Nonparametric variable screening for multivariate additive models
Journal of Multivariate Analysis(2022)
摘要
In this paper we develop a novel procedure of variable screening for a multivariate additive random-effects model, based on B-spline function approximations. With these approximations, the so-called signal-to-noise ratio (SNR) can be defined to inform the importance of each covariate in the model. Then, SNR-based forward filtering is conducted on covariates by using iterative projections of the multiple response data into the space of covariates. The proposed procedure is easy to use and allows the user to pool non-linear information across heterogeneous subjects through random-effects variables. We establish an asymptotic theory on the selection consistency under some regularity conditions. By simulations, we show that the procedure has a superior performance over some existing methods in terms of sensitivity and specificity. We also apply the procedure to anti-cancer drug data, revealing a set of biomarkers that potentially influence concentrations of anti-cancer drugs in cancer cell lines.
更多查看译文
关键词
primary,secondary
AI 理解论文
溯源树
样例
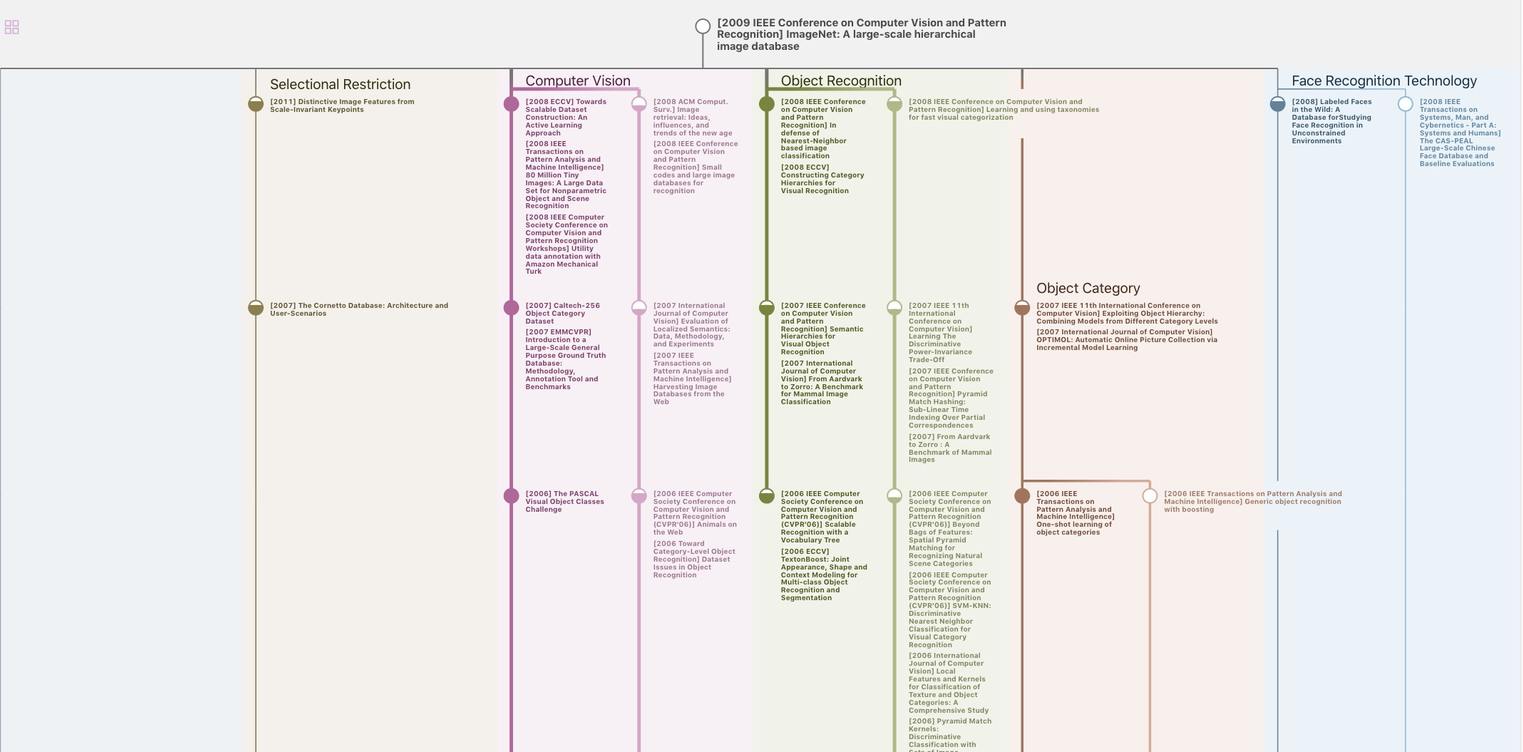
生成溯源树,研究论文发展脉络
Chat Paper
正在生成论文摘要