A Bounded Archiver for Hausdorff Approximations of the Pareto Front for Multi-Objective Evolutionary Algorithms
MATHEMATICAL AND COMPUTATIONAL APPLICATIONS(2022)
摘要
Multi-objective evolutionary algorithms (MOEAs) have been successfully applied for the numerical treatment of multi-objective optimization problems (MOP) during the last three decades. One important task within MOEAs is the archiving (or selection) of the computed candidate solutions, since one can expect that an MOP has infinitely many solutions. We present and analyze in this work ArchiveUpdateHD, which is a bounded archiver that aims for Hausdorff approximations of the Pareto front. We show that the sequence of archives generated by ArchiveUpdateHD yields under certain (mild) assumptions with a probability of one after finitely many steps a Delta(+)-approximation of the Pareto front, where the value Delta(+) is computed by the archiver within the run of the algorithm without any prior knowledge of the Pareto front. The knowledge of this value is of great importance for the decision maker, since it is a measure for the "completeness" of the Pareto front approximation. Numerical results on several well-known academic test problems as well as the usage of ArchiveUpdateHD as an external archiver within three state-of-the-art MOEAs indicate the benefit of the novel strategy.
更多查看译文
关键词
evolutionary multi-objective optimization, archiving, convergence
AI 理解论文
溯源树
样例
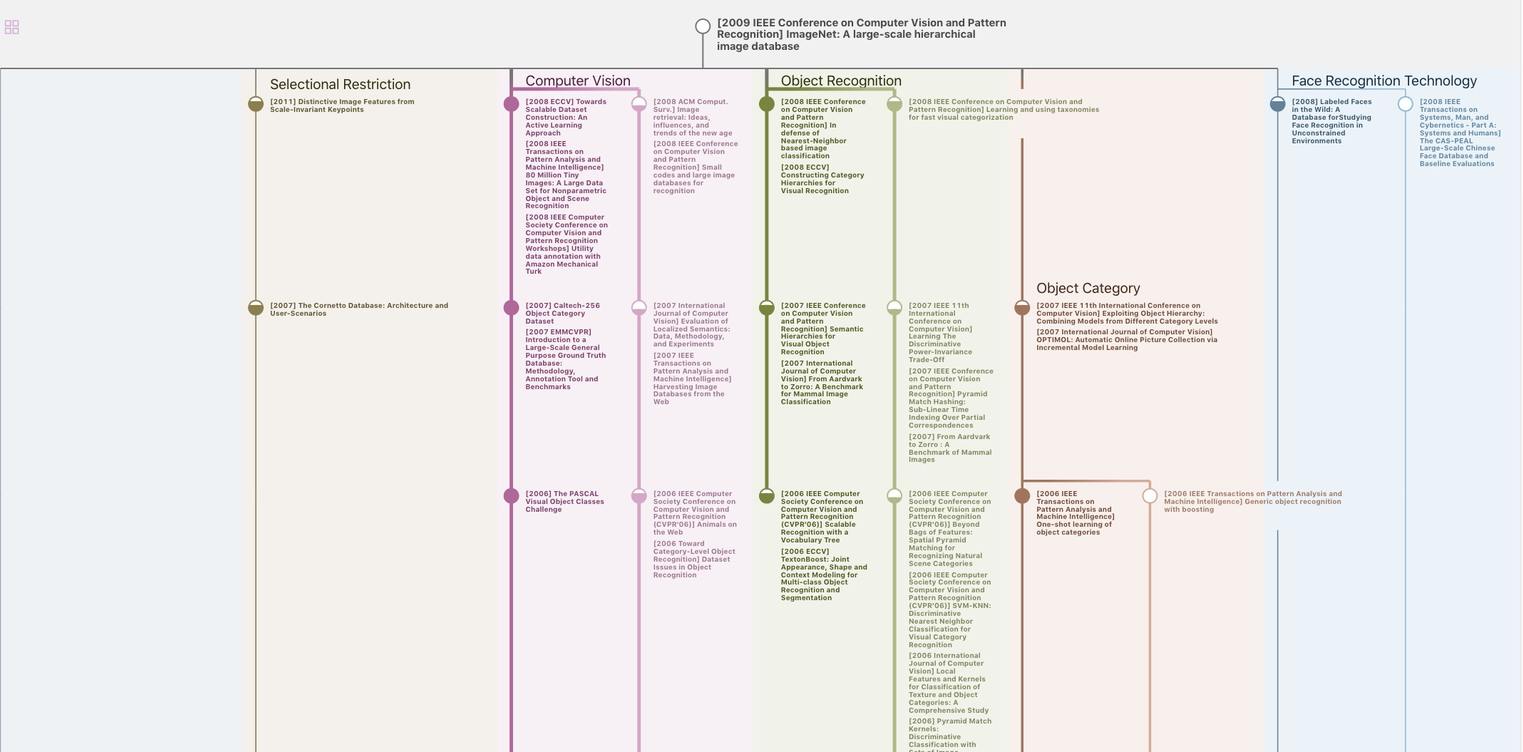
生成溯源树,研究论文发展脉络
Chat Paper
正在生成论文摘要