Improving forecast stability using deep learning
International Journal of Forecasting(2022)
摘要
In this paper, we define forecast (in)stability in terms of the variability in forecasts for a specific time period caused by updating the forecast for this time period when new observations become available, i.e., as time passes. We propose an extension to the state-of-the-art N-BEATS deep learning architecture for the univariate time series point forecasting problem. The extension allows us to optimize forecasts from both a traditional forecast accuracy perspective as well as a forecast stability perspective. We show that the proposed extension results in forecasts that are more stable without leading to a deterioration in forecast accuracy for the M3 and M4 data sets. Moreover, our experimental study shows that it is possible to improve both forecast accuracy and stability compared to the original N-BEATS architecture, indicating that including a forecast instability component in the loss function can be used as regularization mechanism.
更多查看译文
关键词
forecast stability,deep learning
AI 理解论文
溯源树
样例
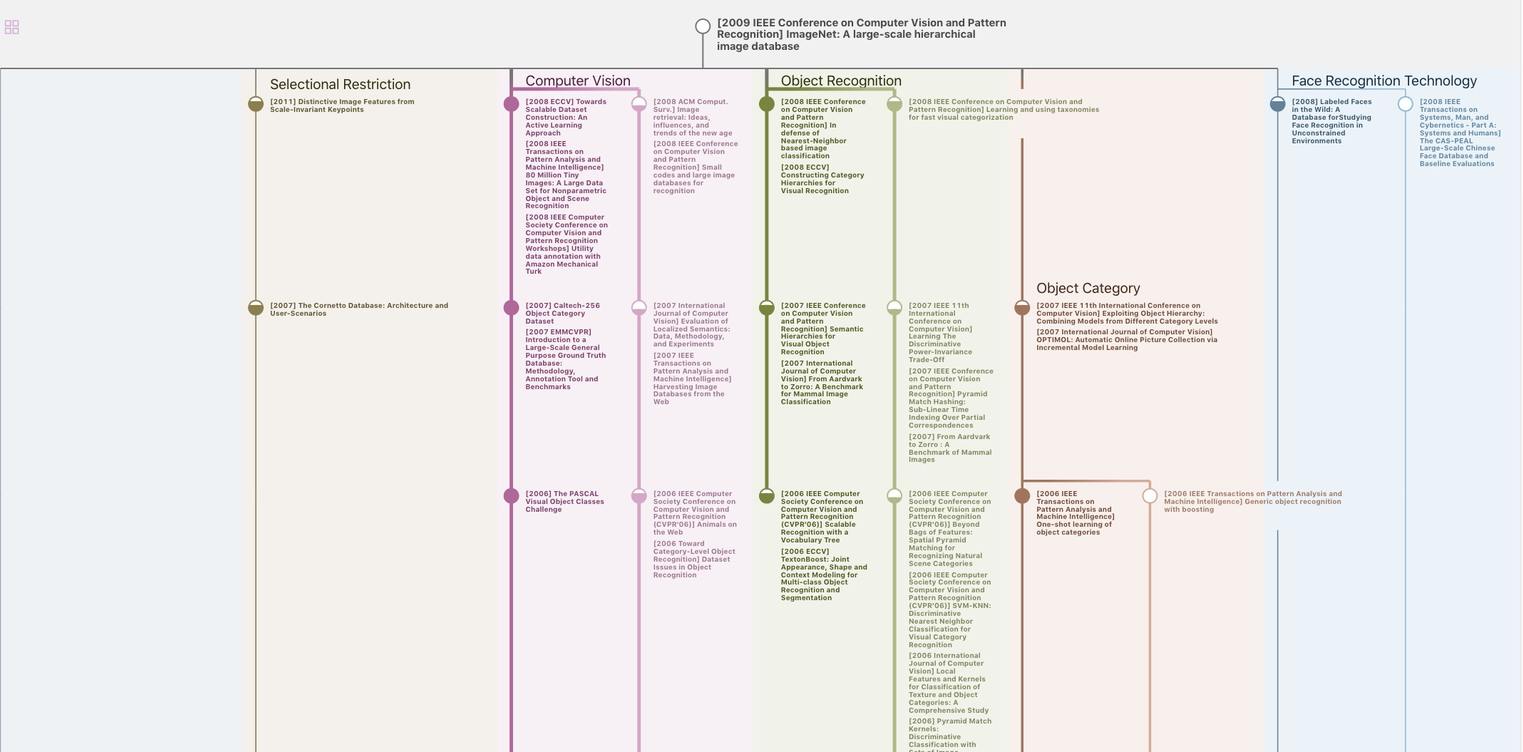
生成溯源树,研究论文发展脉络
Chat Paper
正在生成论文摘要