Generative Data Augmentation Guided by Triplet Loss for Speech Emotion Recognition
Conference of the International Speech Communication Association (INTERSPEECH)(2022)
摘要
Speech Emotion Recognition (SER) is crucial for human-computer interaction but still remains a challenging problem because of two major obstacles: data scarcity and imbalance. Many datasets for SER are substantially imbalanced, where data utterances of one class (most often Neutral) are much more frequent than those of other classes. Furthermore, only a few data resources are available for many existing spoken languages. To address these problems, we exploit a GAN-based augmentation model guided by a triplet network, to improve SER performance given imbalanced and insufficient training data. We conduct experiments and demonstrate: 1) With a highly imbalanced dataset, our augmentation strategy significantly improves the SER performance (+8% recall score compared with the baseline). 2) Moreover, in a cross-lingual benchmark, where we train a model with enough source language utterances but very few target language utterances (around 50 in our experiments), our augmentation strategy brings benefits for the SER performance of all three target languages.
更多查看译文
关键词
speech emotion recognition, speech augmentation, cross lingual
AI 理解论文
溯源树
样例
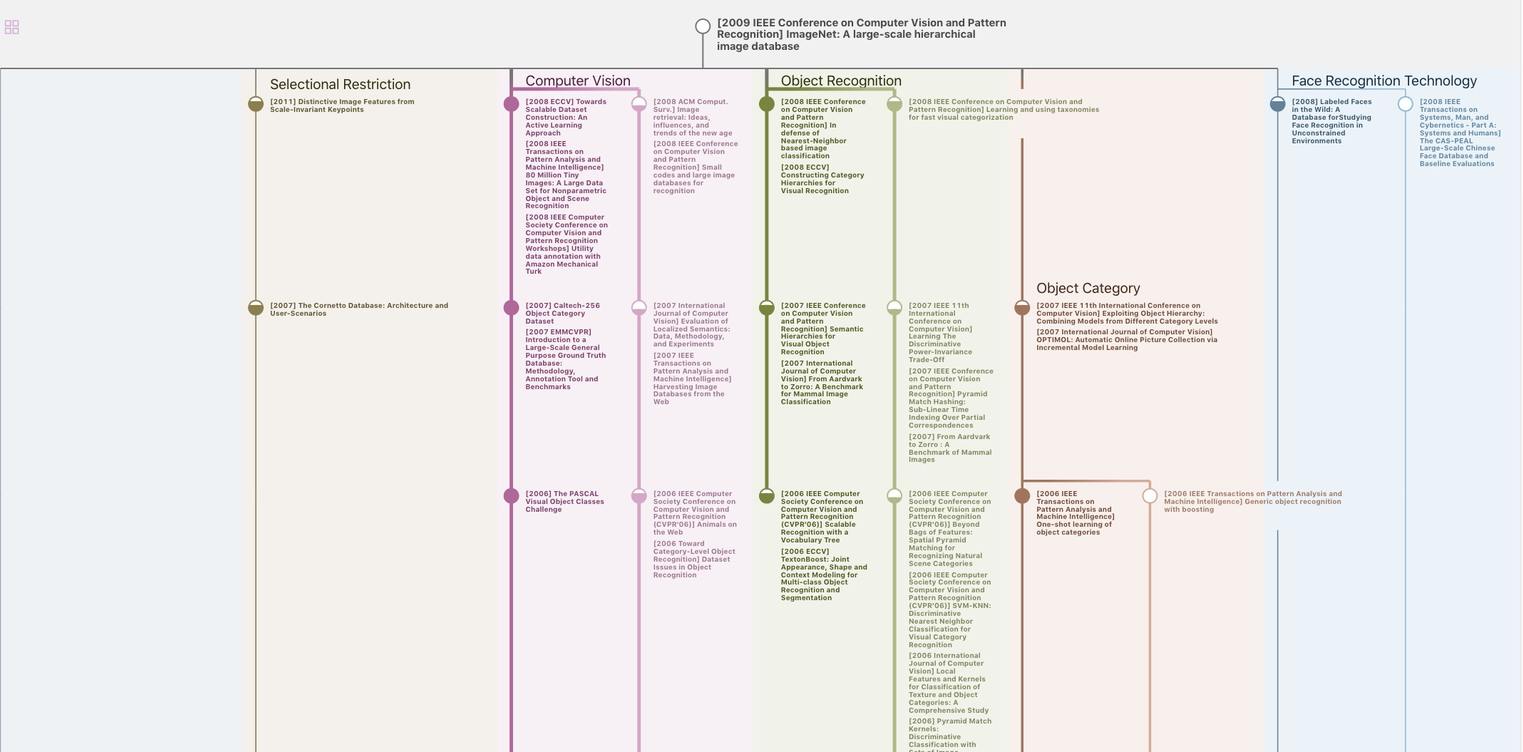
生成溯源树,研究论文发展脉络
Chat Paper
正在生成论文摘要