Personalized risk evaluation for outcome prediction in anca associated vasculitis (aav) using latent class analysis and machine learning.
Annals of the Rheumatic Diseases(2022)
摘要
BackgroundANCA associated vasculitides (AAV) are a heterogeneous group of rare diseases with unknown etiology. In the most severe cases AAV can lead to end stage kidney disease or death. Since etiology and detailed pathogenesis of AAV is not known, the prediction of disease outcome at the time of diagnosis is challenging. Thus, there is an unmet need for tools to identify patients with the highest risk of organ dysfunction and death and apply effective personalized therapy.ObjectivesThe aim of this work was to search for tools allowing outcome prediction at the time of AAV diagnosis. Early identification of patients, who are likely to develop severe organ dysfunction and death is crucial for appropriate disease management. Induction therapy in AAV relays on immunosuppressive drugs characterized by a high risk of severe side effects. Thus, their administration in high doses should be limited only to individual patients with an especially high risk of poor outcome.MethodsWe applied here two methods of identification of AAV patients at risk to develop severe organ dysfunction and death. First method (latent class analysis [LCA] followed by logistic regression) was meant to subcategorize patients and identify a subgroup at subjects at risk to develop chronic renal replacement therapy (CRRT) and death [1]. Second, served to assess individual poor outcome risk and was based on two machine learning (ML) classifiers, which by analyzing clinical information allow assigning computed risk for CRRT and death in an individual patient allowing to identify subjects with high risk of chronic replacement therapy (CRRT) and death. We have evaluated a number of different approaches to build the ML models (including logistic regression, support vector machines, random forests), and obtained the best results for the gradient boosting algorithm implementation called LightGBM [2]. It works as a sequential ensemble of so-called weak learners (decision trees) finally combined in a one prediction model. Both analyses were based on retrospective data from Polish national AAV registry (POLVAS) [3] including presently 565 GPA and 135 MPA patients. The parameters used were: demographic data and laboratory parameters, specific organ involvement, ANCA specificity and time between selected stages of the disease.ResultsLCA used on our AAV cohort identified four subphenotypes – three already previously proposed - and revealing a fourth clinically relevant subphenotype. This new subphenotype includes only GPA patients, usually diagnosed at a younger age as compared to other groups, and characterized by multiorgan involvement, high relapse rate, relatively high risk of death, but no end-stage kidney disease. Logistic regression analysis revealed significant differences in the risk of CRRT and death between those subphenotypes – the worst prognosis was found for severe MPO AAV. On the other hand, using ML approach we obtained an individual prediction model with potentially relevant clinical performance (ROC AUC of 0.85 for CRRT and 0.82 for death).ConclusionWe consider results obtained encouraging. They may offer a new insight into AAV course based on data available at diagnosis, and create a solid foundation for potential clinical decision support system.References[1]Wójcik K et al. Subphenotypes of ANCA-associated vasculitis identified by latent class analysis. Clin Exp Rheumatol. 2021 Mar-Apr;39 Suppl 129(2):62-68.[2]Ke G, at al. Light GBM: A Highly Efficient Gradient Boosting Decision Tree. Advances in Neural Information Processing Systems 30 (NIPS 2017), pp. 3149-3157.[3]Wójcik K et al. Clinical characteristics of Polish patients with ANCA-associated vasculitides-retrospective analysis of POLVAS registry. Clin Rheumatol. 2019 Sep;38(9):2553-2563.AcknowledgementsThis work was supported by a grant from Polish National Science Center UMO-2018/31/B/NZ6/03898Disclosure of InterestsNone declared
更多查看译文
关键词
pos0253 personalized risk evaluation,anca associated vasculitis,outcome prediction,latent class analysis,machine learning
AI 理解论文
溯源树
样例
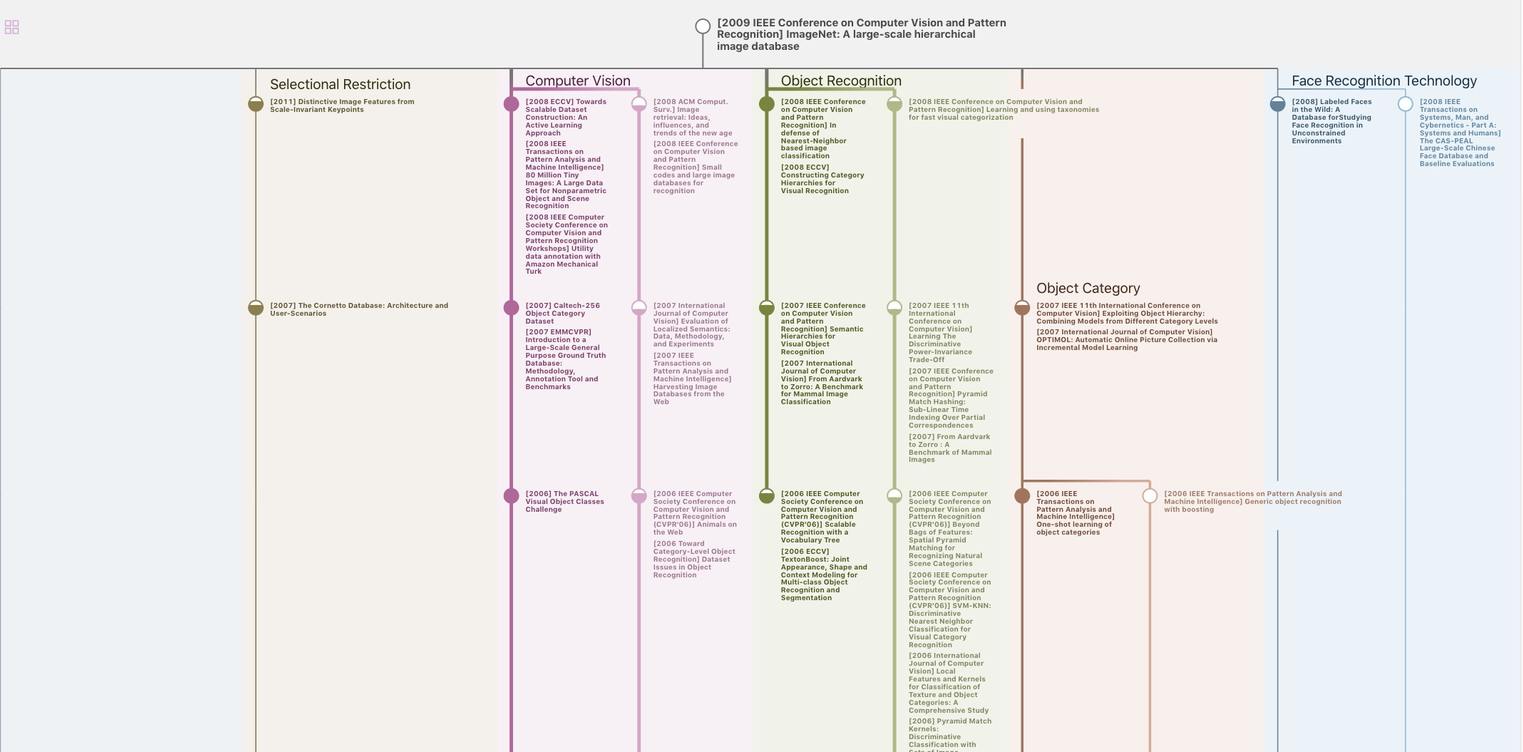
生成溯源树,研究论文发展脉络
Chat Paper
正在生成论文摘要