SRRNet: A Semantic Representation Refinement Network for Image Segmentation
IEEE TRANSACTIONS ON MULTIMEDIA(2023)
摘要
Semantic context has raised concerns in semantic segmentation. In most cases, it is applied to guide feature learning. Instead, this paper applies it to extract the semantic representation, which records the global feature information of each category with a memory tensor. Specifically, we propose a novel semantic representation (SR) module, which consists of semantic embedding (SE) and semantic attention (SA) blocks. The SE block adaptively embeds features into the semantic representation by calculating the memory similarity, and the SA block aggregates the embedded features with semantic attention. The main advantages of the SR module lie in three aspects: i) it enhances the representation ability of semantic context by employing global (cross-image) semantic information; ii) it improves the consistency of intraclass features by aggregating global features of the same categories; and iii) it can be extended to build a semantic representation refinement network (SRRNet) by iteratively applying the SR module across multiple scales, shrinking the semantic gap and enhancing the structural reasoning of the model. Extensive experiments demonstrate that our method significantly improves the segmentation results and achieves superior performance on the PASCAL VOC 2012, Cityscapes, and PASCAL Context datasets.
更多查看译文
关键词
Segmentation,semantic representation,convolutional neural networks,semantic embedding,semantic attention
AI 理解论文
溯源树
样例
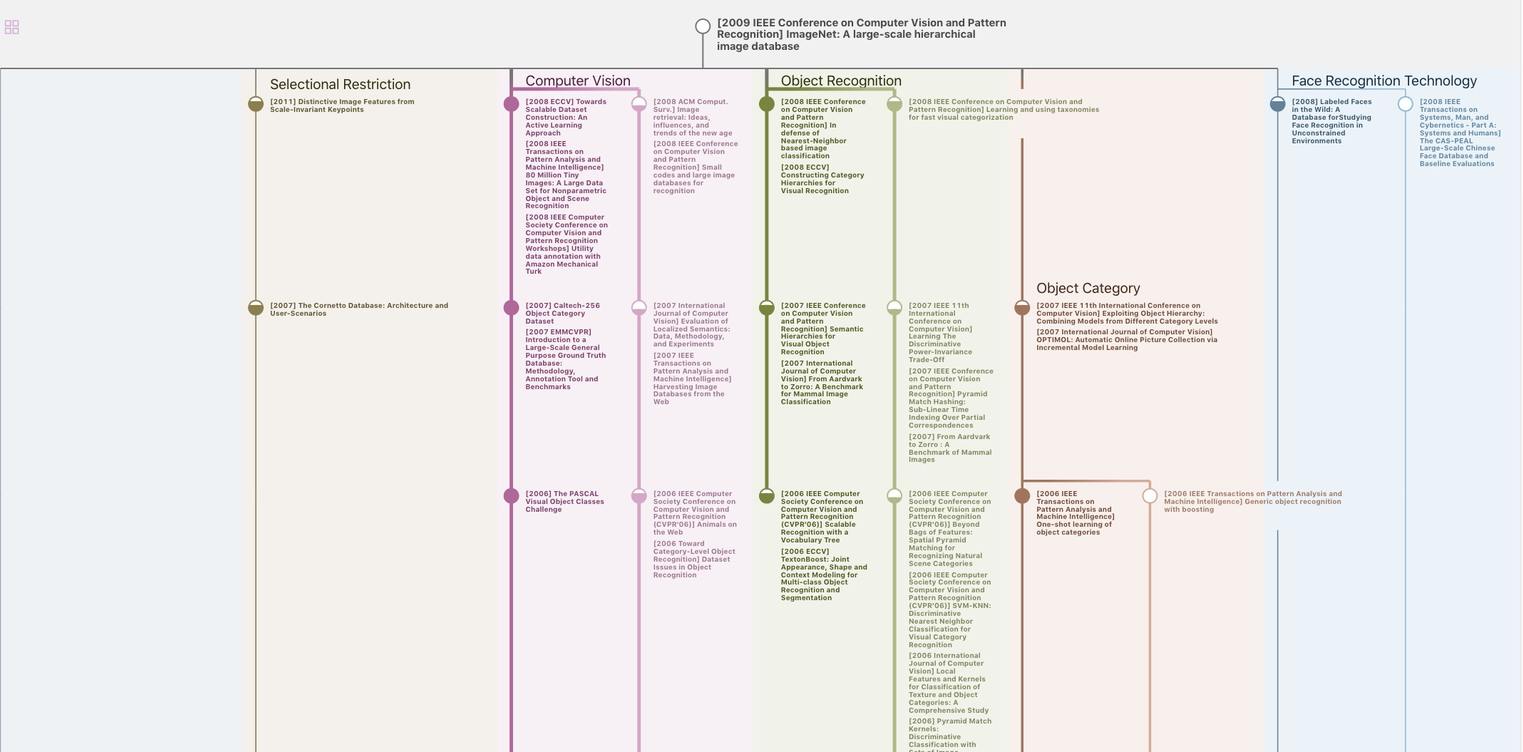
生成溯源树,研究论文发展脉络
Chat Paper
正在生成论文摘要