square error. Two distinct ML methods, i.e., Random Forest and XGBoost, are used and tested on a real-world dataset that contains 3D body scans of 212 participants in the Kansas-Missouri area of the United States. The results show the effectiveness of the proposed methods with roughly 3% of Mean Absolute Percentage Errors in estimating the missing data. The two ML methods with the proposed hybrid feature selection and the Baysian search are comprehensively compared. The comparative results suggest that the Random Forest method performs better than the XGBoost counterpart in filling missing 3D body measurements.
更多查看译文
关键词
3D body measurement, machine learning, feature selection, bayesian optimization
AI 理解论文
溯源树
样例
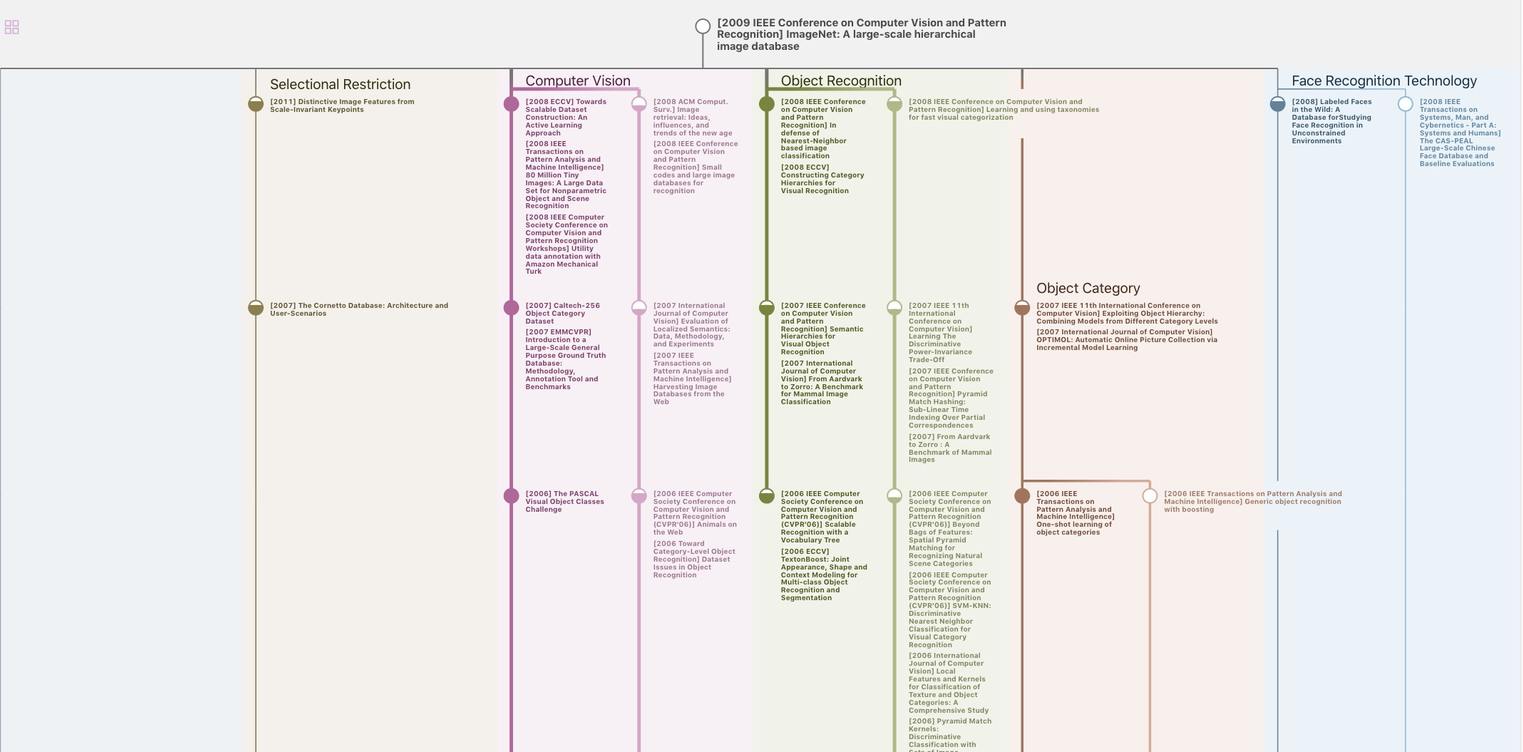
生成溯源树,研究论文发展脉络
Chat Paper
正在生成论文摘要