Handling occlusion in prohibited item detection from X-ray images
NEURAL COMPUTING & APPLICATIONS(2022)
摘要
Prohibited item detection from X-ray images determines whether any prohibited items are present in baggage, and great progress has recently been made in this field with the development of deep learning. Nevertheless, the appearance of an occluded item interacts with the cover, which is different from occlusions encountered in conventional object detection. We design three mechanisms to handle this challenge on the assumption that the occluded part is still partially observed. First, we propose a scale interaction module in which the features in neighboring scales interact one or more times to enhance the model’s perception ability. Then, we design a cross-image weakly supervised semantic analysis model utilizing the coattention mechanism to perceive similar and different targets, breaking through the information bottleneck of the isolated detection of a single image. Finally, we introduce a multitask learning module to simultaneously optimize the model at the global level and pixel level. We evaluate our approach on the publicly available security inspection X-ray (SIXray) dataset, the occluded prohibited items X-ray (OPIXray) dataset, and the HIXray dataset, and the results show that our approach is competitive with other X-ray baggage inspection approaches.
更多查看译文
关键词
Computer vision, Convolutional neural network, Information fusion, Weakly supervised learning
AI 理解论文
溯源树
样例
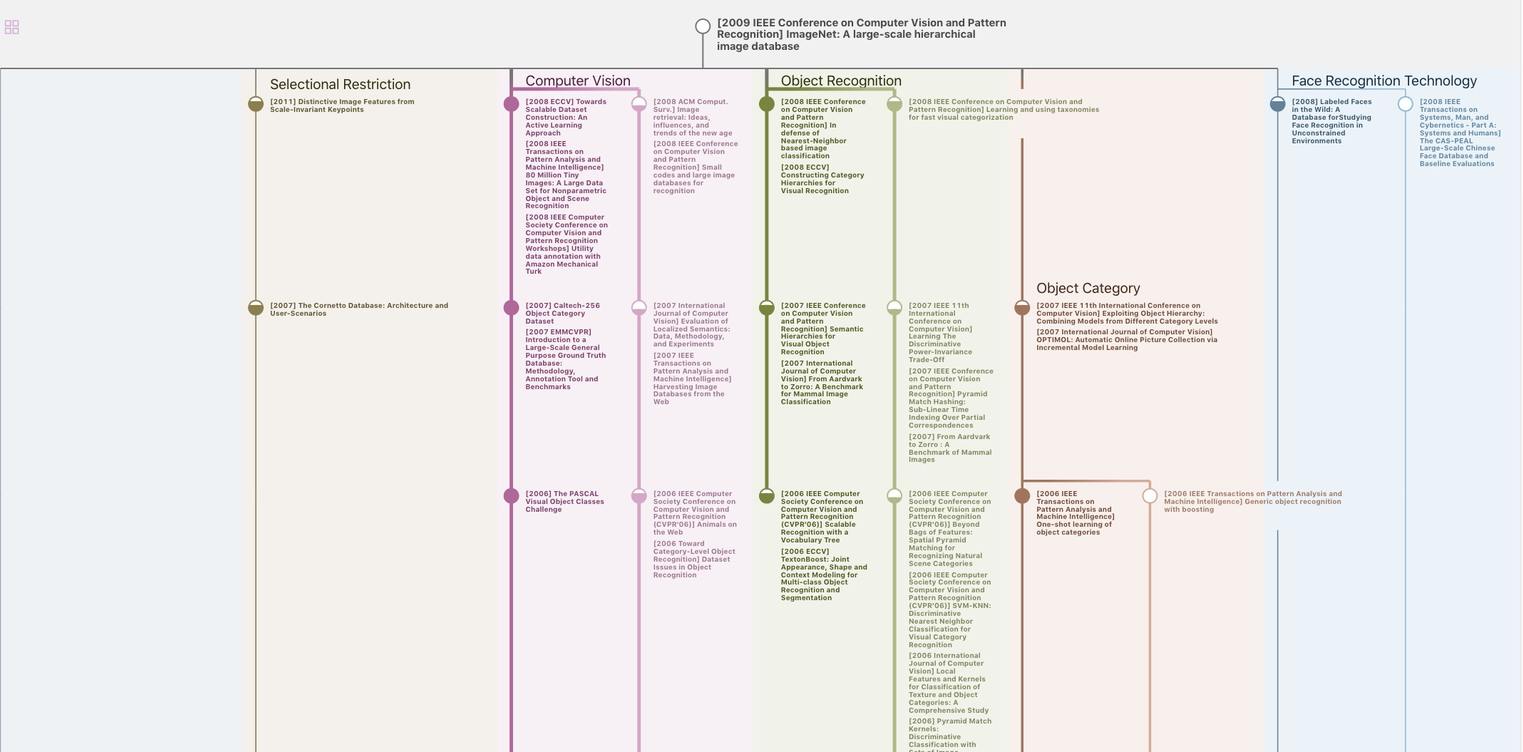
生成溯源树,研究论文发展脉络
Chat Paper
正在生成论文摘要