Projection-Free Non-Smooth Convex Programming
arXiv (Cornell University)(2022)
摘要
In this paper, we provide a sub-gradient based algorithm to solve general constrained convex optimization without taking projections onto the domain set. The well studied Frank-Wolfe type algorithms also avoid projections. However, they are only designed to handle smooth objective functions. The proposed algorithm treats both smooth and non-smooth problems and achieves an $O(1/\sqrt{T})$ convergence rate (which matches existing lower bounds). The algorithm yields similar performance in expectation when the deterministic sub-gradients are replaced by stochastic sub-gradients. Thus, the proposed algorithm is a projection-free alternative to the Projected sub-Gradient Descent (PGD) and Stochastic projected sub-Gradient Descent (SGD) algorithms.
更多查看译文
关键词
projection-free,non-smooth
AI 理解论文
溯源树
样例
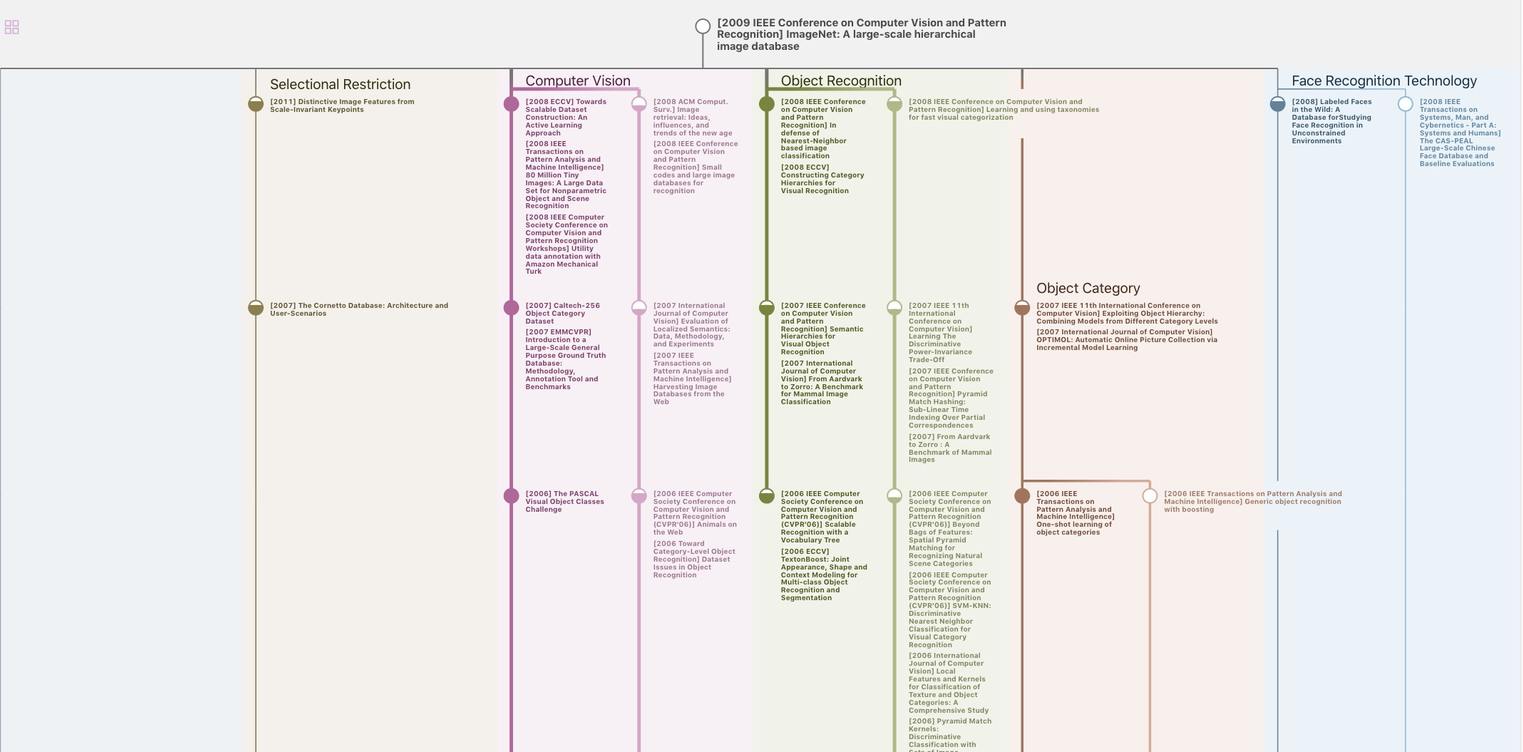
生成溯源树,研究论文发展脉络
Chat Paper
正在生成论文摘要