A denoising and multiscale residual deep network for soft sensor modeling of industrial processes
MEASUREMENT SCIENCE AND TECHNOLOGY(2022)
摘要
Deep learning plays an important role in soft sensors of industrial processes for the timely measurement of key quality variables. However, since sensors are often operated under noisy and nonstationary industrial conditions, the collected industrial process data exhibit extreme complexity, which severely restricts the learning capacity and measurement accuracy of deep learning methods. In this paper, a novel denoising and multiscale residual deep network (DMRDN) is proposed for soft sensor modeling. Firstly, a stacked denoising autoencoder with level-aware attention is developed to denoise the process data, in which denoised features on different levels are learned and fused. Secondly, the denoised features are fed into multiscale residual convolutional neural network with scale-aware attention, which is designed to capture and fuse deep dynamic features from different scales. Finally, experiments were conducted on an industrial debutanizer column. The experimental results demonstrate that the proposed DMRDN greatly strengthens the learning ability and achieves better prediction performance compared with other methods.
更多查看译文
关键词
soft sensor, deep learning, stacked denoising autoencoder, convolutional neural network, denoising and multiscale residual deep network
AI 理解论文
溯源树
样例
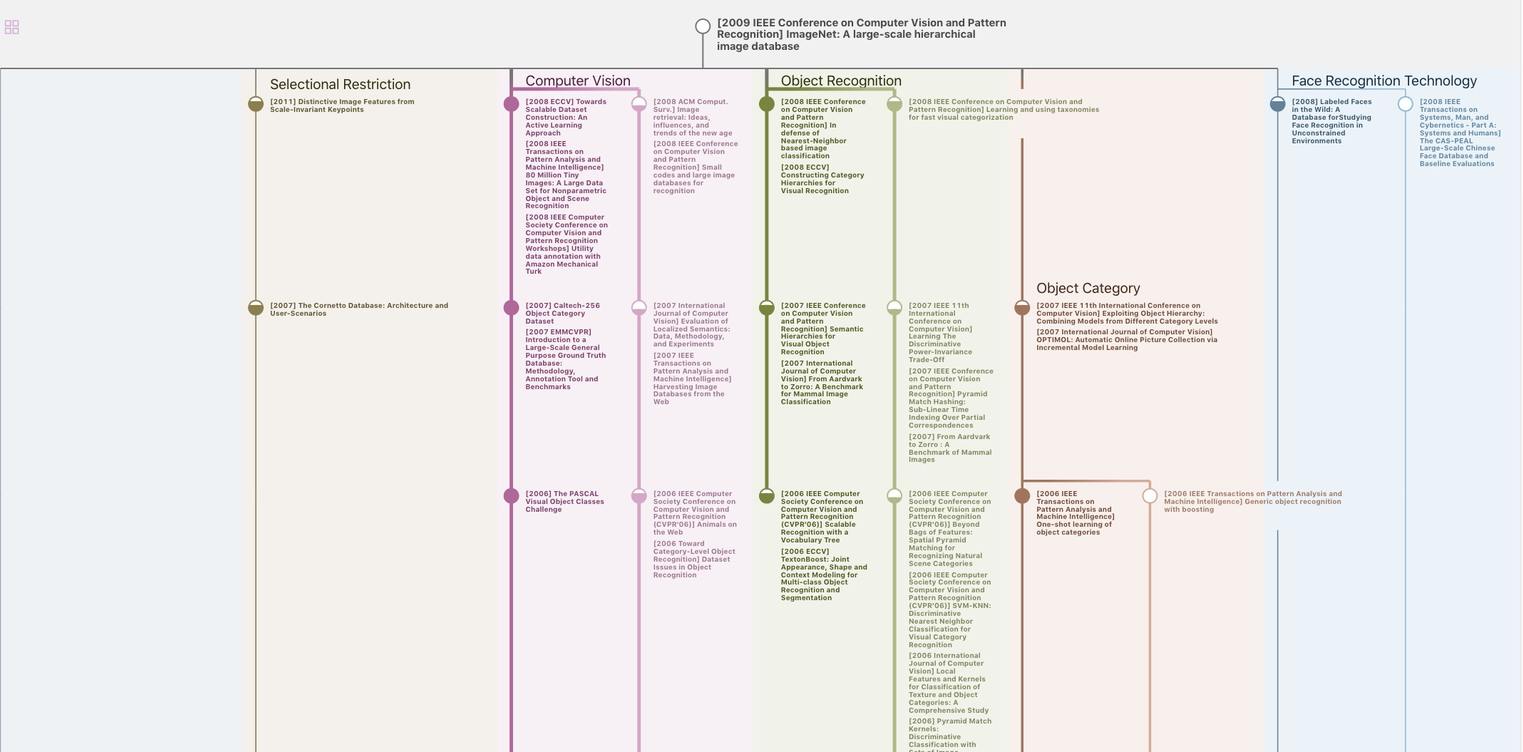
生成溯源树,研究论文发展脉络
Chat Paper
正在生成论文摘要