Extraction of Floating Raft Aquaculture Areas from Sentinel-1 SAR Images by a Dense Residual U-Net Model with Pre-Trained Resnet34 as the Encoder
REMOTE SENSING(2022)
摘要
Marine floating raft aquaculture (FRA) monitoring is significant for marine ecological environment and food security assessment. Synthetic aperture radar-based monitoring is considered to be an effective means of FRA identification because of its capability for all-weather applications. Considering the poor generalization and extraction accuracy of traditional monitoring methods, a semantic segmentation model called D-ResUnet is proposed to extract FRA areas from Sentinel-1 images. The proposed model has a U-Net-like structure but combines the pre-trained ResNet34 as the encoder and adds dense residual units into the decoder. For this model, the final layer and cropping operation of the original U-Net model are removed to eliminate the model parameters. The mean and standard deviation of Precision, Recall, Intersection over Union (IoU), and F1 score are calculated under a five-fold training strategy to evaluate the model accuracy. The test experiments indicated that the proposed model performs well with the F1 of 92.6% and IoU of 86.24% in FRA extraction tasks. In particular, the ablation experiments and application experiments proved the effectiveness of the improvement strategy and the portability of the proposed D-ResUnet model, respectively. Compared with the other three state-of-the-art semantic segmentation models, the experiments demonstrate a clear accuracy advantage of the D-ResUnet model. For the FRA extraction task, this paper presents a promising approach that has refined extraction capability, high accuracy, and acceptable model complexity.
更多查看译文
关键词
floating raft aquaculture, remote sensing, deep learning, residual unit, synthetic aperture radar
AI 理解论文
溯源树
样例
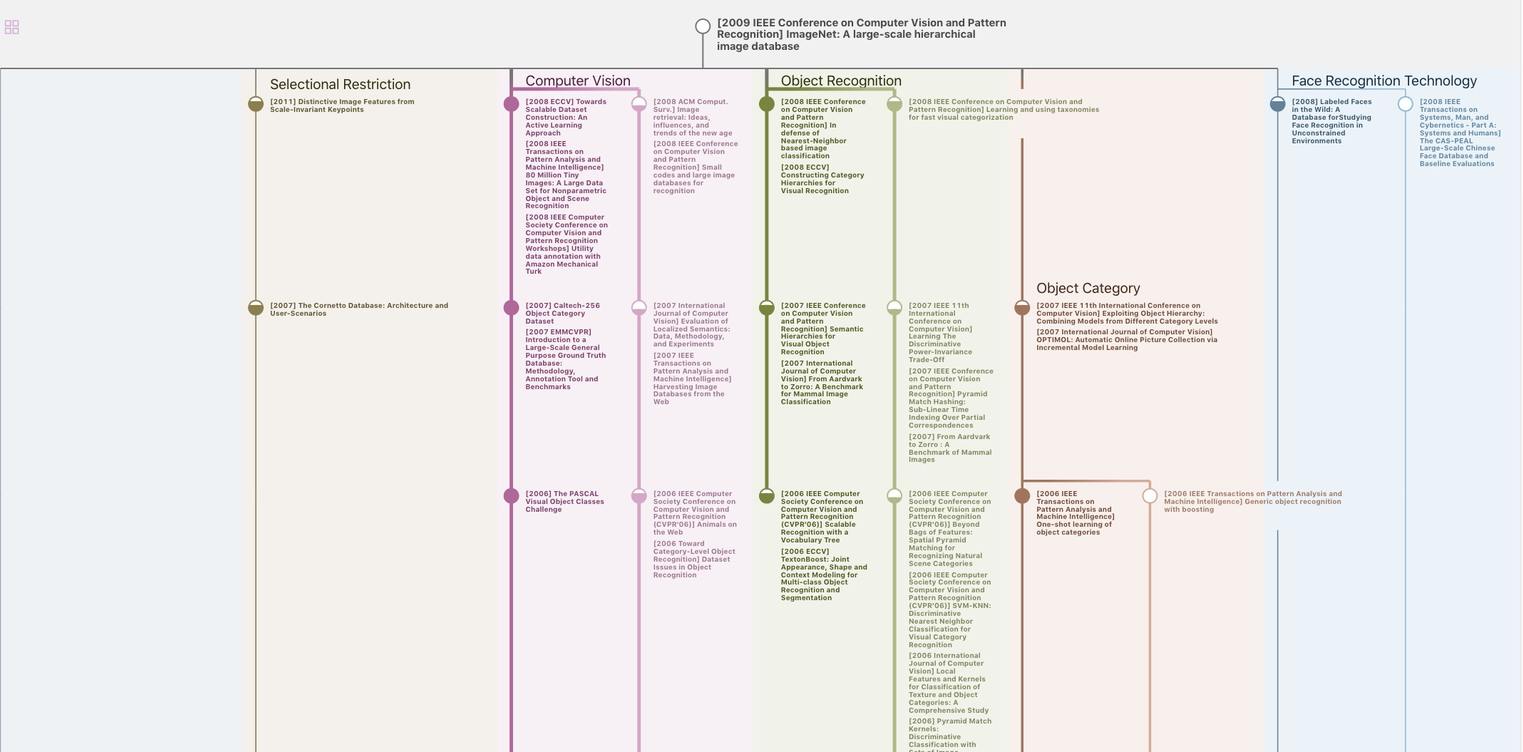
生成溯源树,研究论文发展脉络
Chat Paper
正在生成论文摘要