A Two-Stage Framework for Directed Hypergraph Link Prediction
MATHEMATICS(2022)
摘要
Hypergraphs, as a special type of graph, can be leveraged to better model relationships among multiple entities. In this article, we focus on the task of hyperlink prediction in directed hypergraphs, which finds a wide spectrum of applications in knowledge graphs, chem-informatics, bio-informatics, etc. Existing methods handling the task overlook the order constraints of the hyperlink's direction and fail to exploit features of all entities covered by a hyperlink. To make up for the deficiency, we present a performant pipelined model, i.e., a two-stage framework for directed hyperlink prediction method (TF-DHP), which equally considers the entity's contribution to the form of hyperlinks, and emphasizes not only the fixed order between two parts but also the randomness inside each part. The TF-DHP incorporates two tailored modules: a Tucker decomposition-based module for hyperlink prediction, and a BiLSTM-based module for direction inference. Extensive experiments on benchmarks-WikiPeople, JF17K, and ReVerb15K-demonstrate the effectiveness and universality of our TF-DHP model, leading to state-of-the-art performance.
更多查看译文
关键词
hyperlink prediction, hypergraph, Tucker decomposition
AI 理解论文
溯源树
样例
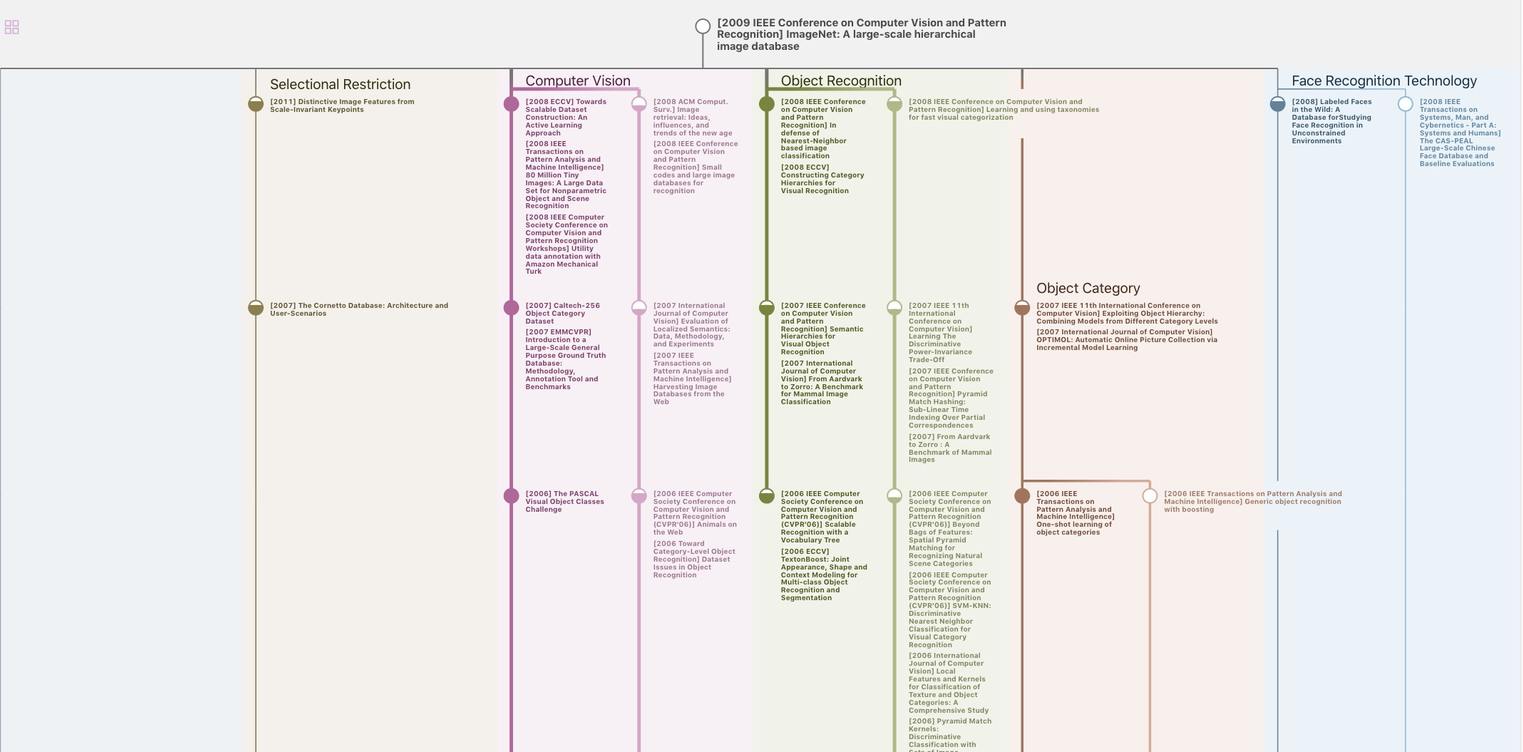
生成溯源树,研究论文发展脉络
Chat Paper
正在生成论文摘要