Climate-informed models benefit hindcasting but present challenges when forecasting species-habitat associations
ECOGRAPHY(2022)
摘要
Although species distribution models (SDMs) are commonly used to hindcast fine-scale population metrics, there remains a paucity of information about how well these models predict future responses to climate. Many conventional SDMs rely on spatially-explicit but time-invariant conditions to quantify species distributions and densities. We compared these status quo 'static' models with more climate-informed 'dynamic' SDMs to assess whether the addition of time-varying processes would improve hindcast performance and/or forecast skill. Here, we present two groundfish case studies from the Bering Sea - a high latitude system that has recently undergone considerable warming. We relied on conventional statistics (R-2, % deviance explained, UBRE or GCV) to evaluate hindcast performance for presence-absence, numerical abundance and biomass of arrowtooth flounder Atheresthes stomias and walleye pollock Gadus chalcogrammus. We then used retrospective skill testing to evaluate near-term forecast skill. Retrospective skill testing enables direct comparisons between forecasts and observations through a process of fitting and forecasting nested submodels within a given time series. We found that the inclusion of time-varying covariates improved hindcasts. However, dynamic models either did not improve or decreased forecast skill relative to static SDMs. This is likely a result of rapidly changing temperatures within the ecosystem, which required models to predict species responses to environmental conditions that were outside the range of observed values. Until additional model development allows for fully dynamic predictions, static model forecasts (or persistence forecasts from dynamic models) may serve as reliable placeholders, especially when anomalous conditions are anticipated. Nonetheless, our findings demonstrate support for the use of retrospective skill testing rather than selecting forecast models a priori based on their ability to quantify species-habitat associations in the past.
更多查看译文
关键词
climate change, forecast skill, generalized additive model, hindcast performance, retrospective skill testing, species distribution model
AI 理解论文
溯源树
样例
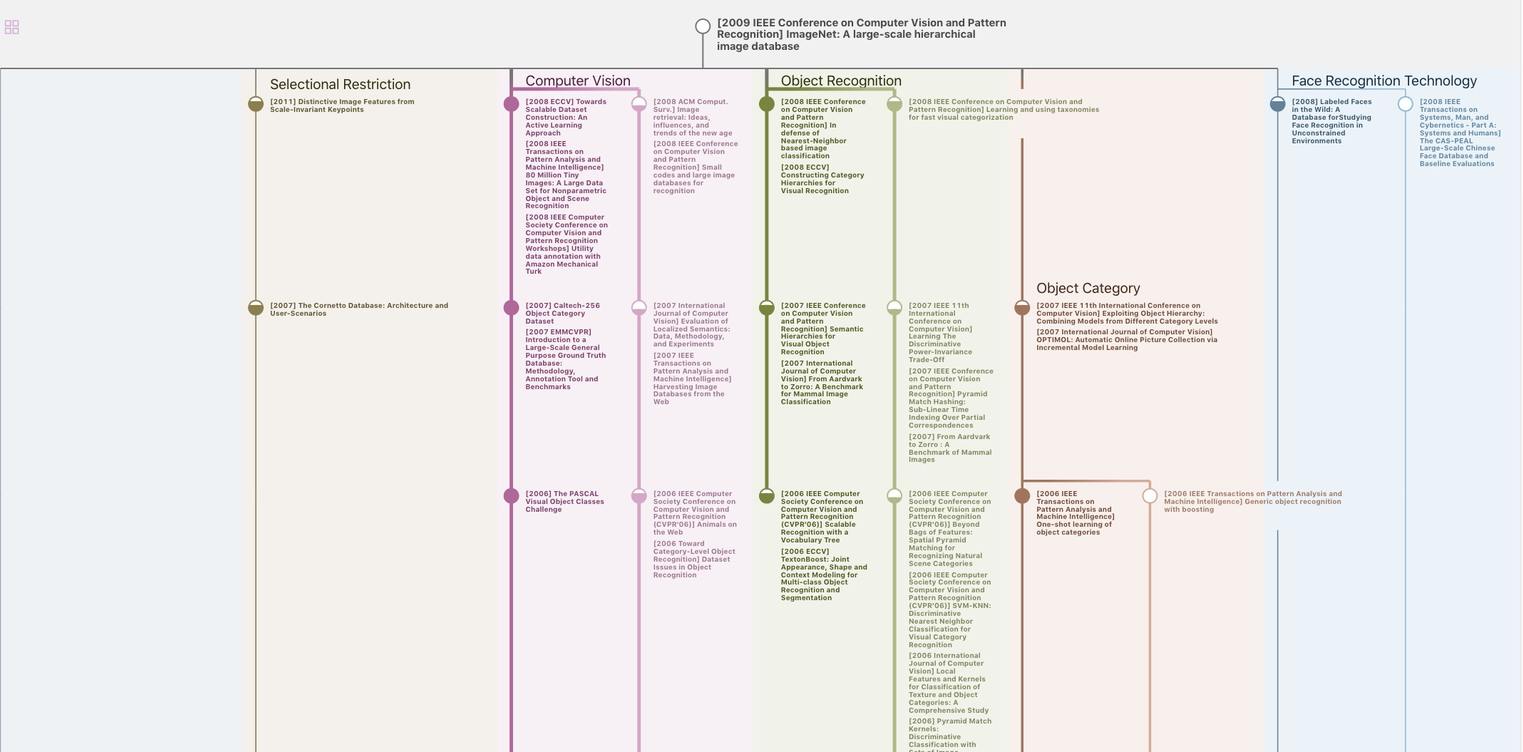
生成溯源树,研究论文发展脉络
Chat Paper
正在生成论文摘要