A multi-autoencoder fusion network for fast image prediction of aircraft ice accretion
PHYSICS OF FLUIDS(2022)
摘要
Icing scaling is of great importance for ice wind tunnel experiments and airworthiness certification. In this study, MAEFN (Multi-Autoencoder Fusion Network) method is developed to achieve fast icing image prediction. The novelty of MAEFN lies in its multi-module network structure, which enables the control of the training process. Such a structure can solve the problem that icing image generation networks are difficult to train directly and effectively adjust the accuracy of icing images. MAEFN is divided into four modules, i.e., feature extraction, mapping relationship construction, image generation, and image post-processing. Four neural networks are used to complete different steps, and all models are finally fused into the MAEFN model. MAEFN generates a 120 x 120 icing image through a seven-dimensional icing condition vector and achieves an average pixel accuracy of 98.85% on the supercritical wing. The standard deviation on the validation set is 0.0117, and the pixel accuracy of 680 out of 777 validation images exceeds 98%. It takes 2000 s to train 7000 icing images on V100 GPU. Furthermore, the efficiency of the fast icing algorithm is improved significantly by 25 000 times compared with the traditional icing algorithm, which only costs for a single case. Based on MAEFN, the exhaustive icing scaling method is realized, and similar icing results of four special ice shapes are found from 1 x 10(6) icing images in a few minutes. The proposed image generation method MAEFN can be further developed for highly complex icing images such as long-time icing results or other anti-icing tasks such as critical icing analysis. Published under an exclusive license by AIP Publishing.
更多查看译文
关键词
fast image prediction,ice,aircraft,multi-autoencoder
AI 理解论文
溯源树
样例
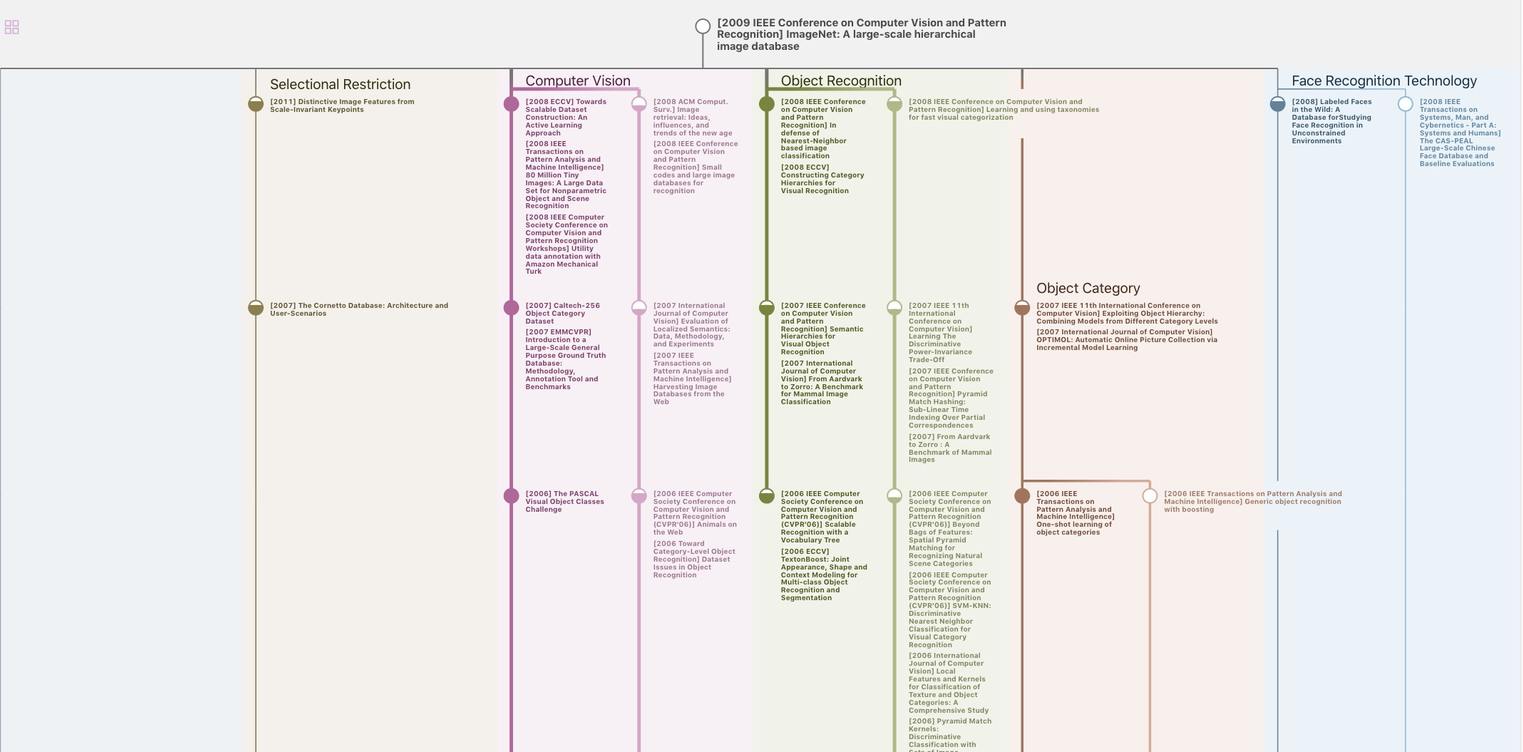
生成溯源树,研究论文发展脉络
Chat Paper
正在生成论文摘要