Automation of cleaning and ensembles for outliers detection in questionnaire data
Expert Systems with Applications(2022)
摘要
This article is focused on the automatic detection of the corrupted or inappropriate responses in questionnaire data using unsupervised outliers detection. The questionnaire surveys are often used in psychology research to collect self-report data and their preprocessing takes a lot of manual effort. Unlike with numerical data where the distance-based outliers prevail, the records in questionnaires have to be assessed from various perspectives that do not relate so much. We identify the most frequent types of errors in questionnaires. For each of them, we suggest different outliers detection methods ranking the records with the usage of normalized scores. Considering the similarity between pairs of outlier scores (some are highly uncorrelated), we propose an ensemble based on the union of outliers detected by different methods. Our outlier detection framework consists of some well-known algorithms but we also propose novel approaches addressing the typical issues of questionnaires. The selected methods are based on distance, entropy, and probability. The experimental section describes the process of assembling the methods and selecting their parameters for the final model detecting significant outliers in the real-world HBSC dataset.
更多查看译文
关键词
Anomaly detection,Outliers,Questionnaire data,Data cleaning,HBSC
AI 理解论文
溯源树
样例
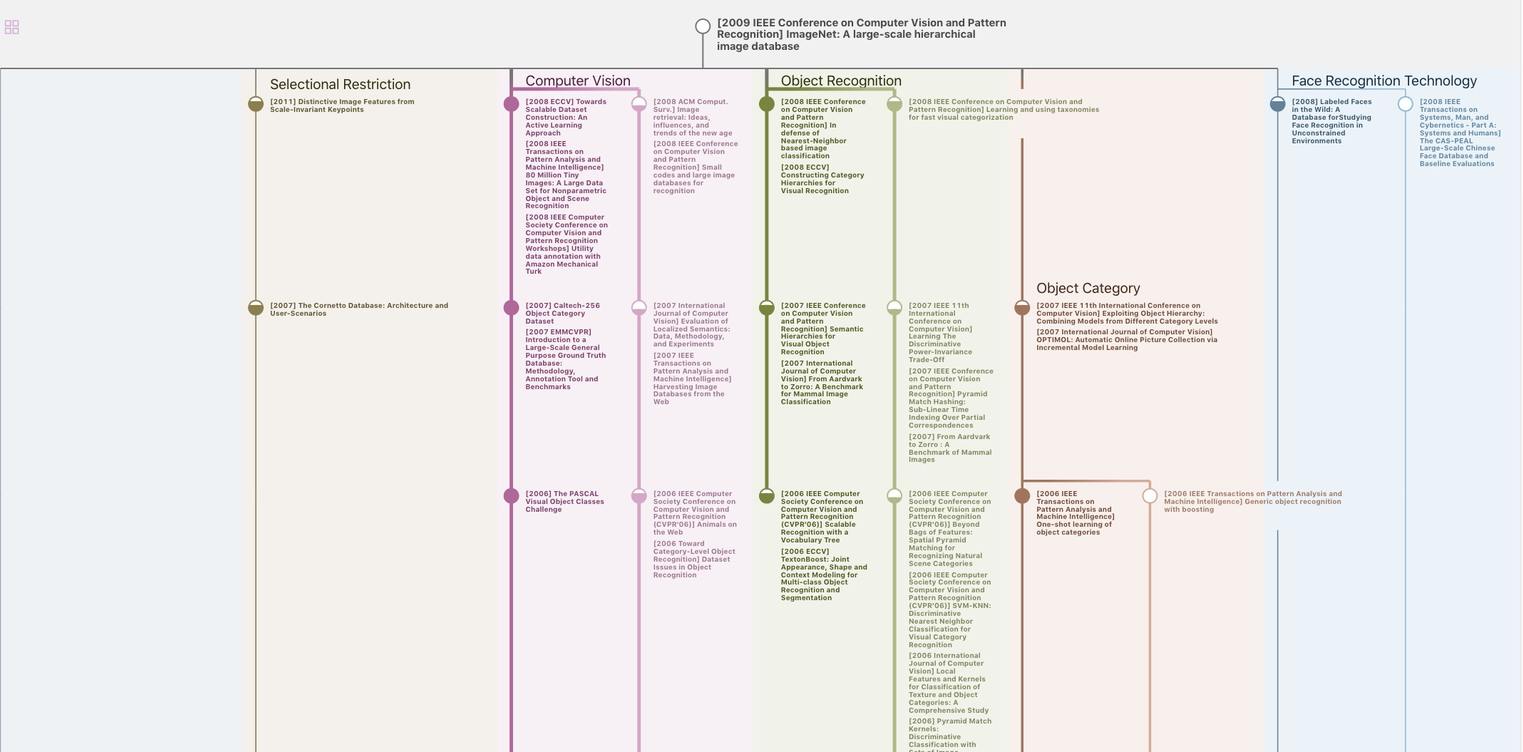
生成溯源树,研究论文发展脉络
Chat Paper
正在生成论文摘要