Resource-Aware Edge-Based Stream Analytics
IEEE Internet Computing(2022)
摘要
Understanding how machine learning (ML) algorithms can be used for stream processing on edge devices remains an important challenge. Such ML algorithms can be represented as operators and dynamically adapted based on the resources on which they are hosted. Deploying ML algorithms on edge resources often focuses on carrying out inference on the edge, while learning and model development takes place on a cloud data center. In this article, we describe TinyMOA, a modified version of the open-source massive online analytics library for stream processing, that can be deployed across both local and remote edge resources using the Parsl and Kafka systems. Using an experimental testbed, we demonstrate how ML stream-processing operators can be configured based on the resource on which they are hosted, and discuss subsequent implications for edge-based stream-processing systems.
更多查看译文
关键词
edge computing,MOA,sensor data processing,stream processing,machine learning
AI 理解论文
溯源树
样例
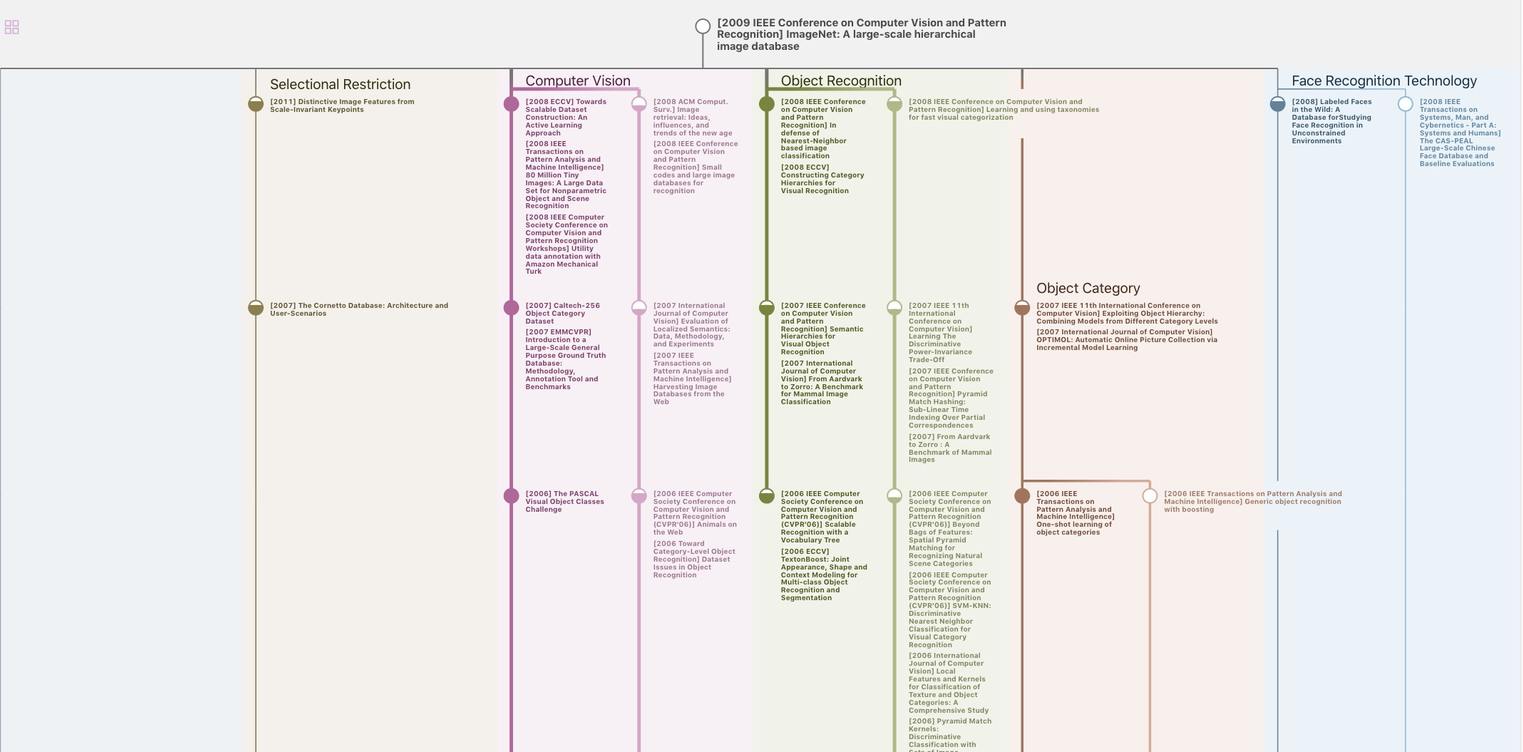
生成溯源树,研究论文发展脉络
Chat Paper
正在生成论文摘要