Short-term Load Forecast Using Improved Long-short Term Memory Network
2022 IEEE 5th International Electrical and Energy Conference (CIEEC)(2022)
摘要
Short-term load forecast is an important task for power system scheduling and operation. In recent years, deep learning methods have been widely applied to load forecast problems because of high accuracy. Among various deep learning models, the Long Short-Term Memory network (LSTM) is one of the most popular models because it can effectively capture the temporal dependencies of load data thus achieving great performance. Different from existing works that combine LSTM with other models, this paper improves the load forecast performance of LSTM by modifying the network structure and fully utilizing multi-time scale load features. First, an Improved LSTM model is proposed by adding a zigzag pathway in the network which helps to improve the transfer of information between different time steps and layers. In the meanwhile, a framework is also proposed for short-term load forecast which utilizes multi-time scale information including weekly features, daily features, and recent-hour features. Such a framework can well capture the load trend at different time scales, thus helping to improve forecast accuracy. Case studies on a public load dataset demonstrate the performance of the proposed method.
更多查看译文
关键词
short-term load forecast,Improved LSTM network,multi-time scale features
AI 理解论文
溯源树
样例
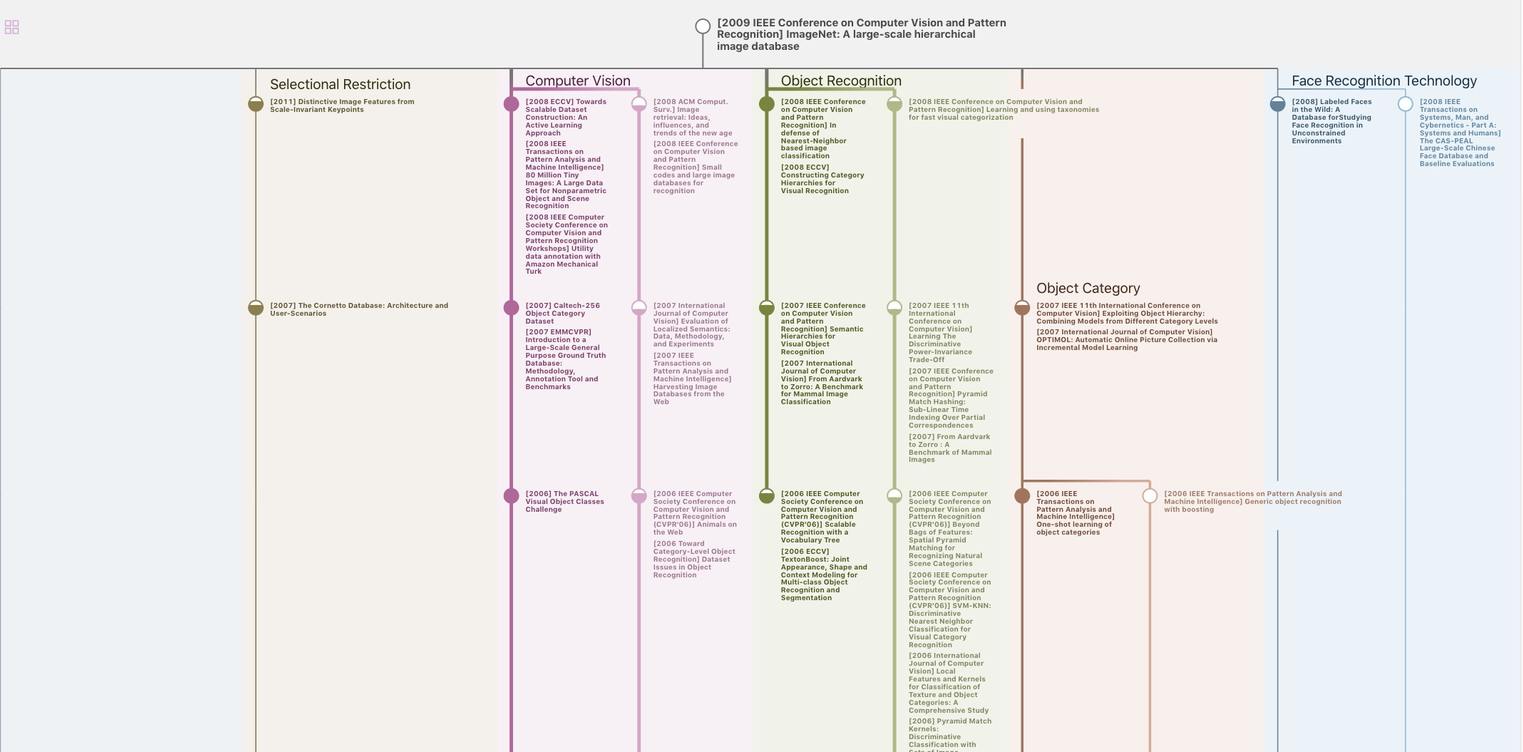
生成溯源树,研究论文发展脉络
Chat Paper
正在生成论文摘要