Representation learning of rare temporal conditions for travel time prediction
arxiv(2022)
摘要
Predicting travel time under rare temporal conditions (e.g., public holidays, school vacation period, etc.) constitutes a challenge due to the limitation of historical data. If at all available, historical data often form a heterogeneous time series due to high probability of other changes over long periods of time (e.g., road works, introduced traffic calming initiatives, etc.). This is especially prominent in cities and suburban areas. We present a vector-space model for encoding rare temporal conditions, that allows coherent representation learning across different temporal conditions. We show increased performance for travel time prediction over different baselines when utilizing the vector-space encoding for representing the temporal setting.
更多查看译文
关键词
travel time
AI 理解论文
溯源树
样例
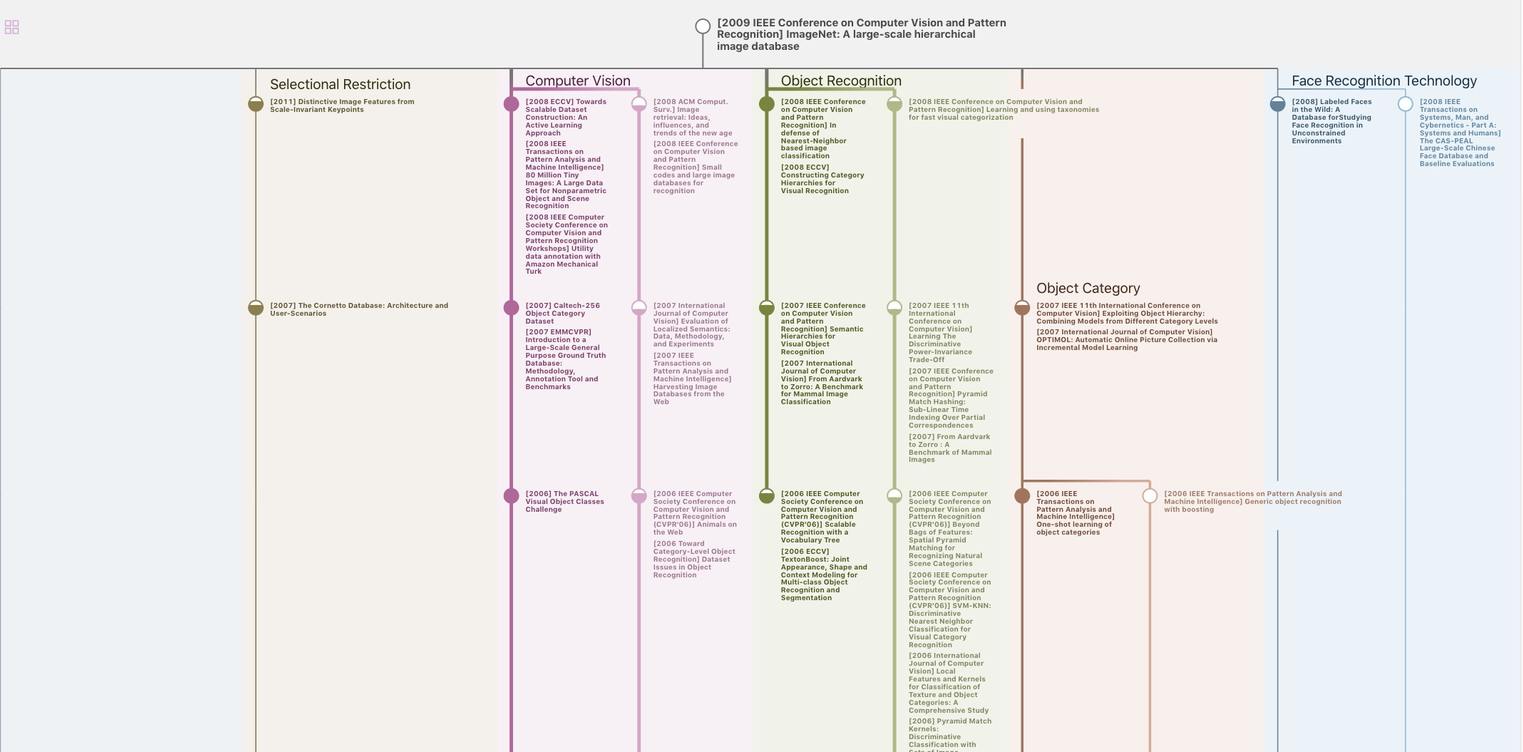
生成溯源树,研究论文发展脉络
Chat Paper
正在生成论文摘要