Characterization of portuguese sown rainfed grasslands using remote sensing and machine learning
Precision Agriculture(2022)
摘要
Grasslands are crucial ecosystems that support and provide a diverse number of ecosystem services. Sown biodiverse pastures rich in legumes (SBP) were developed with the main goal of increasing grassland production while minimizing fertilizers inputs. In this paper, the main properties of SBP in Portugal were estimated using remote sensing and machine learning in six different farms and two production years (spring 2018 and 2019). Four pasture characteristics were considered: aboveground standing biomass, fraction of legumes, plant nitrogen (N) content and plant phosphorus (P) content. Remote sensing data were obtained from Sentinel-2. The spectral bands combined with 5 vegetation indices and 9 covariates were used. Multiple linear regression, LASSO, Ridge, random forests, XGBoost and LightGBM regression models were used. Two cross-validation approaches were used: (1) a random approach with random selection of the folds (RN-CV), and (2) a structured approach where each fold is a unique combination of farm and year, which is subsequently used to assess the performance of the model obtained with the 8 other folds (LLYO-CV). Results showed that the random forest method had the best estimation accuracy for all pasture characteristics. Regarding cross-validation approaches, the algorithms with RN-CV have higher estimation accuracy for all pasture characteristics (on average about 10% lower RMSE and an R 2 85% higher), as compared to the algorithms with LLYO-CV. However, LLYO-CV should avoid overfitting and improve generalization of the models because in each fold the model is tested in a farm and year that was not used for training. The RMSE for all variables were significantly low, especially in RN-CV. Plant P is the variable where the choice of CV approach has the least influence (RMSE of test set with RN-CV: 0.71 g P kg − 1 ; LLYO-CV: 0.72 g P kg − 1 ). Standing biomass is the variable with the highest difference between CV approaches (RN-CV: 722 kg ha − 1 ; LLYO-CV: 825 kg ha − 1 ). The RMSE, of legumes and plant N were moderately affected by the CV approach (legume RN-CV: 0.11; LLYO-CV: 0.12 – plant N RN-CV: 3.96 g N kg − 1 ; LLYO-CV: 3.99 g N kg − 1 ). The algorithms developed here were applied for entire parcels in the two farms with the most different climate conditions as demonstration of their potential future use for precision farming.
更多查看译文
关键词
Sentinel-2,Multiple linear regression,LASSO,Ridge,XGBoost,LightGBM,Random forests,Cross-validation
AI 理解论文
溯源树
样例
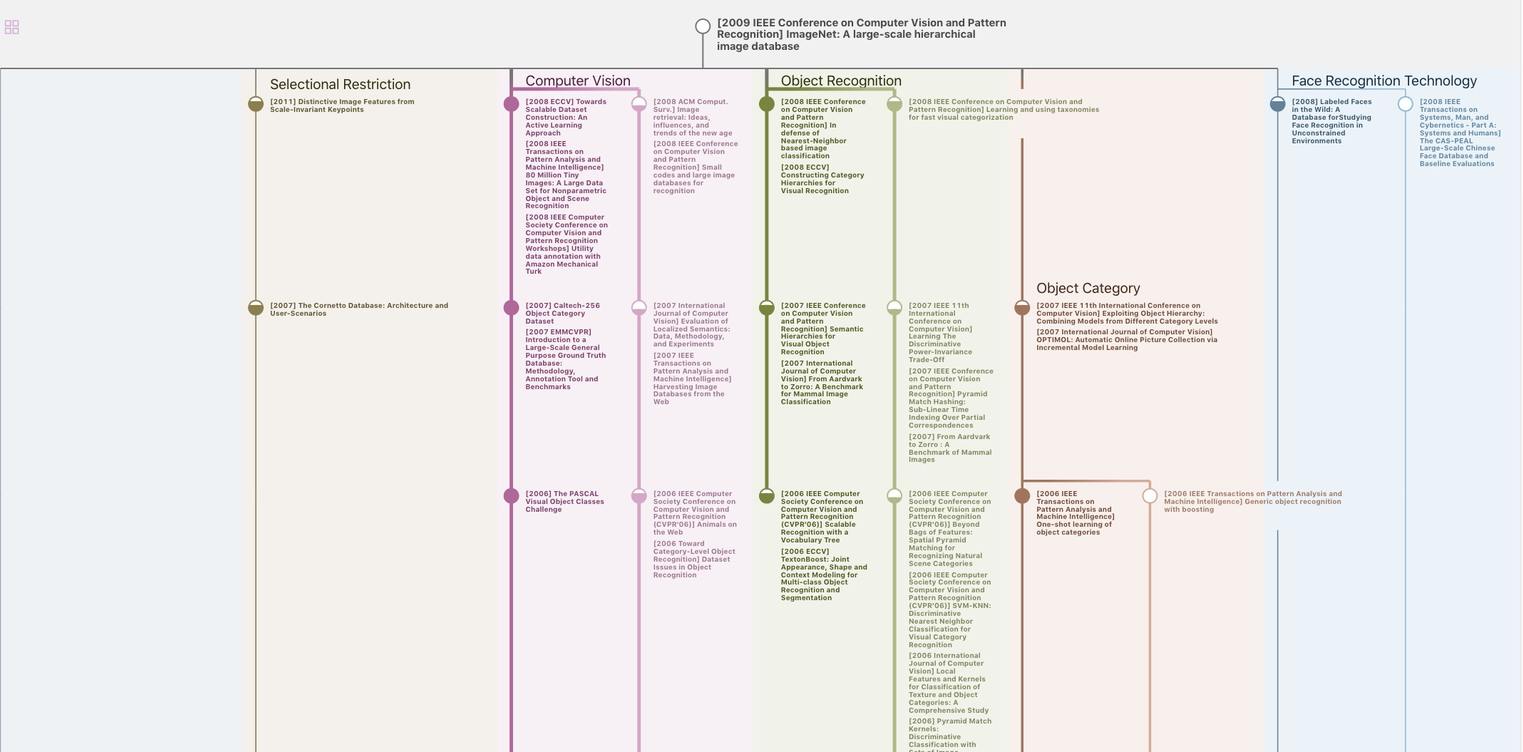
生成溯源树,研究论文发展脉络
Chat Paper
正在生成论文摘要