Atmospheric turbulence degraded image restoration using a modified dilated convolutional network
IET IMAGE PROCESSING(2022)
Abstract
Long-distance imaging is generally blurry and warped due to the presence of atmospheric turbulence, which harms the performance of the photoelectric system. Among these, real-time restoration based on a single turbulence-degraded image has always been a challenging topic that everyone is concerned about. The approach performed here optimizes the convolutional neural network using residual learning and smoothed dilated convolutions, which may increase the field of vision under the situation of limited GPU memory. To identify the model's performance, the authors employ training and test data with strong, medium, and weak levels synthesized by the Fried kernel, the real-time data captured by the Ritchey-Chretien telescope, the Open Turbulent Images Set and the real comparative data. Furthermore, the proposed model is compared to previous state-of-the-art approaches. The experimental results demonstrate that the proposed novel model can recover turbulently degraded images more effectively.
MoreTranslated text
Key words
Single Image Restoration,Depth Estimation,Low-Light Enhancement,Underwater Imaging,Image Processing
AI Read Science
Must-Reading Tree
Example
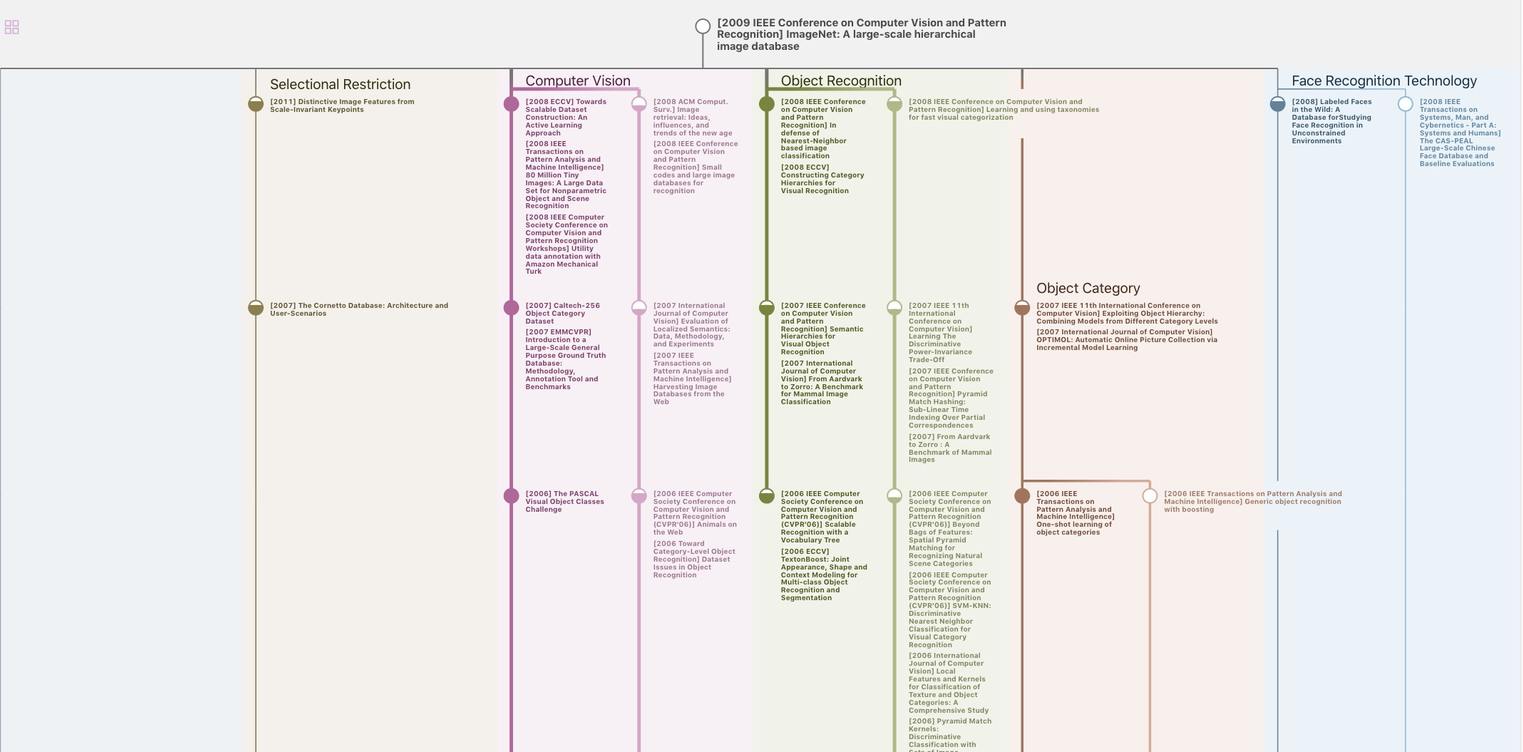
Generate MRT to find the research sequence of this paper
Chat Paper
Summary is being generated by the instructions you defined