A systematic approach to diagnosis and categorization of bone fractures in X-Ray imagery
INTERNATIONAL JOURNAL OF HEALTHCARE MANAGEMENT(2022)
摘要
Researchers have proposed several ways of diagnosing, classifying and categorizing bone fractures. Nevertheless, no standardized classification has yet been established for all identified fractures. In recent times Machine learning and deep learning are becoming more popular. Deep Neural Networks (DNN) are well-known models for their image classification capabilities and capacity to tackle complex problems. To categorize (normal, comminute, oblique, spiral, greenstick, impacted, and transverse) and recognize fragmented images, the -Speeded Up Robust Features (SURF) and Scale Invariant Feature Transform (SIFT) feature extraction approaches were utilized for several algorithms: K-Nearest Neighbor (KNN), Support Vector Machine (SVM), Random Forest (RF), Inception V3 and ResNeXt101. This research aims to construct an image processing system, including information from X-ray and Computer Tomography (CT) scans, classifying bone fractures rapidly and precisely. Preprocessing, quality enhancement, and extraction techniques are utilized to process X-ray images of the shattered bone collected from the hospital. The images are then separated and classified into fractured and unfractured bones and are compared with the accuracy of other methodologies like KNN, SVM, RF, InceptionV3, and ResNeXt101. According to the findings, the bone fracture detection and classification system perform well-using ResNeXt101, with an accuracy rate of 93.75%.
更多查看译文
关键词
Convolutional neural network, deep learning, edge detection, bone fracture classification, image segmentation, machine learning
AI 理解论文
溯源树
样例
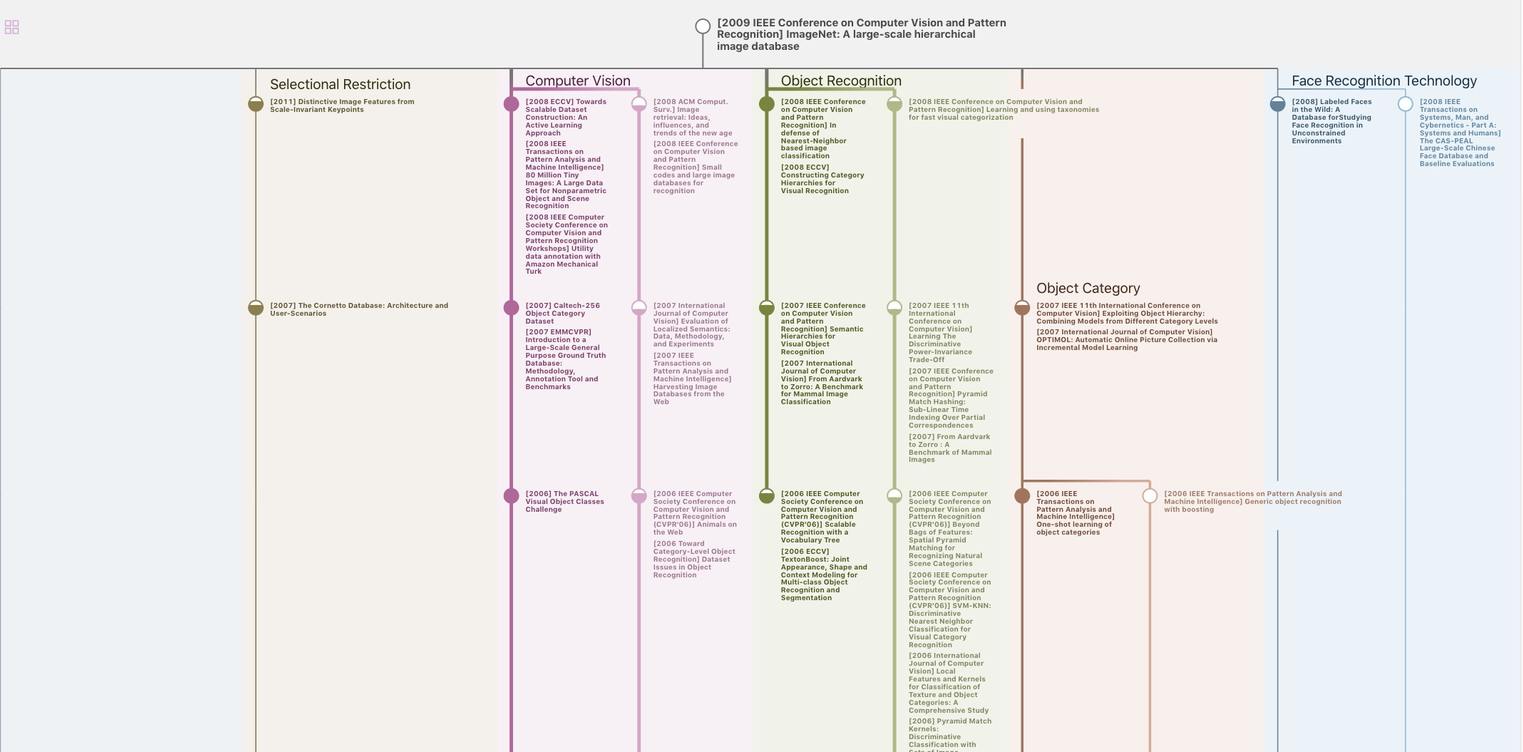
生成溯源树,研究论文发展脉络
Chat Paper
正在生成论文摘要