Tightly Coupled Multimodal Sensor Data Fusion for Robust State Observation With Online Delay Estimation and Compensation
IEEE SENSORS JOURNAL(2022)
Abstract
Ego-vehicle state estimation can be achieved by utilizing classic vehicle and environmental sensors. However, each type of sensor has specific strengths and limitations regarding accuracy and robustness. Using existing sensor concept cannot fulfill the requirements for both accuracy and robustness against changing vehicle parameters and environments while driving. Therefore, we propose a framework to exploit the advantages of each individual sensor type seeking high accuracy and robustness. The uncertainty of state variables is estimated by the fusion of an inertial measurement unit, a global navigation satellite system, a radar sensor, and a lidar sensor. The multimodal sensor data are processed in a distributed manner in each sensor model at a low level, and the physical quantities from the sensor models are fused centrally by a probabilistic method, namely, the synchronized error-state extended Kalman filter, at a high level. We propose an event-based approach to estimate sensor system delay and compensate lagged signals using forward prediction fusion. The concept was implemented in a test vehicle and evaluated in field tests for dynamic driving and on public roads. The algorithm represents real-time estimation with high accuracy for different driving maneuvers and robustness against different disturbances caused by the environment and changing chassis parameters.
MoreTranslated text
Key words
Sensors, Laser radar, Sensor fusion, State estimation, Intelligent sensors, Global navigation satellite system, Robustness, Intelligent vehicles, state estimation, sensor data fusion, Kalman filter, multimodal sensor data
AI Read Science
Must-Reading Tree
Example
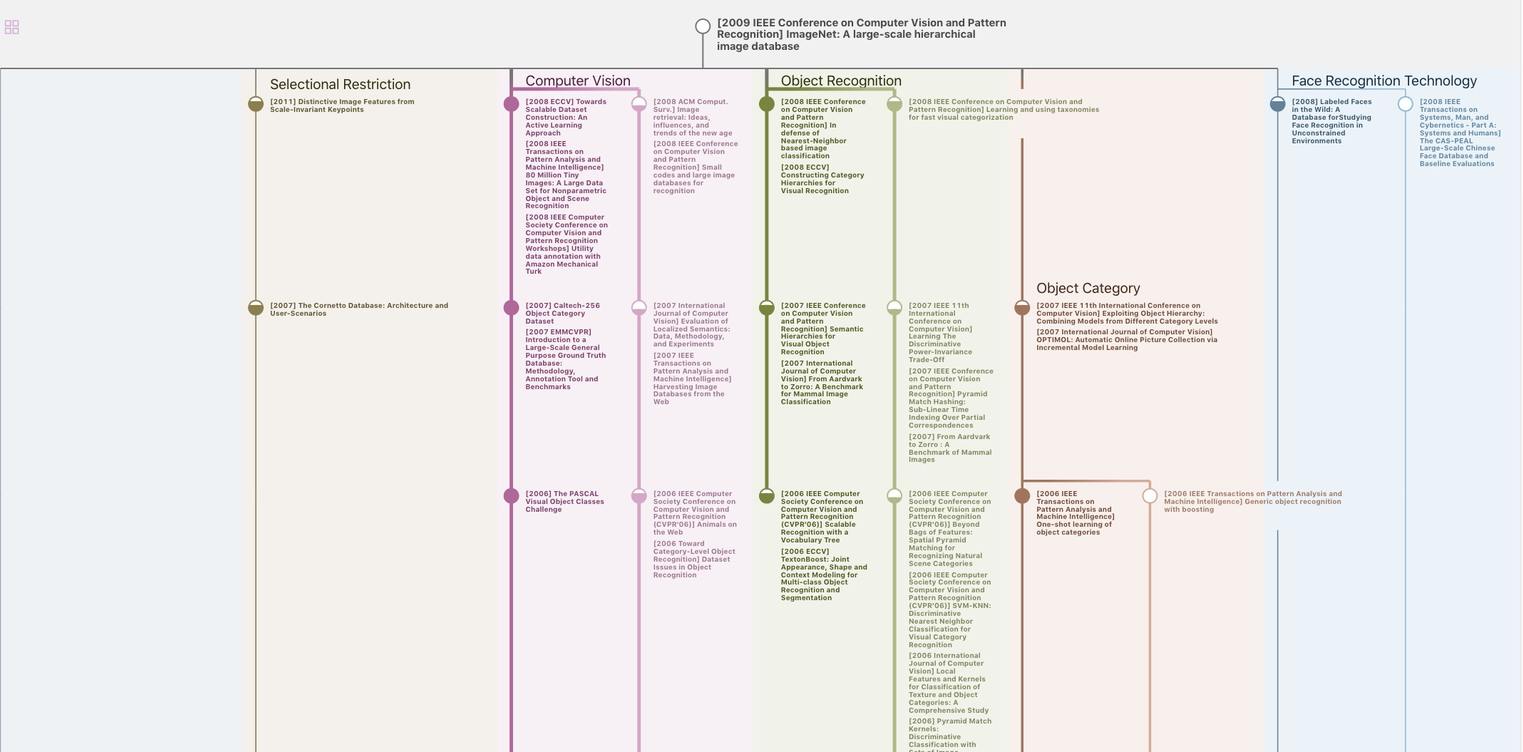
Generate MRT to find the research sequence of this paper
Chat Paper
Summary is being generated by the instructions you defined