Artificial intelligence-driven thermal design for additively manufactured reactor cores
Nuclear Engineering and Design(2022)
摘要
•The paper describes application of machine learning (ML), specifically deep learning and Gaussian processes, to optimize the design of complex nuclear engineering systems for which predictive, but computationally intensive, multiphysics solutions are available. With advancements in additive manufacturing (AM), the topological space of component design has dramatically increased, making computational design an essential tool in modern manufacturing. The approach combines reduced-order modeling, simulation, and ML for computational design. High-fidelity reactor thermal hydraulics (TH) simulations are utilized effectively, minimizing the computational and physical costs of large-scale simulations and expensive prototyping, by using reduced-order models to explore the design space. Models are bias corrected by ML error correction using information from the high-fidelity simulations. This method draws on the uncertainty quantification intrinsic to Gaussian processes to provide the best predictions of optimal designs with error bounds. Based on the multi-objective loss function, the method proposes a new set of simulations that maximizes reduction of uncertainty in the regions identified as the most promising to minimize loss.•This work explores, the automatic construction of physics-informed ML methods using emulators validated by very sparse sampling of coupled thermal diffusion and viscous, turbulent flow solutions. The emulators become more accurate as new data are generated by steady-state reduced-order modeling, in which an individual design can be executed on a single graphics processing unit (GPU) in a few minutes. The parallel nature of probing the design space results in almost half a million different reactor designs can be evaluated in an hour on Summit, an Oak Ridge Leadership Computing Facility (OLCF) supercomputer.
更多查看译文
关键词
Artificial intelligence (AI),Machine learning (ML),Computational fluid dynamics (CFD),Thermal hydraulics (TH),Reactor design,Optimization
AI 理解论文
溯源树
样例
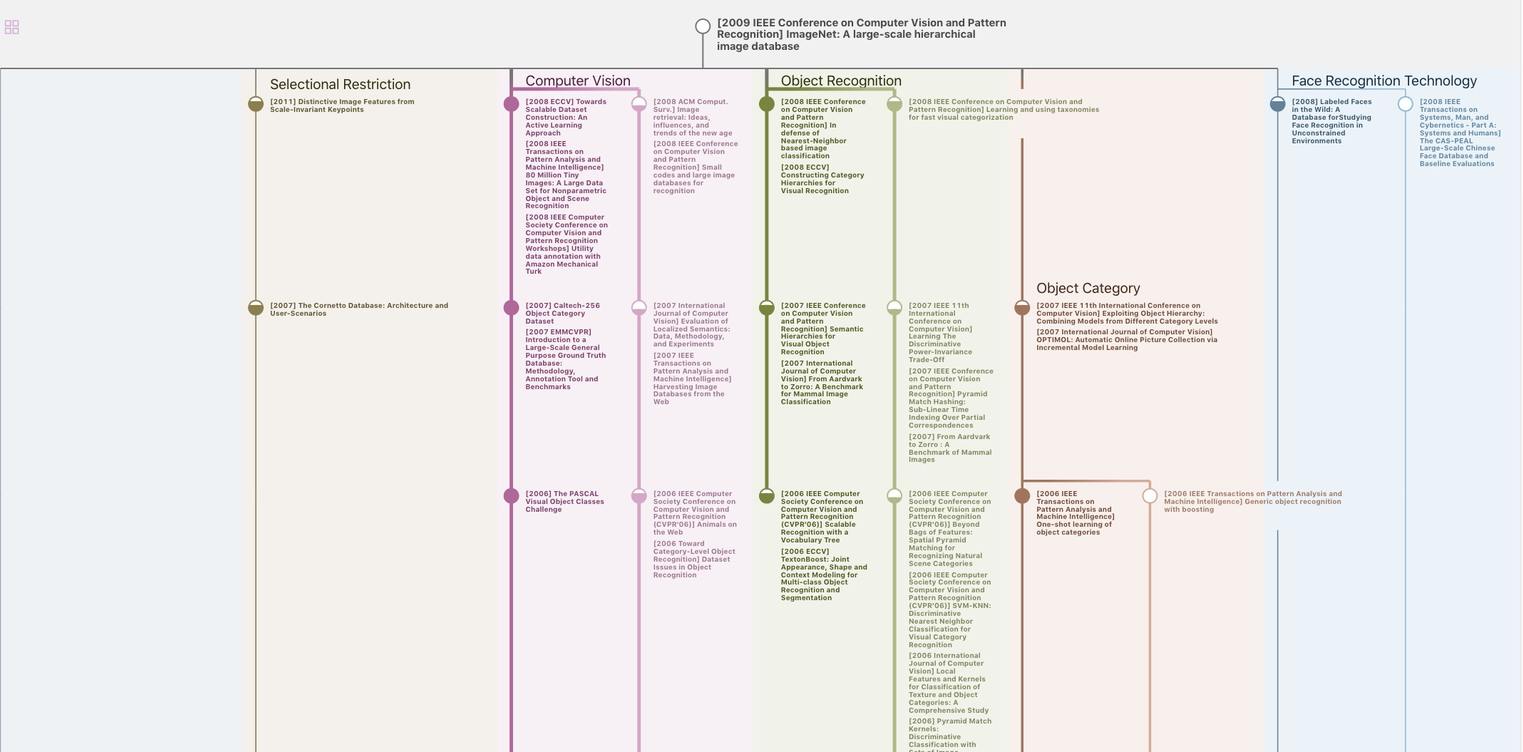
生成溯源树,研究论文发展脉络
Chat Paper
正在生成论文摘要