A novel hybrid model integrating modified ensemble empirical mode decomposition and LSTM neural network for multi-step precious metal prices prediction
Resources Policy(2022)
摘要
Accurately predicting precious metal prices is of extreme significance as they possess an essential position in both financial and industrial fields. To achieve higher prediction accuracy, in this paper, a modified ensemble empirical mode decomposition (MEEMD) method integrated with long short-term memory neural network (LSTM) is used for precious metal price prediction. The multi-scale permutation entropy (MPE) analysis demonstrated that MEEMD has better decomposition effect than ensemble empirical mode decomposition (EEMD). Then input each intrinsic mode function (IMF) obtained by MEEMD into LSTM for prediction. Finally add each IMF forecasting value to get the final prediction results. Compared with traditional multilayer perceptron neural network (MLP), support vector regression (SVR) and a combination forecasting model super learner (SL), MEEMD-LSTM improves the prediction performance in one-step ahead prediction and multi-step ahead prediction. The multi-horizon model confidence set (MCS) test are adopted to comprehensively and statistically testify the best prediction performance of MEEMD-LSTM. Furthermore, this research indicates that the model still shows better prediction accuracy either under different proportions of the training set to the test set or in different periods of business cycle. This competitive precious metal price forecasting model is a promising technique for government agencies, investors and related enterprises.
更多查看译文
关键词
Precious metal prices,Modified ensemble empirical mode decomposition,Long short-term memory,Multi-step ahead forecasting,Multi-horizon model confidence set,Business expansion periods and recession periods
AI 理解论文
溯源树
样例
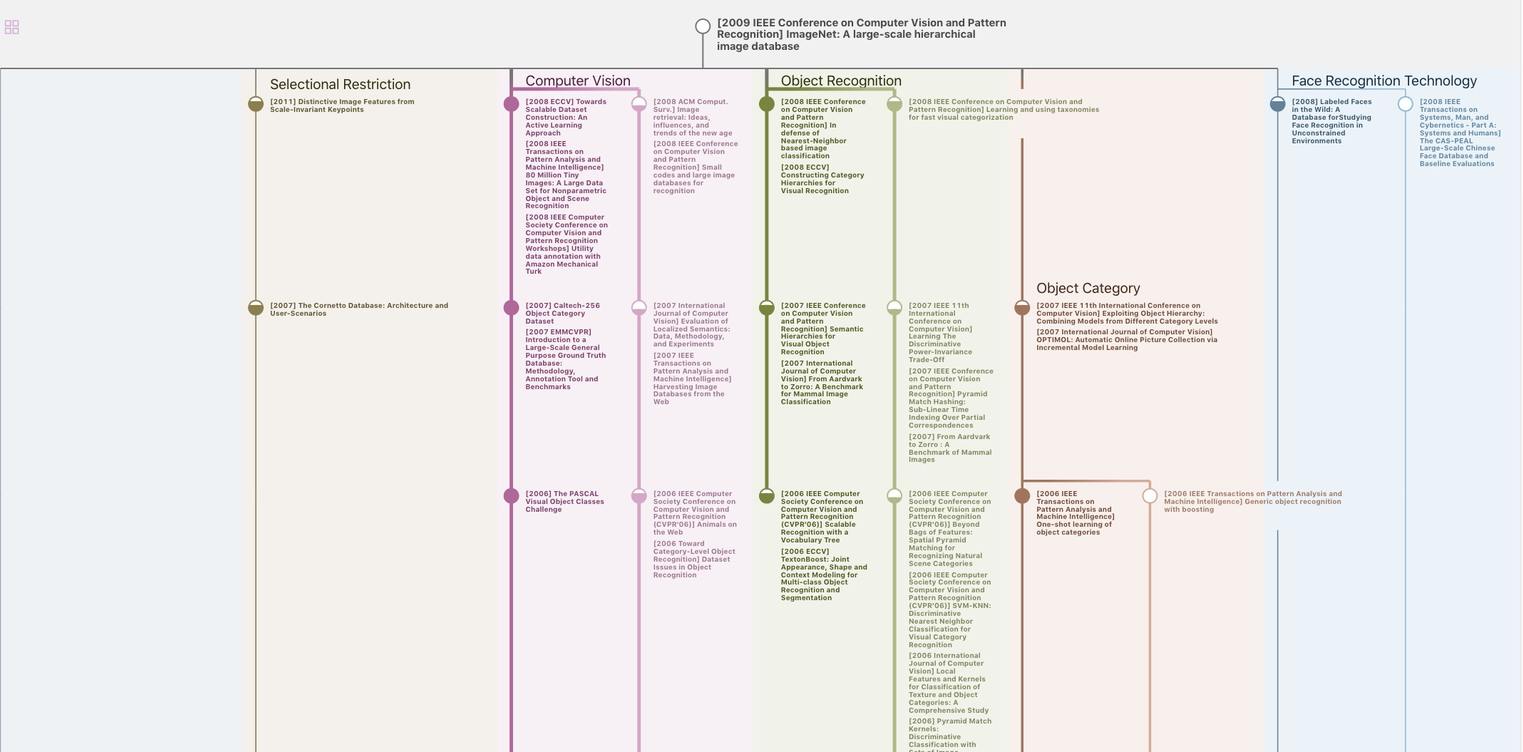
生成溯源树,研究论文发展脉络
Chat Paper
正在生成论文摘要