An Improved Dictionary-Based Method for Gas Identification with Electronic Nose
APPLIED SCIENCES-BASEL(2022)
摘要
The dictionary learning algorithm has been successfully applied to electronic noses because of its high recognition rate. However, most dictionary learning algorithms use l0-norm or l1-norm to regularize the sparse coefficients, which means that the electronic nose takes a long time to test samples and results in the inefficiency of the system. Aiming at accelerating the recognition speed of the electronic nose system, an efficient dictionary learning algorithm is proposed in this paper where the algorithm performs a multi-column atomic update. Meanwhile, to solve the problem that the singular value decomposition of the k-means (K-SVD) dictionary has little discriminative power, a novel classification model is proposed, a coefficient matrix is achieved by a linear projection to the training sample, and a constraint is imposed where the coefficients in the same category should keep a large coefficient and be closer to their class centers while coefficients in the different categories should keep sparsity. The algorithm was evaluated and analyzed based on the comparisons of several traditional classification algorithms. When the dimension of the sample was larger than 10, the average recognition rate of the algorithm was maintained above 92%, and the average training time was controlled within 4 s. The experimental results show that the improved algorithm is an effective method for the development of an electronic nose.
更多查看译文
关键词
sparse representation,dictionary learning,pattern recognition,electronic nose
AI 理解论文
溯源树
样例
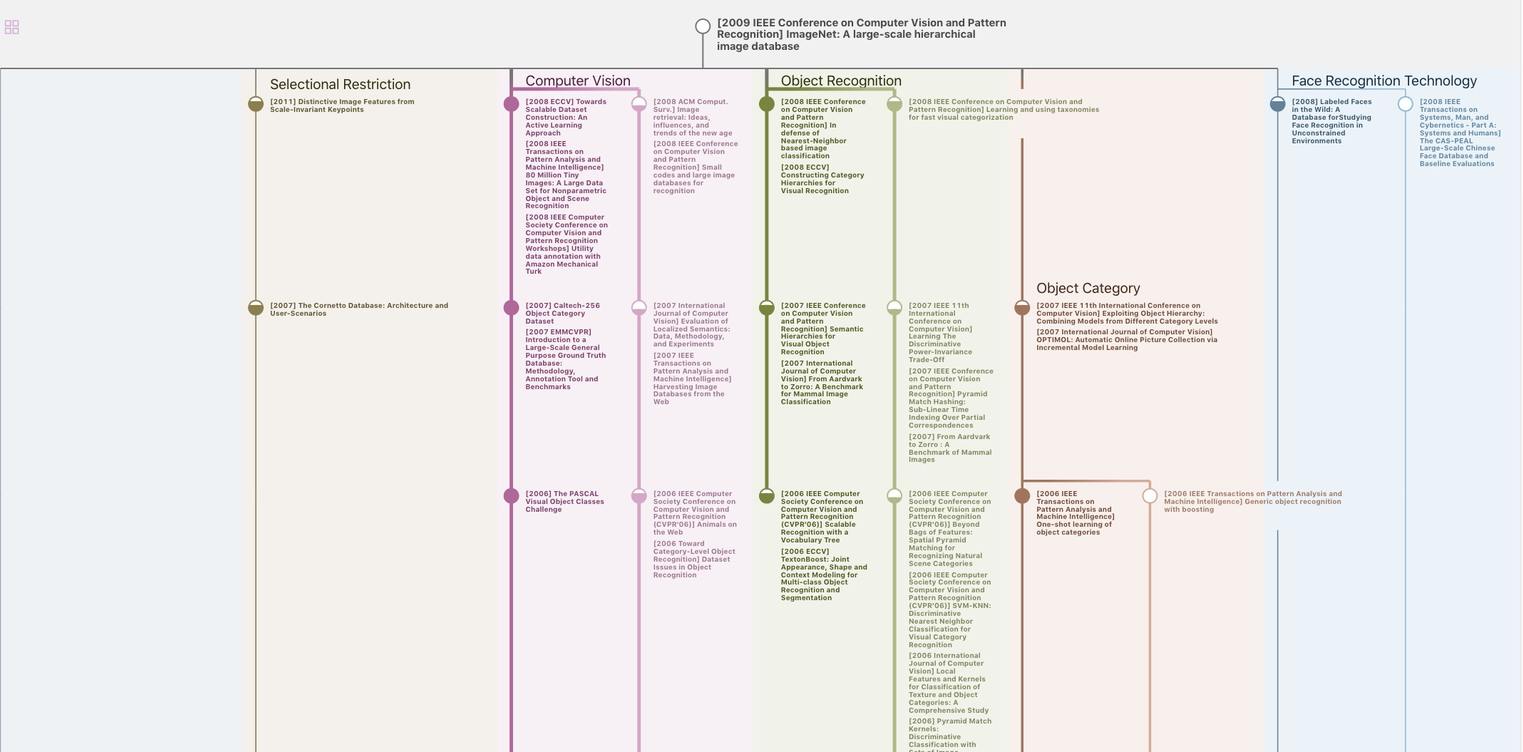
生成溯源树,研究论文发展脉络
Chat Paper
正在生成论文摘要